Inversion of radiative transfer model through one-dimensional convolutional neural network to retrieve foliar traits of temperate forest canopies with imaging spectrocopy
IGARSS 2023 - 2023 IEEE INTERNATIONAL GEOSCIENCE AND REMOTE SENSING SYMPOSIUM(2023)
摘要
Estimation of foliar traits through imaging spectroscopy is one of the current major challenge in remote sensing to monitor ecosystem functions. New spaceborne hyperspectral sensors are upcoming or already in activity. In this context, future estimation models are required to be robust to temporal variations (e.g. illumination and phenological conditions) and changes in vegetation geometry in order to cope with the wide variety of canopy observations. Our work is threefold. First, we analyze the direct radiative transfer model to highlight potentials of one-dimensional CNN (1D-CNN) to retrieve foliar traits. Secondly, we build a 1D-CNN inverse model and search for an optimal architecture. Finally, we test the retrieval performance of our 1D-CNN on four oak species over four forest sites in California at different phenological stages during two years.
更多查看译文
关键词
Foliar traits,One-dimensional CNN,PROSPECT,DART,temperate forests,AVIRIS
AI 理解论文
溯源树
样例
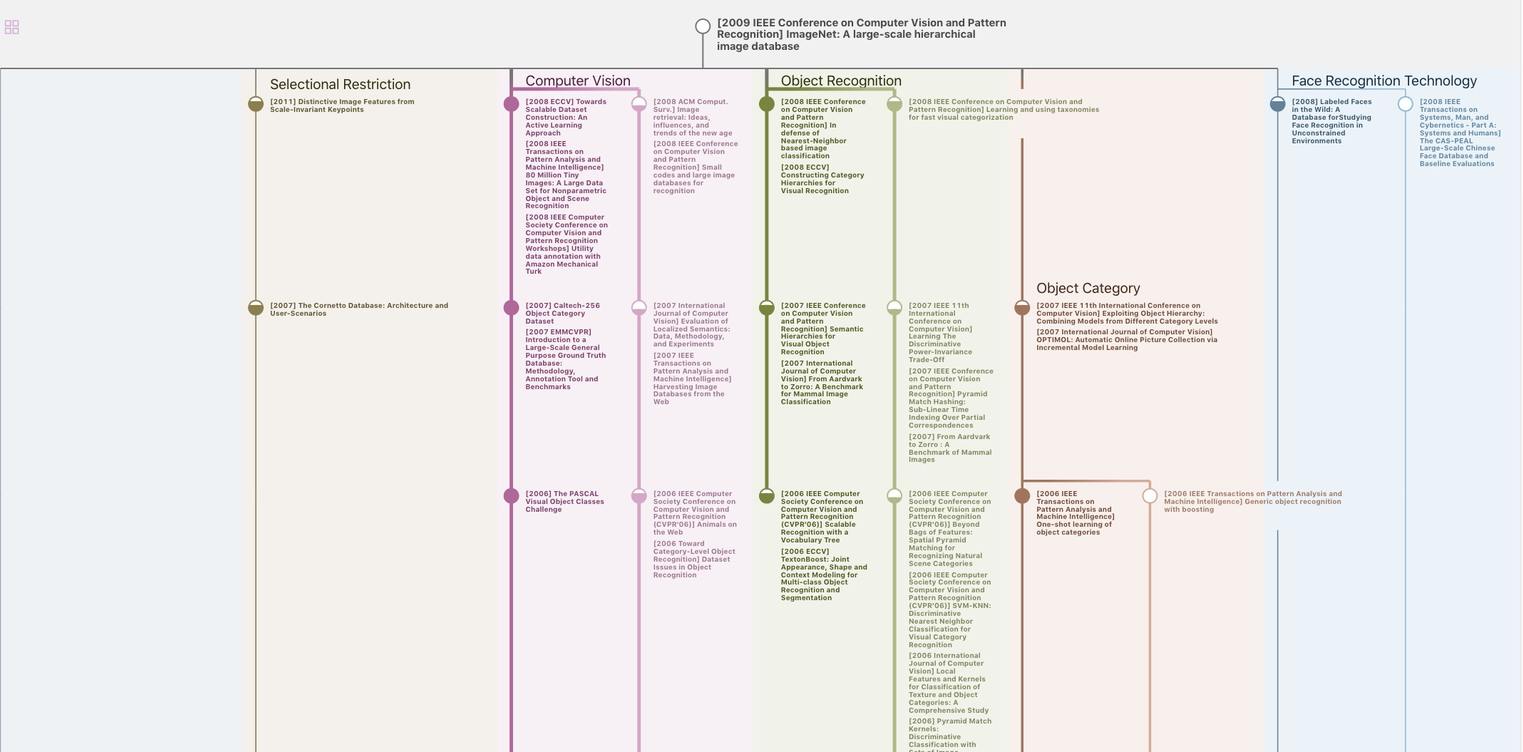
生成溯源树,研究论文发展脉络
Chat Paper
正在生成论文摘要