Transfer learning on self-supervised model for sar target recognition with limited labeled data
IGARSS 2023 - 2023 IEEE INTERNATIONAL GEOSCIENCE AND REMOTE SENSING SYMPOSIUM(2023)
摘要
Deep learning contributes to significant improvements in synthetic aperture radar (SAR) target recognition performance. Most SAR target recognition methods are based on supervised learning and require labeled SAR data. There only exists limited labeled data due to the time-consuming and laborious work of labeling, and there is still a large amount of available unlabeled radar data. Therefore, we aim to explore whether unlabeled data can provide the network with sufficient feature information and enable the network to cluster similar target features and distinguish different target features, thereby improving the SAR target recognition performance. In this paper, we propose a new framework to train a deep neural network for SAR target recognition to eliminate the need for a large amount of labeled training data. Our idea is based on transferring knowledge from a self-supervised model, where the data can train without label information. Experiments are performed on the moving and stationary target acquisition and recognition (MSTAR) benchmark dataset, and the experimental results demonstrate the improvements in recognition performance achieved by our proposed method with limited labeled data.
更多查看译文
关键词
synthetic aperture radar,transfer learning,self-supervised,target recognition
AI 理解论文
溯源树
样例
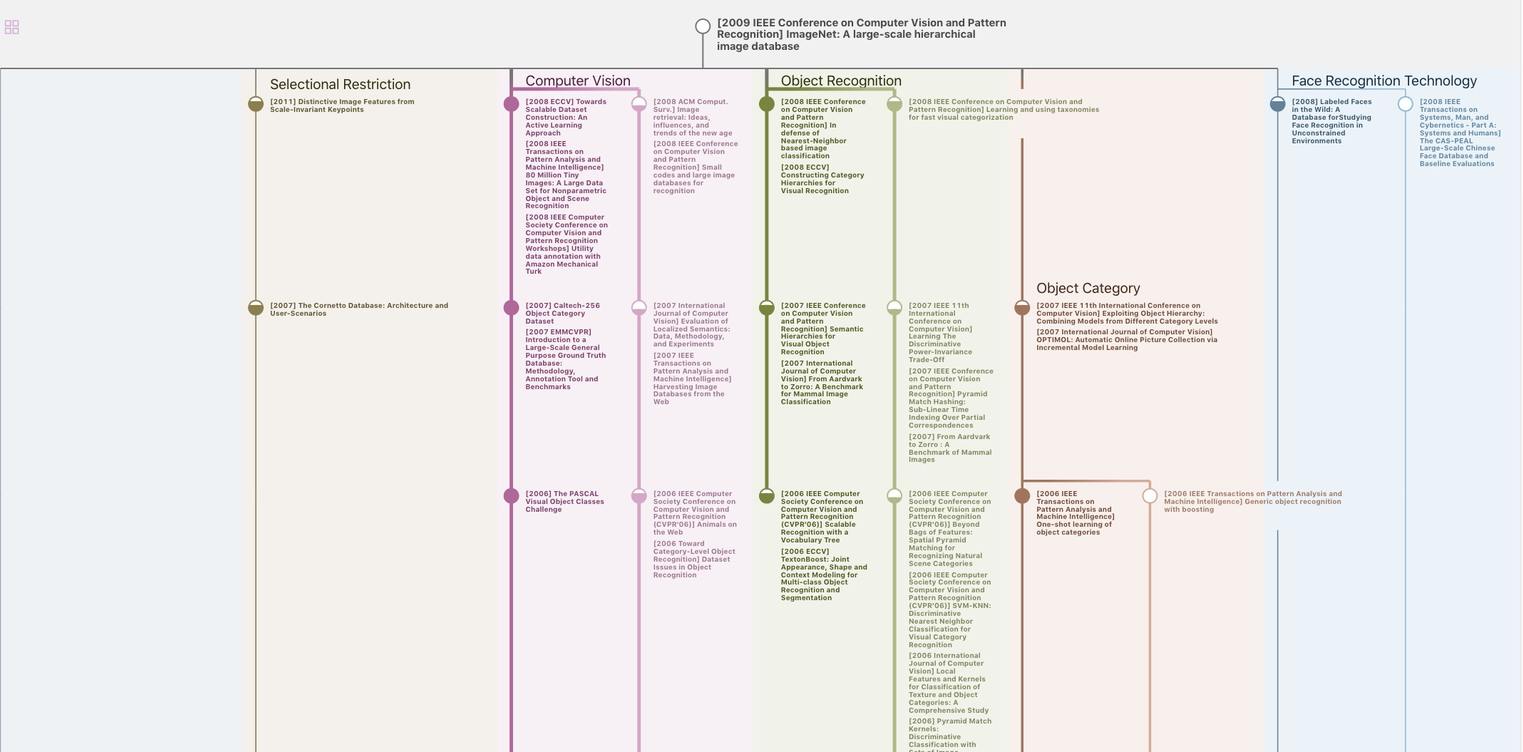
生成溯源树,研究论文发展脉络
Chat Paper
正在生成论文摘要