Principles for Optimal Window Size Selection for Infant and Adult EEG Connectivity Analysis
2023 8th International Conference on Signal and Image Processing (ICSIP)(2023)
摘要
Neural connectivity analysis is often performed on continuous data that has been discretized into temporal windows of a fixed length. However, the selection of an optimal window length is non-trivial, and depends on the properties of the connectivity metric being used as well as the effects of interest within the data (e.g. developmental or inter-brain effects). A systematic investigation of these factors, and objective criteria for window size selection are currently missing in the literature, particularly in regard to pediatric datasets. Here, we provide a principled examination of the effect of window size on optimization of signal to noise ratio for linear and non-linear EEG connectivity, as applied to infant, adult and dyadic (infant-adult) datasets. We employed a linear weighted phase lag index (wPLI), and a nonlinear weighted symbolic mutual information (wSMI) metric to assess brain connectivity for each dataset. Our results showed a clear polar dissociation between linear and non-linear metrics, as well as between infant and adult datasets in optimal window size. Further, optimal dyadic (infant-adult) window size settings defaulted to one or the partner rather than reflecting an intermediate compromise. Given the specificity of these results (i.e. there was no single window size that was optimal for all contrasts), we conclude that a formal analysis of optimal window size may be useful prior to conducting any new connectivity analysis. Here, we recommend guiding principles, performance metrics and decision criteria for optimal and unbiased window size selection.
更多查看译文
关键词
electroencephalography (EEG),connectivity,wPLI,wSMI,adult,infant,hyperscanning
AI 理解论文
溯源树
样例
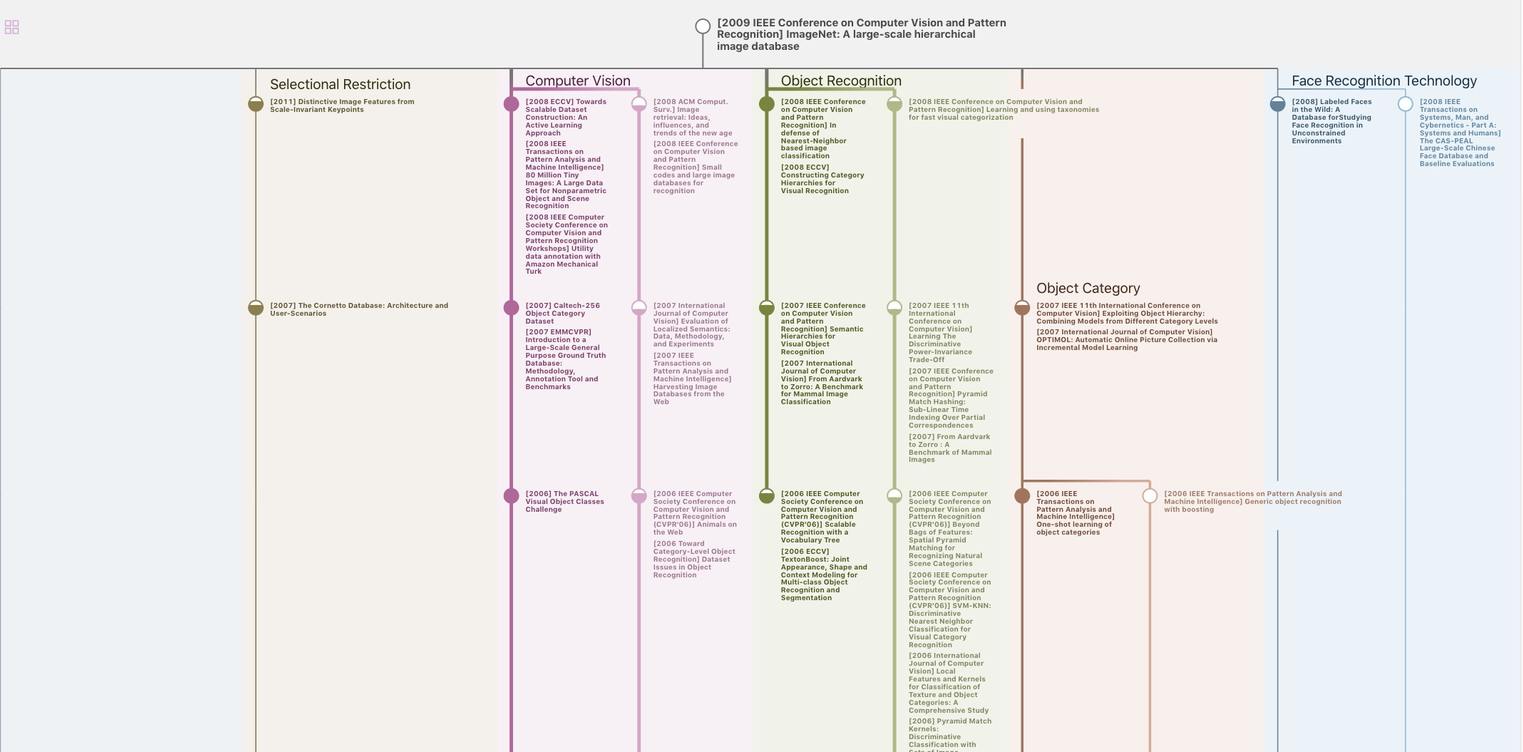
生成溯源树,研究论文发展脉络
Chat Paper
正在生成论文摘要