Safe Deep Reinforcement Learning for Power System Operation under Scheduled Unavailability
2023 IEEE Power & Energy Society General Meeting (PESGM)(2023)
摘要
The electrical grid is a safety-critical system, since incorrect actions taken by a power system operator can result in grid failure and cause harm. For this reason, it is desirable to have an automated power system operator that can reliably take actions that avoid grid failure while fulfilling some objective. Given the existing and growing complexity of power system operation, the choice has often fallen on deep reinforcement learning (DRL) agents for automation, but these are neither explainable nor provably safe. Therefore in this work, the effect of shielding on DRL agent survivability, validation computational time, and convergence are explored. To do this, shielded and unshielded DRL agents are evaluated on a standard IEEE 14-bus network. Agents are tasked with balancing generation and demand through redispatch and topology changing actions at a human timescale of 5 minutes. To test survivability under controlled conditions, varying degrees of scheduled unavailability events are introduced which could cause grid failure if unaddressed. Results show improved convergence and generally greater survivability of shielded agents compared with unshielded agents. However, the safety assurances provided by the shield increase computational time. This will require trade-offs or optimizations to make real-time deployment more feasible.
更多查看译文
关键词
Deep reinforcement learning, power system operation, deep learning, safe deep reinforcement learning
AI 理解论文
溯源树
样例
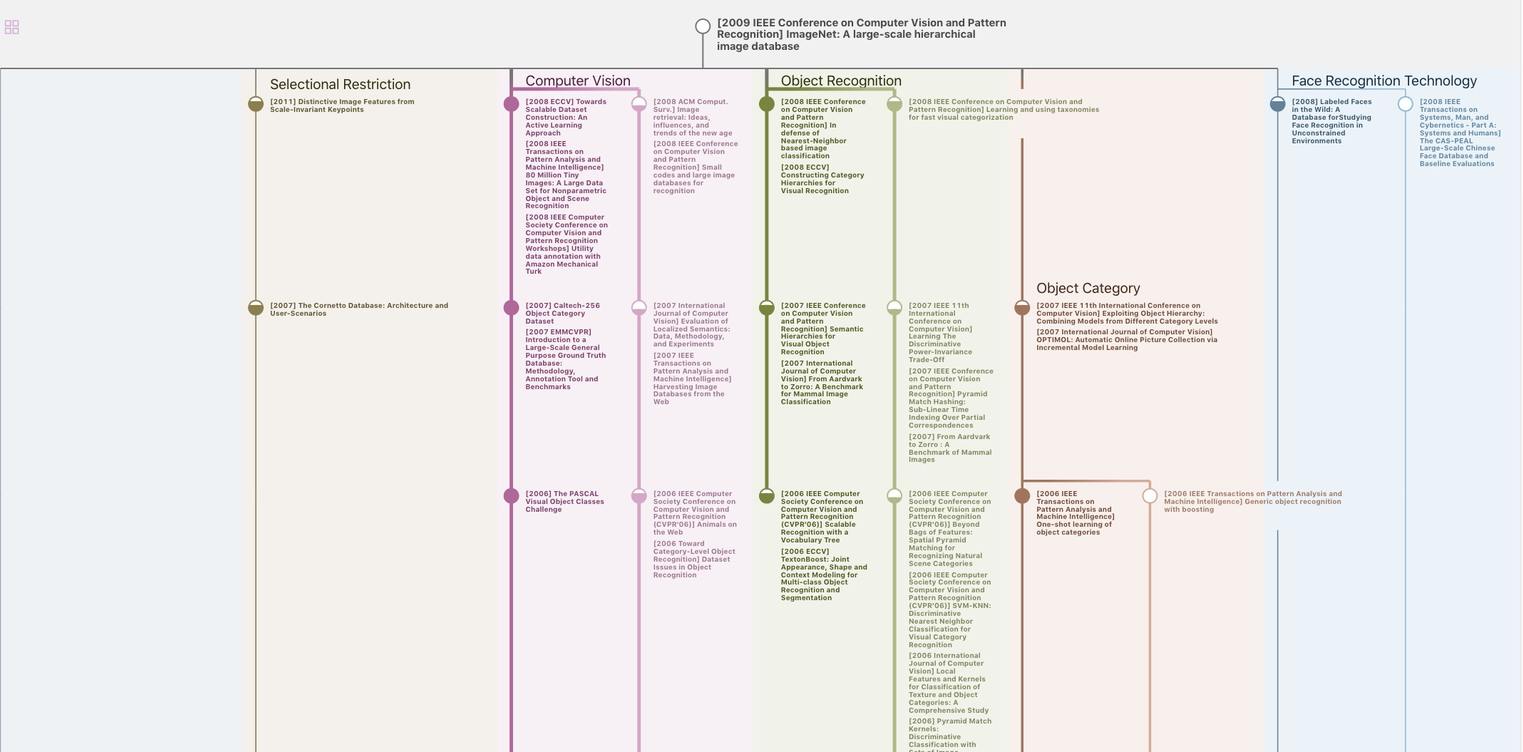
生成溯源树,研究论文发展脉络
Chat Paper
正在生成论文摘要