Machine learning for spatiotemporal mapping and monitoring of mangroves and shoreline changes along a coastal arid region
IGARSS 2023 - 2023 IEEE INTERNATIONAL GEOSCIENCE AND REMOTE SENSING SYMPOSIUM(2023)
摘要
Mangroves are coastal ecosystems with enormous ecological benefits. These coastal protectors provide a living environment to many marine organisms, and it is considered a unique contributor against climate change in their carbon storage and sequestration process. Mangroves experience severe losses due to natural factors and intensive anthropogenic activities. Therefore, mapping, monitoring, and obtaining consistent recent information about these valuable resources is essential for conservation and protection. The United Arab Emirates (UAE) is the home of sixty million mangroves covering an area of more than 180 km(2) and storing 43,000 tons of carbon dioxide yearly [1]. The mangrove area located in the coastal region of UAE provides various benefits to the region and is considered a protective shield against the risk of erosion and sea intrusion. Therefore, UAE promised in the Conference of the Parties 2026 (COP26) to plant 100 million mangroves by the year 2030 [1]. Remote sensing and digital image processing techniques had proven to understand the mangrove ecosystem dynamics. Therefore, this study aims to investigate the changes in the Mangrove area and the effect of these changes on coastal erosion hazards over the last 20 years. The first step is to use a pixel-based machine learning (ML) classifiers along with multi-temporal, medium-resolution Landsat satellite images within Google Earth Engine (GEE) cloud computing platform, to create multi-temporal mangrove distribution maps of the UAE coastal area during the last 20 years. Second, qualitative and quantitative evaluations are conducted using ground truth data to validate the robustness of the proposed methodology and the accuracy of the results. Finally, coastline analysis is carried out using open-source tools, such as Digital Shoreline Analysis System (DSAS) [2] to estimate coastline changes and analyze coastal erosion risk. This method allows the identification of the mangrove gains and losses, as well as measurement of the change of coastline (accretion and erosion) over the past 20 years. The generated maps can lead to improvements in the ecosystems' management and protection procedures. This study presents an effective workflow for mangrove detection and temporal mapping, using open-source medium-resolution satellite images, big data processing platforms, such as GEE, and open-source tools, such as DSAS.
更多查看译文
关键词
Mangrove,Land cover Land Use (LCLU),Digital Shoreline Analysis System (DSAS),Shoreline Change Envelope (SCE),Net Shoreline Movement (NSM),End Point Rate(EPR)
AI 理解论文
溯源树
样例
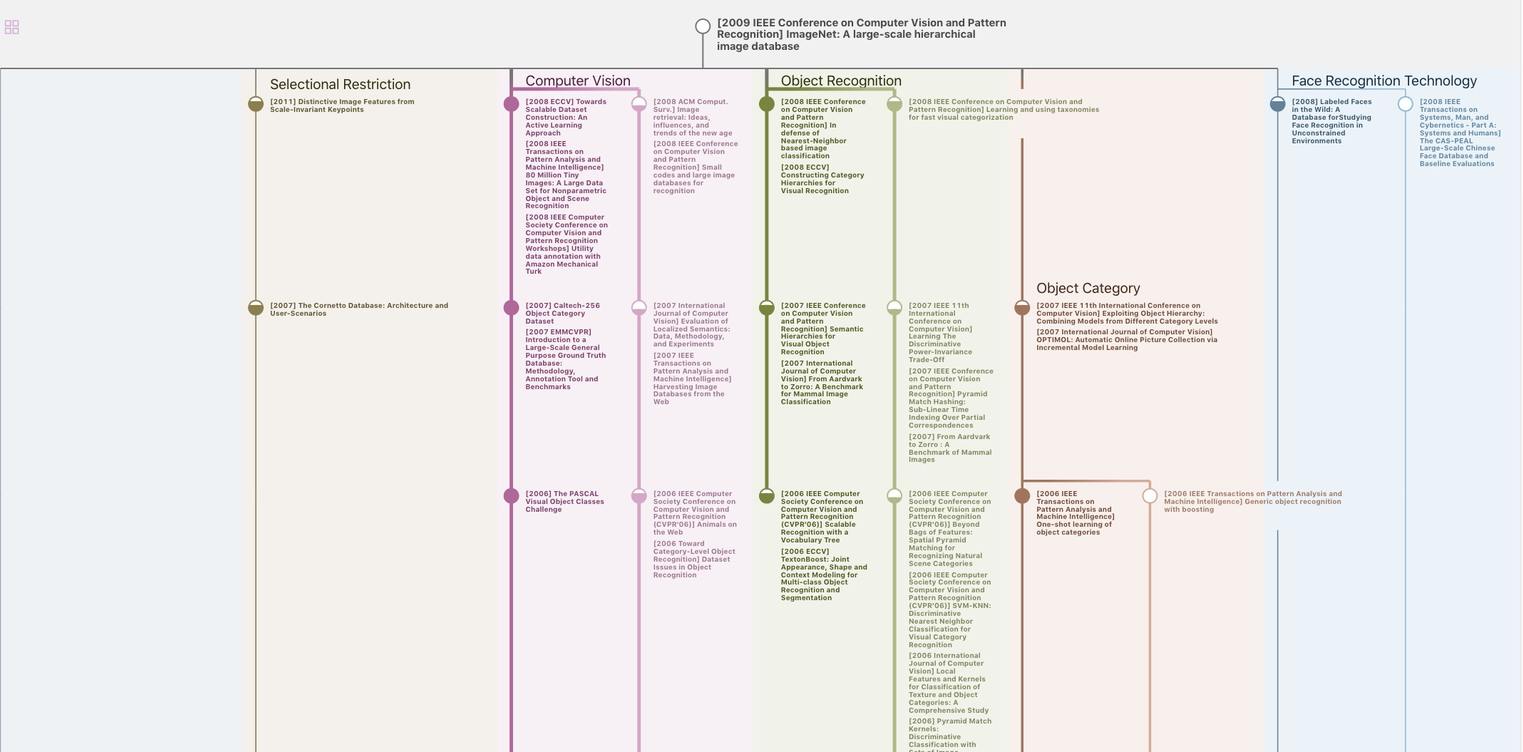
生成溯源树,研究论文发展脉络
Chat Paper
正在生成论文摘要