DMotion: Robotic Visuomotor Control with Unsupervised Forward Model Learned from Videos
2021 IEEE/RSJ International Conference on Intelligent Robots and Systems (IROS)(2021)
摘要
Learning an accurate model of the environment is essential for model-based control tasks. Existing methods in robotic visuomotor control usually learn from data with heavily labelled actions, object entities or locations, which can be demanding in many cases. To cope with this limitation, we propose a method, dubbed DMotion, that trains a forward model from video data only, via disentangling the motion of controllable agent to model the transition dynamics. An object extractor and an interaction learner are trained in an end-to-end manner without supervision. The agent’s motions are explicitly represented using spatial transformation matrices containing physical meanings. In the experiments, DMotion achieves superior performance on learning an accurate forward model in a Grid World environment, as well as a more realistic robot control environment in simulation. With the accurate learned forward models, we further demonstrate their usage in model predictive control as an effective approach for robotic manipulations. Code, video and more materials are available at: https://hyperplane-lab.github.io/dmotion.
更多查看译文
关键词
robotic visuomotor control,heavily labelled actions,video data,controllable agent,object extractor,Grid World environment,realistic robot control environment,model predictive control,robotic manipulations,model-based control task,DMotion,unsupervised forward model learning,transition dynamics,interaction learner,agent motion representation,spatial transformation matrices
AI 理解论文
溯源树
样例
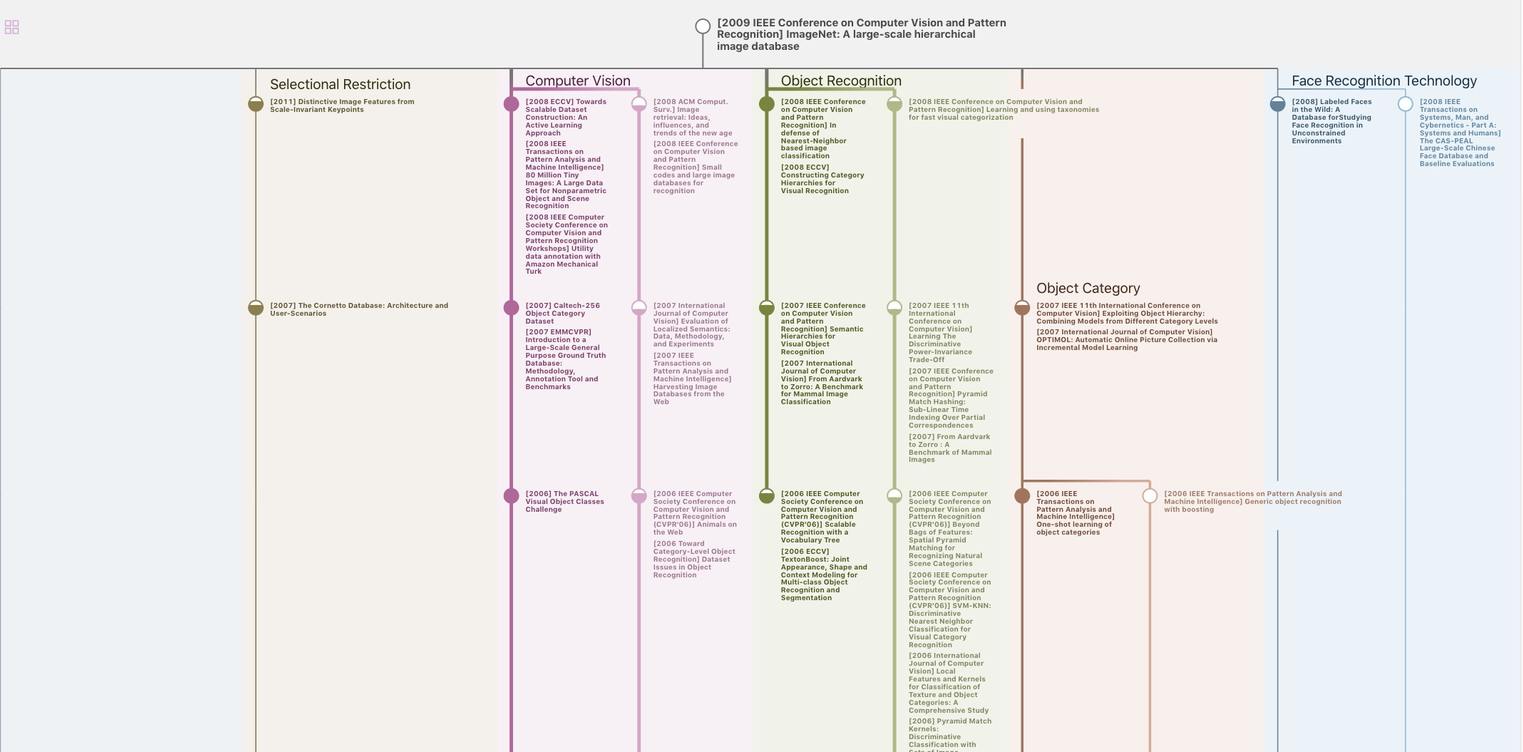
生成溯源树,研究论文发展脉络
Chat Paper
正在生成论文摘要