Self-supervised spatio-temporal representation learning of satellite image time series
IGARSS 2023 - 2023 IEEE INTERNATIONAL GEOSCIENCE AND REMOTE SENSING SYMPOSIUM(2023)
摘要
In this paper, a new self-supervised strategy for learning meaningful representations of complex optical Satellite Image Time Series (SITS) is presented. The methodology proposed named U-BARN, a Unet-BERT spAtio-temporal Representation eNcoder, exploits irregularly sampled SITS. The designed architecture allows learning rich and discriminative features from unlabelled data, enhancing the synergy between spatio-spectral and temporal dimensions. To train on unlabelled data, a time series reconstruction pretext task inspired by the BERT strategy is proposed. A Sentinel-2 large-scale unlabelled dataset is used to pre-trained U-BARN. To demonstrate its feature learning capability, representations of SITS encoded by U-BARN, are then used to generate semantic segmentation maps. Experimental results, on a labelled PASTIS dataset, corroborate that accuracies obtained by a shallow classifier using representations learned by the pre-trained model are better than results obtained by the raw SITS. Additionally, a fully supervised experiment is conducted on this same labelled PASTIS dataset to evaluate the effectiveness of the proposed U-BARN architecture. The obtained results show that U-BARN architecture reaches performances similar to the spatio-temporal baseline (U-TAE).
更多查看译文
关键词
Satellite Image Time series (SITS),Transformer,Self-Supervised Learning,Spatio-Temporal network,Unet,Representation Learning
AI 理解论文
溯源树
样例
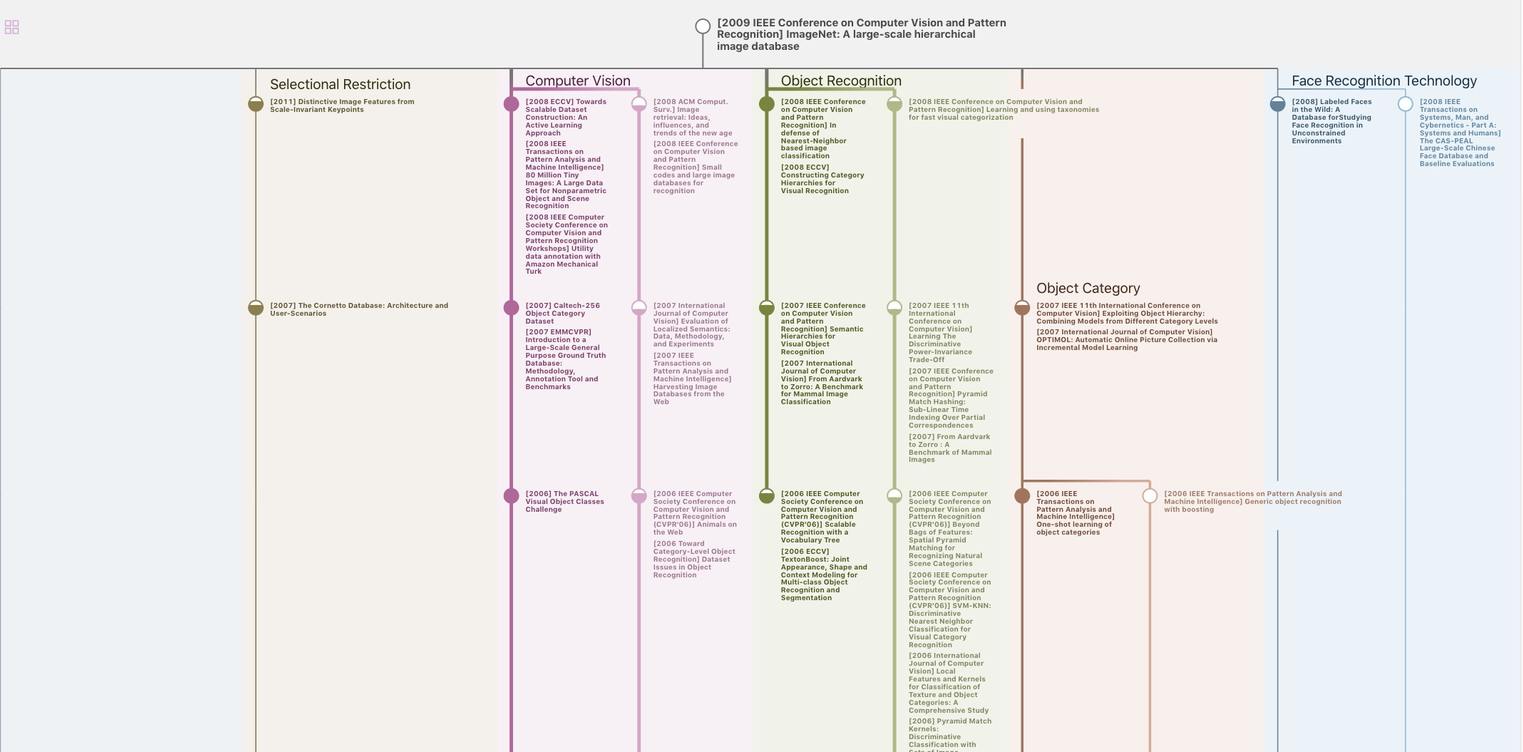
生成溯源树,研究论文发展脉络
Chat Paper
正在生成论文摘要