Scaling Machine Learning at the Edge-Cloud: A Distributed Computing Perspective
2023 19th International Conference on Distributed Computing in Smart Systems and the Internet of Things (DCOSS-IoT)(2023)
摘要
The widespread diffusion of Internet of Things (IoT) devices has led to an exponential growth in the volume of data generated at the edge of the network. With the rapid spread of machine learning (ML)-based applications, performing compute and resource-intensive learning tasks at the edge has become a critical issue, resulting in the need for scalable and efficient solutions that can overcome the resource constraints of edge devices. This paper analyzes the problem of scaling ML applications and algorithms at the edge-cloud continuum from a distributed computing perspective. In particular, we first highlight the limitations of traditional distributed architectures (e.g., clusters, clouds, and HPC systems) when running ML applications that use data generated at the edge. Next, we discuss how to enable traditional ML algorithms combining the benefits of edge computing, such as low-latency processing and privacy preservation of personal user data, with those of cloud computing, such as virtually unlimited computational and storage capabilities. Our analysis provides insights into how properly separated parts of a ML application can be deployed across edge-cloud architectures in order to optimize its execution. More-over, examples of ML applications and algorithms appropriately adapted for the edge-cloud continuum are shown.
更多查看译文
关键词
Machine learning,distributed machine learning,Internet of Things,edge computing,cloud computing,edge-cloud continuum
AI 理解论文
溯源树
样例
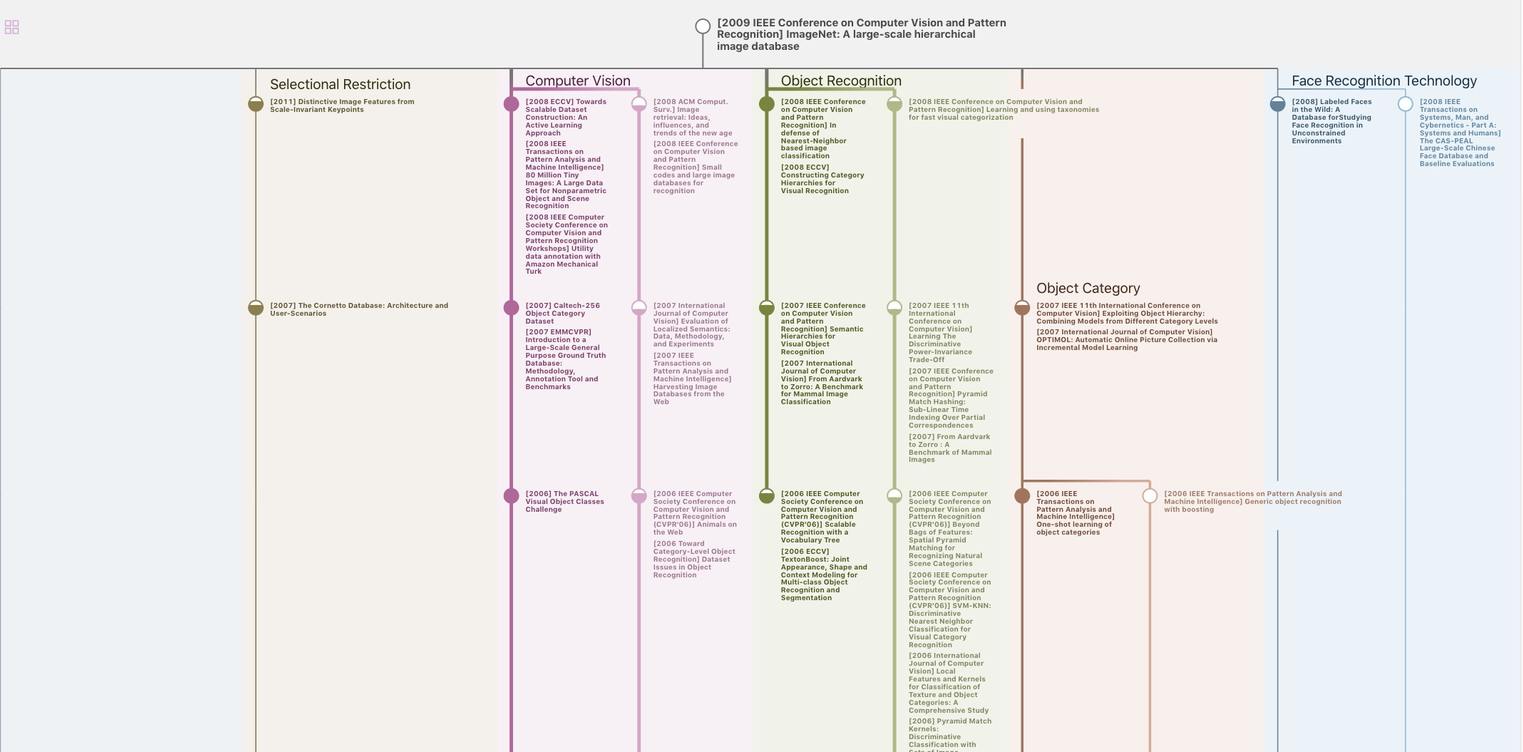
生成溯源树,研究论文发展脉络
Chat Paper
正在生成论文摘要