Multi-Label Oversampling Based on Borderline
2023 International Conference on Machine Learning and Cybernetics (ICMLC)(2023)
摘要
Learning from imbalanced multi-label data is a challenging task. Resampling is one of the most commonly used techniques for handling the imbalance issue by preprocessing the multi-label dataset. Previous studies in traditional single-label learning show that the minority samples located at the borderline are easy to be misclassified, thus cloning them can help improving the performance of the classifier. However, the importance of these samples are overlooked in existing multi-label resampling methods. This paper proposes a multi-label oversampling method based on borderline. We provide a delineation of the minority borderline samples based on their nearest neighbors, and propose a dangerous rate to reflect the risk of a borderline sample being misclassified, which is used to determine the frequency of cloning. Experimental results demonstrate that the proposed method is competitive, which significantly enhances the classification performance.
更多查看译文
关键词
Multi-label classification,Class imbalance,Heuristic,Over-sampling,Borderline
AI 理解论文
溯源树
样例
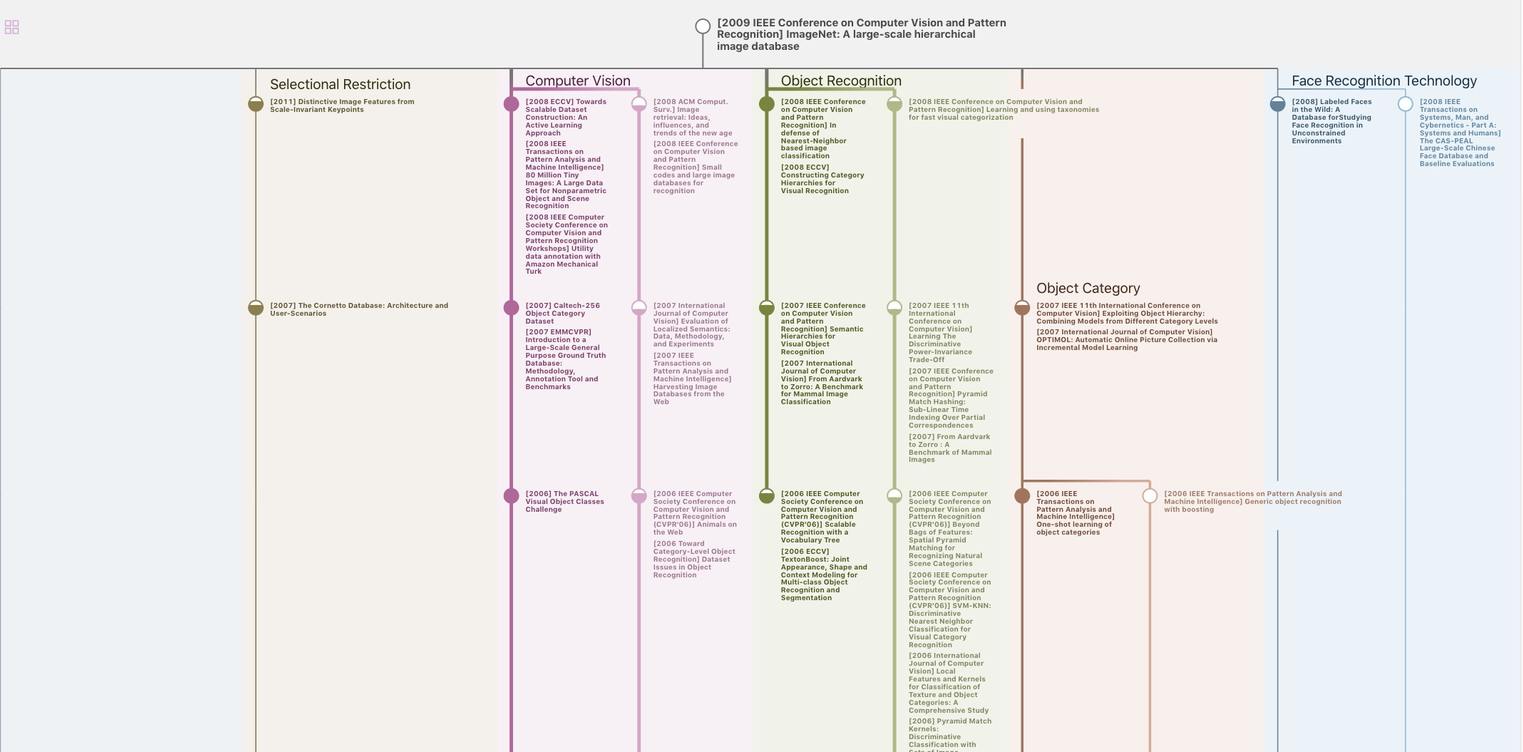
生成溯源树,研究论文发展脉络
Chat Paper
正在生成论文摘要