Adaptive Model Aggregation for Decentralized Federated Learning in Vehicular Networks
2023 19TH INTERNATIONAL CONFERENCE ON NETWORK AND SERVICE MANAGEMENT, CNSM(2023)
摘要
Decentralized federated learning (DFL) enables collaborative training of machine learning models without sharing sensitive data. As such, its application in vehicular networks has gained significant attention. At the core of DFL is the so-called model aggregation, in which each vehicle combines its locally trained model with those received from its neighboring vehicles to generate an updated model that, over time, converges to a global model shared by all vehicles. However, due to high mobility and wireless communication, vehicle-to-vehicle communication is lossy. As a result, a vehicle may receive its neighboring vehicle models only partially. A technical challenge in DFL is how to efficiently utilize such partial models to speed up model training without increasing the training overhead. In this paper, we present an Adaptive Model Aggregation (AMA) algorithm to address this challenge. Our algorithm runs asynchronously on each vehicle and uses a threshold to decide whether to use a partially received model for aggregation. We show that due to the mobility of vehicles, a static threshold is not sufficient and subsequently develop an algorithm based on the contextual multi-arm bandit theory to adaptively compute an optimal threshold for each vehicle based on the dynamics of the network. We evaluate the performance of AMA in realistic environments that include different mobility patterns. Our results show that AMA can decrease DFL aggregation overhead by 83% without reducing the training accuracy compared to non-adaptive aggregation.
更多查看译文
AI 理解论文
溯源树
样例
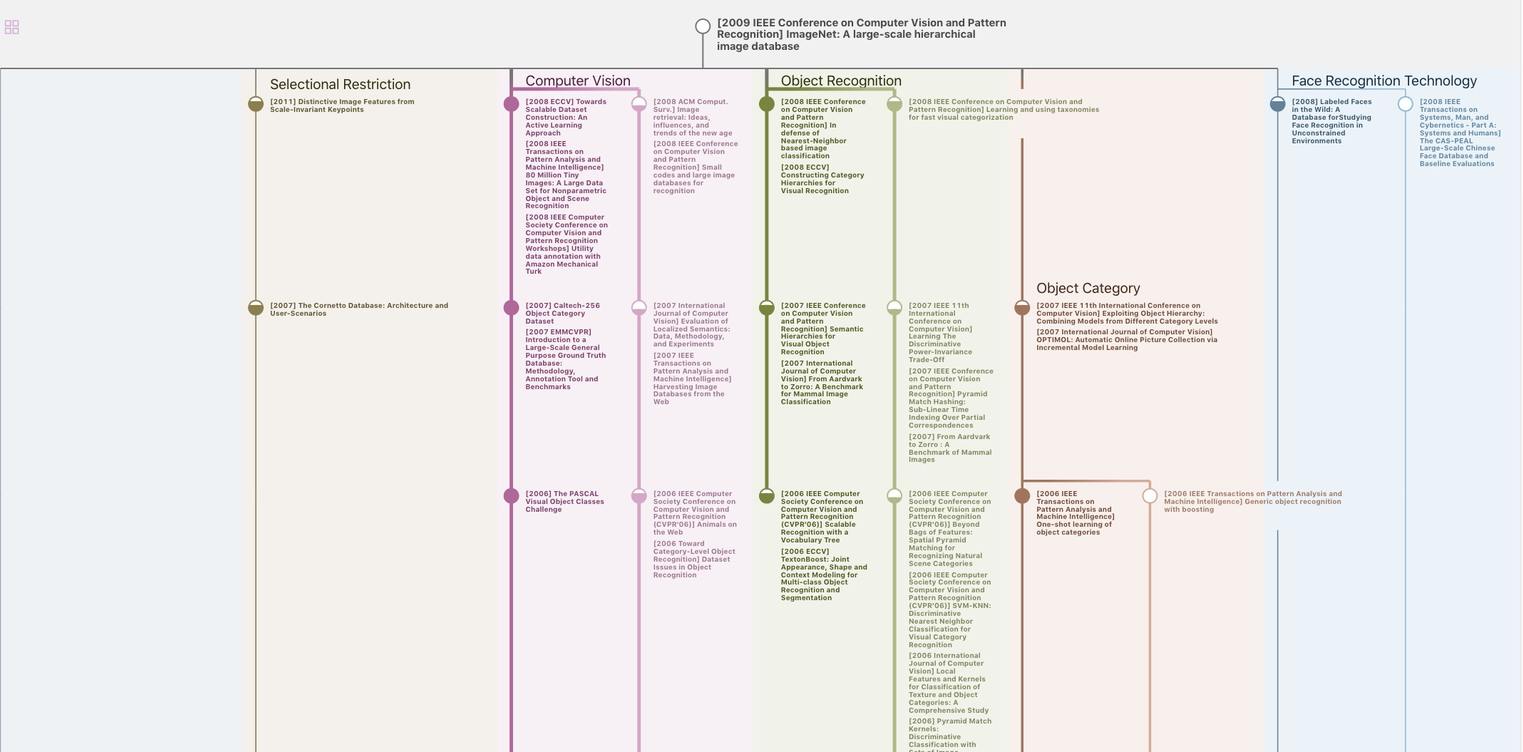
生成溯源树,研究论文发展脉络
Chat Paper
正在生成论文摘要