Improving the Adversarial Robustness of Deep Neural Networks via Efficient Two-Stage Training
2023 International Conference on Machine Learning and Cybernetics (ICMLC)(2023)
摘要
Despite the widespread applications of deep neural networks (DNNs), the potential threats of adversarial examples remain considerable concerns. In this paper, we propose a new proactive defense method based on a two-stage training procedure in order to enhance the adversarial robustness of DNNs. The first stage intends to map the input samples into a feature embedding space with high separability, while the second stage fixes the feature generator and learns the parameters only for the last layer for classification. Different from most of the existing state-of-the-art methods, our method neither spends extensive costs to generate adversarial information, nor constructs a complex penalty function to force the model satisfying specific restrictions under subjective assumptions. Instead, our method cuts off the interactions between the parameter updating for the deep feature generator and the classifier, discovers the relationship between adversarial robustness and separability in embedding space, results in a better interpretability. Substantial experiments on various network structures deployed on benchmark data sets including MNIST, FASHION MNIST and CIFAR10 and their adversary from different attacks demonstrate the rationality of our method.
更多查看译文
关键词
Adversarial robustness,Adversarial defense,Deep neural network,Two-stage training
AI 理解论文
溯源树
样例
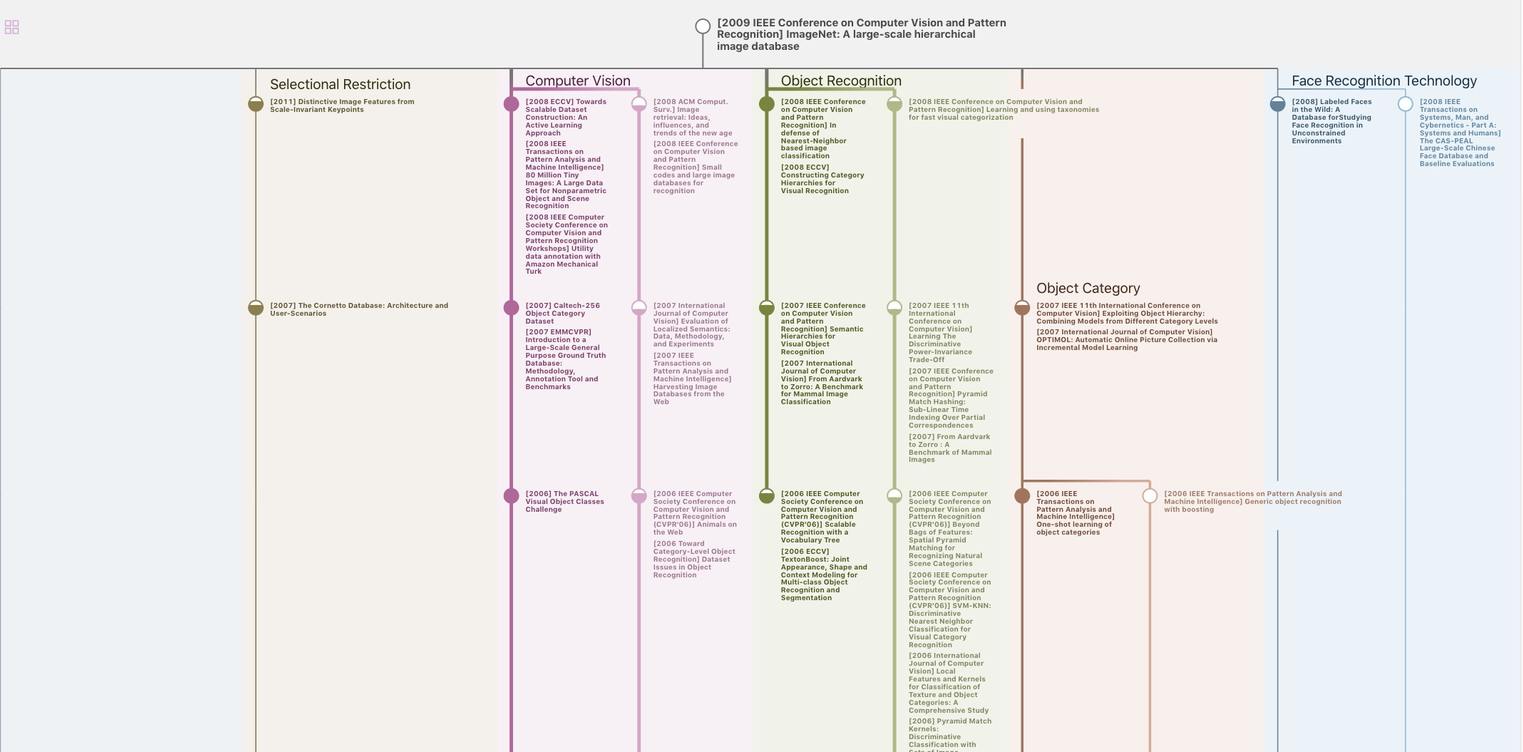
生成溯源树,研究论文发展脉络
Chat Paper
正在生成论文摘要