Real-World Data-Driven Electric Vehicle Charge and Discharge Planning with Deep Q-Network
2023 IEEE International Conference on Environment and Electrical Engineering and 2023 IEEE Industrial and Commercial Power Systems Europe (EEEIC / I&CPS Europe)(2023)
摘要
With the increasing utilization of electric vehicles (EVs) for transportation, it has become crucial to effectively manage its battery charging and discharging processes. Smart charging and discharging strategies considering Vehicle-to-Grid (V2G) integration are instrumental in achieving this, as they contribute to cost-effective energy consumption for end users and facilitate load balancing for the grid. In this study, we develop a method using deep Q-Networks (DQN) to create smart charging and discharging plans for EVs that take into account V2G capabilities. To ensure adaptability to diverse and complex driving patterns, we train and evaluate the DQN agent using real-world driving data from various vehicles. Moreover, our approach anticipates the widespread availability of public charging infrastructure and V2G opportunities in the future, assuming that EVs can be charged and discharged at any location, not just at home. While our study has some limitations, such as not accounting for battery degradation and the need for developing models for larger battery capacities, our proposed method offers a promising step toward more efficient and sustainable EV energy management with V2G integration.
更多查看译文
关键词
EV,V2G,Deep Reinforcement Learning,Deep Q-Network,Charge/Discharge Plan
AI 理解论文
溯源树
样例
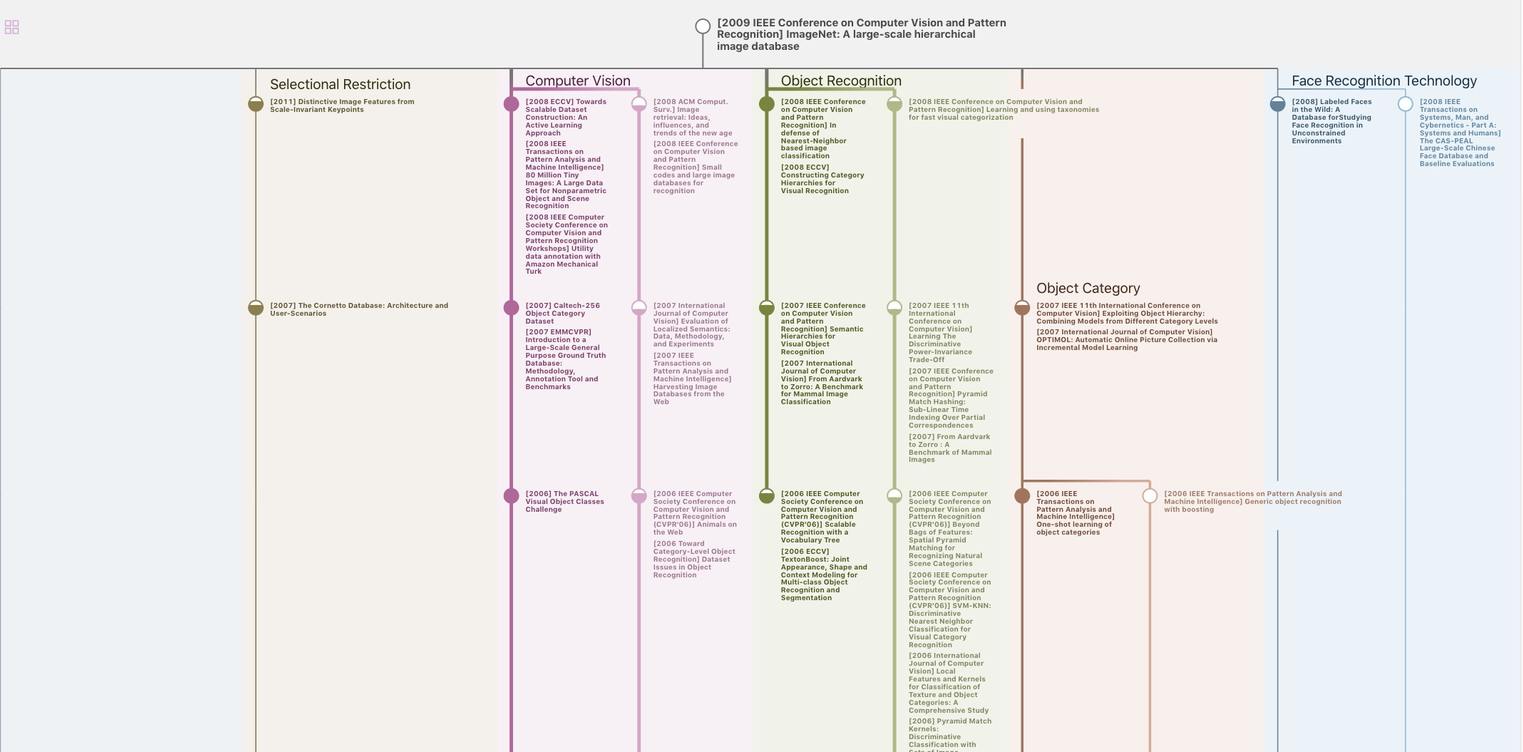
生成溯源树,研究论文发展脉络
Chat Paper
正在生成论文摘要