Research on Machine Learning-based Metagrating Design
2023 IEEE International Workshop on Electromagnetics: Applications and Student Innovation Competition (iWEM)(2023)
摘要
In addition to phase-gradient metasurfaces, metagratings are emerging as another novel approach for radar cross-section (RCS) enhancement. However, traditional electromagnetics-based design methods challenge the expertise and knowledge base of researchers. In this paper, a machine learning-based design scheme for metagrating surface patterns is designed. Based on the Floquet-Bolch (FB) model and the grating equation, the retro-reflection pattern of the incident electromagnetic wave can be controlled by a rational design of the period length of the metagrating. We establish a surrogate model between the geometric parameters of a "butterfly" metagrating structure and its S-parameters. With the assistance of a pretrained neural network and the PSO optimization algorithm, we design a metagrating that achieves a backscattering angle of 32° at 7GHz. Through simulation, we validate that the retroreflection efficiency of the generated metagrating structure exceeds 80%, demonstrating the feasibility of using machine learning to aid in metagrating design.
更多查看译文
关键词
Metagratings,Machine Learning,Artificial Neural Network,Electromagnetic Wave Manipulating
AI 理解论文
溯源树
样例
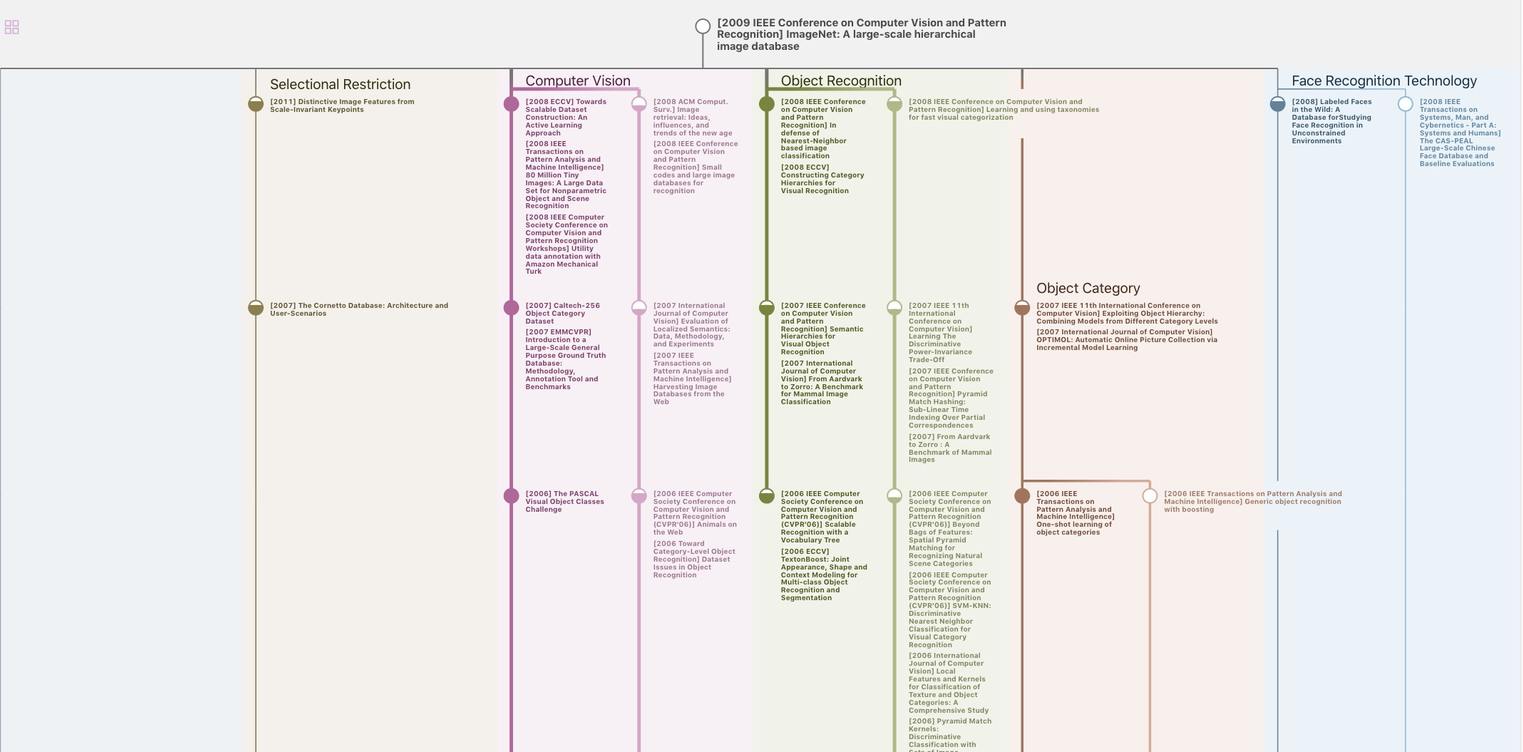
生成溯源树,研究论文发展脉络
Chat Paper
正在生成论文摘要