A framework for large scale semantic similarity search on satellite imagery
IGARSS 2023 - 2023 IEEE INTERNATIONAL GEOSCIENCE AND REMOTE SENSING SYMPOSIUM(2023)
摘要
Searching for Earth Science phenomena in large archives of Earth Observation Satellite Imagery data requires elaborate processing and spatio-temporal indexing of the images into categories of the said phenomena. Manual tagging is laborious as it needs constant monitoring through vast volumes of satellite data, the volume and velocity of which is ever-increasing. A complete re-indexing is also needed when a new phenomenon of interest is to be searched through the data archive. Previous efforts to automate tagging have leveraged Machine Learning (ML) techniques to classify images into phenomena of interest. In this method, multiple ML algorithms, each specifically trained for detecting a particular phenomenon, are used for spatio-temporal indexing. While doing so negates the need for human indexing, the process of creating ML models for identifying a class of phenomena involves significant time and computation overhead. Moreover, ML algorithms require vast amounts of extremely scarce labeled data. Furthermore, the computation needed for re-indexing the data whenever a new phenomenon is added to be tagged is not negligible. We propose an alternative, data-driven framework to search through vast amounts of satellite data, that eliminates the need for manual indexing, labeling, or creating purpose-built ML classifiers. The proposed method leverages Self-Supervised Learning (SSL) techniques to obtain feature vectors that are used for search and retrieval of satellite images. An Approximate Nearest Neighbors (ANN) algorithm is used to cluster and retrieve images exhibiting similar features, and by extension, similar Earth Science phenomena. Our unique contribution in this work is the orchestration of the methodology with various cloud services that facilitates searching through millions of images within a short span of time. To showcase the framework, we created a web interface to search through 21 years worth of daily satellite imagery with global coverage. In this paper, we discuss the progress we have made in enabling Embedding Based Search within Remote Sensing, and discuss the potential benefits and pitfalls involved in realizing this method. We also aim to provide insights and experiences we documented while developing such a system along with potential limitations of the current stage of the framework.
更多查看译文
关键词
Remote Sensing,Computer vision,Similarity Search,Vector Databases,Cloud Computing
AI 理解论文
溯源树
样例
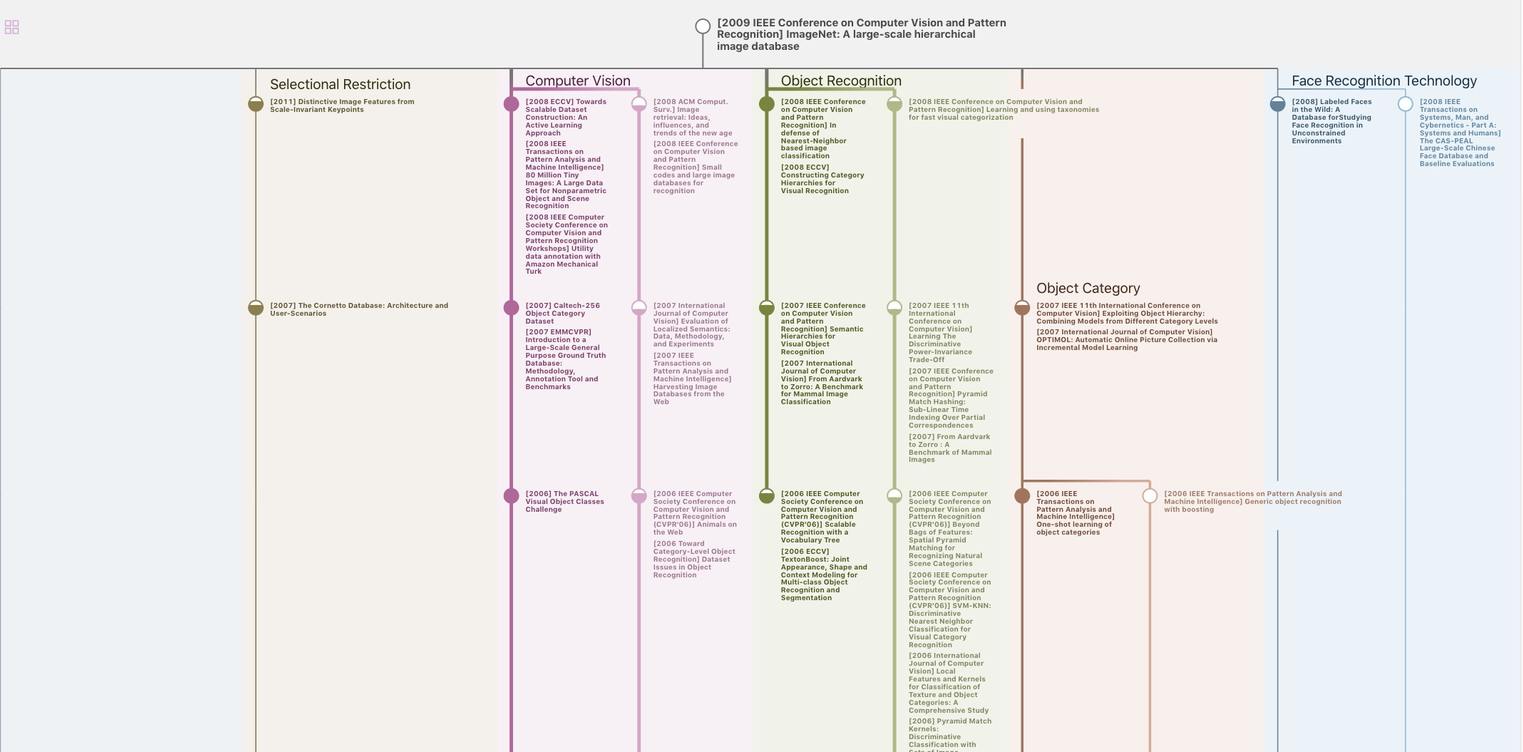
生成溯源树,研究论文发展脉络
Chat Paper
正在生成论文摘要