A Novel Learning Scheme for Inversely-Designing Bonding Wire With Multi-fidelity Data Fusion
2023 International Applied Computational Electromagnetics Society Symposium (ACES-China)(2023)
摘要
This paper introduces a novel approach for efficiently designing bonding wire (BW) using multi-fidelity data assistance. The proposed learning framework consists of two key components: an inversion network and a residual network. The inversion network is utilized to understand the complex relationship between the performance of the BW and its structural parameters within the low-fidelity model space. On the other hand, the residual network is employed to capture the differences between the low-fidelity and high-fidelity data. By combining these networks in a cascaded manner, we can effectively carry out inverse design for the BW. The method achieves an average testing accuracy of 95.62% based on 3000 tests. Notably, compared to traditional forward optimization approaches, our proposed method can fulfill the design requirements in just a fraction of seconds. To validate our approach experimentally, we weld the BW designed using our method onto the microstrip line and perform a de-embedding process. The measured performance aligns well with the design specifications, confirming the effectiveness of our approach.
更多查看译文
关键词
bonding wire,multi-fidelity data,residual learning,input augmentation,neural network
AI 理解论文
溯源树
样例
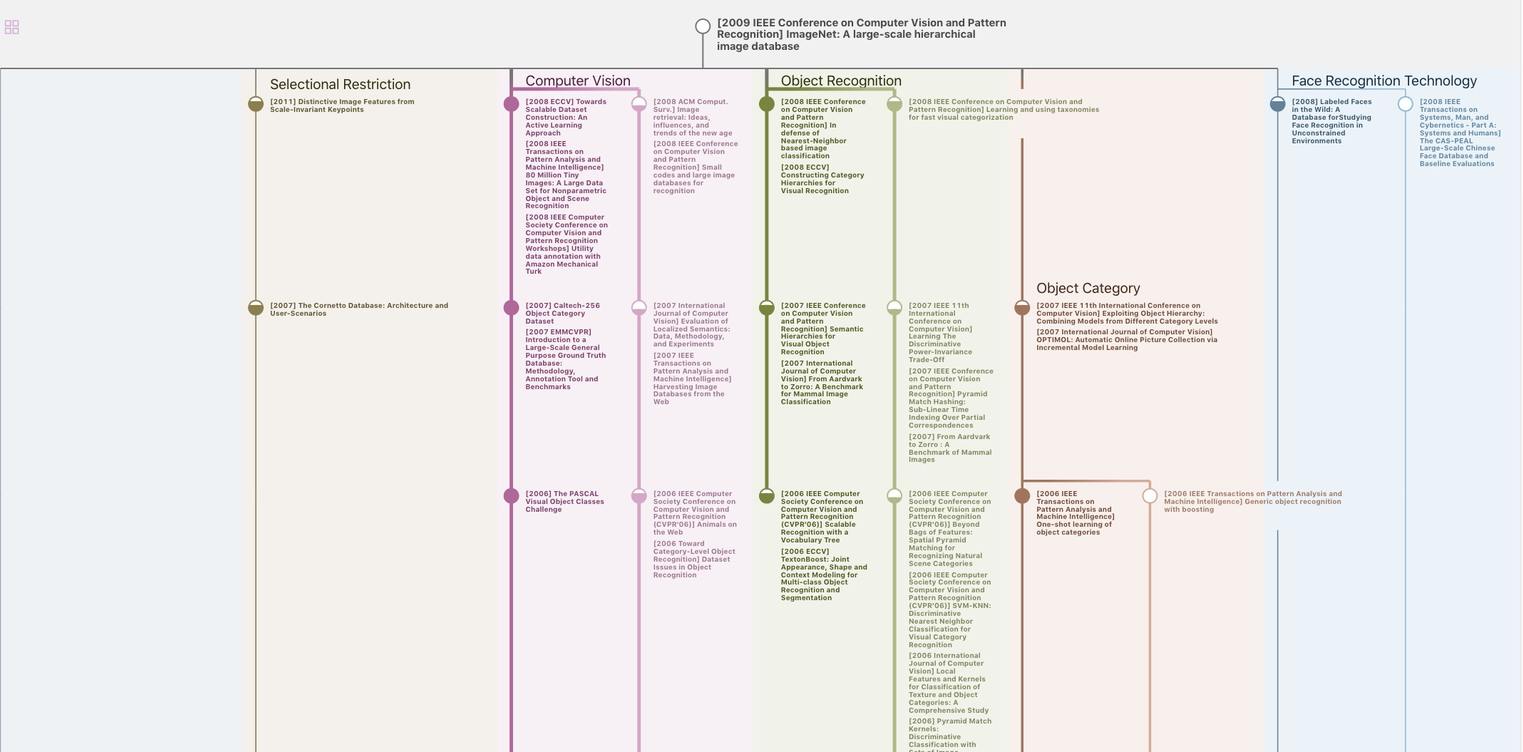
生成溯源树,研究论文发展脉络
Chat Paper
正在生成论文摘要