Enhanced Multi-Task Nasopharyngeal Carcinoma Recurrence Prediction by Knowledge Distillation with Loss-Balanced Weighting Strategy
2023 4th International Conference on Intelligent Computing and Human-Computer Interaction (ICHCI)(2023)
摘要
Recurrence of nasopharyngeal carcinoma (NPC) generally leads to a poor prognosis, and a recurrence prediction method based on Magnetic Resonance Imaging (MRI) is valuable and meaningful for clinical treatment. However, a major challenge in effective prediction is the obvious difficulty of learning both global and local anatomical information from the tumor, due to irregular spatial representations in various MRI data. To address these issues, we construct a hard-parameter-sharing multi-task network for NPC recurrence prediction enhanced by Similarity-Preserving Knowledge Distillation (SPKD) with loss-balanced weighting strategy. In our method, the backbone generates global anatomical information and the auxiliary segmentation task extracts local anatomical information. Besides, through SPKD, the valuable hidden representations of single-task predictive network are distilled into the primary network and ease the negative transfer in the multi-task framework. Furthermore, we explore that the loss-balanced weighting strategy might not only reduce negative transfer but also constrain distillation of over-confidence knowledge, and the proper distilling layers positions in our framework encourage positive learning in specific knowledge-sharing tasks. Experiments demonstrate that the proposed multi-task learning (MTL) framework enhanced by similarity distillation knowledge distillation with loss-balanced weighting algorithm improves the overall performance.
更多查看译文
关键词
component,NPC recurrence prediction,knowledge distillation,multi-task learning,loss-balanced weight
AI 理解论文
溯源树
样例
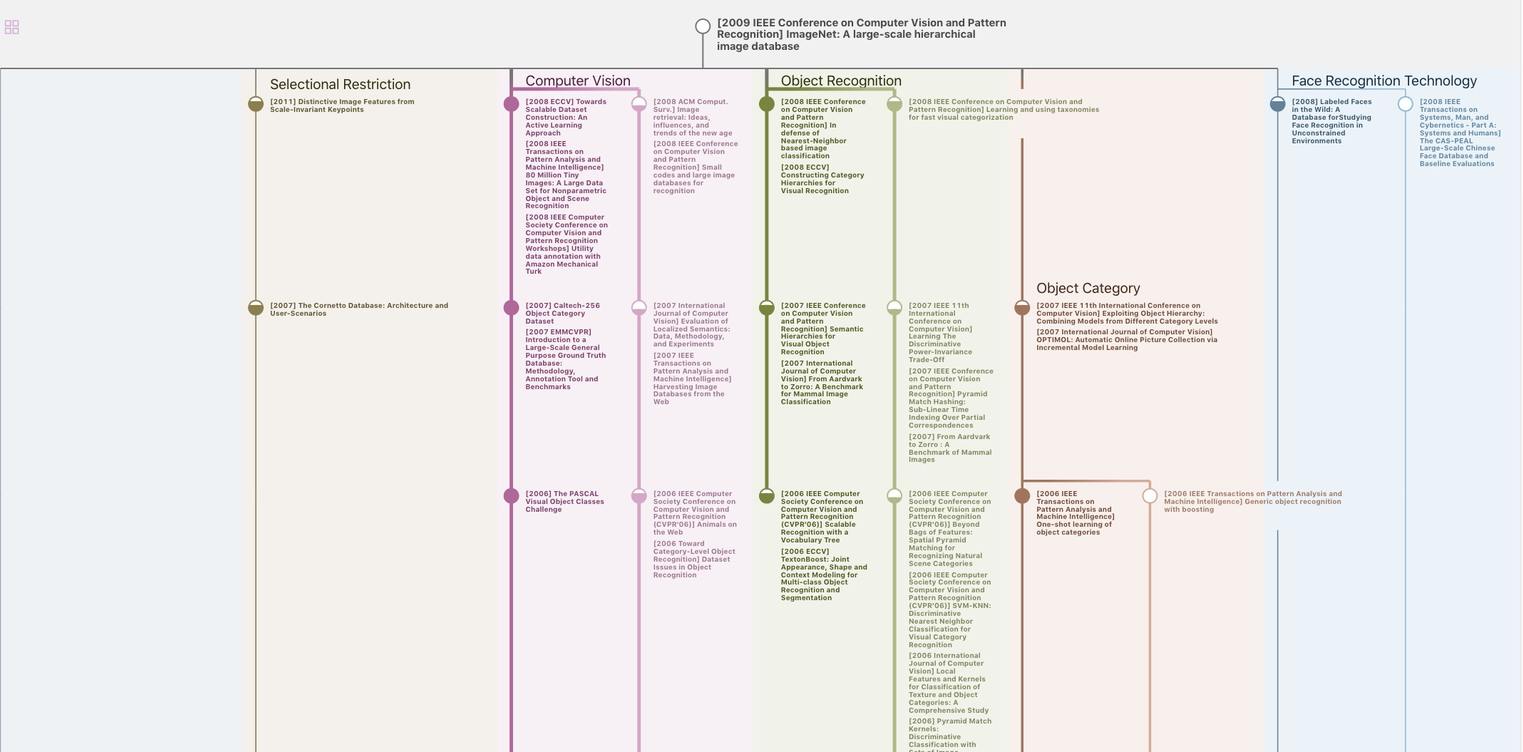
生成溯源树,研究论文发展脉络
Chat Paper
正在生成论文摘要