Suspect Recommendation For Memory Devices
2023 IEEE Women in Technology Conference (WINTECHCON)(2023)
摘要
During manufacturing of memory devices, there may be various defects which may be introduced at multiple stages of manufacturing such as fabrication, production and testing. These defects such as assembly line defects, external defects, equipment or process defects, may results in the failure of that memory device and may be identified using automated or manual mass production tests. For large-scale manufacturing, manual inspection of these defects is time-inefficient and error-prone. This process of identifying probable causes of failure in embedded memory devices is known as suspect recommendation. In this paper, a machine learning approach to identify defective device lots from non-defective device lots is proposed. The proposed algorithm consolidates the predictions from three different feature importance methods such that critical features affecting the manufacturing memory devices can be identified with confidence. Moreover, class-specific feature importance scores are derived from the three feature importance methods so that critical features affecting defective and non-defective device lots can be detected and a novel way of combining them is proposed. Furthermore, we design a domain specific performance criterion, namely Feature Match Index (FMI) to compare the subject matter expert’s (SME) view with the critical features identified by the proposed solution and achieve an 88.89% match. The proposed solution has been deployed successfully in the large-scale production environment of memory devices.
更多查看译文
关键词
Memory devices,fault detection,suspect recommendation,machine learning,over-sampling,under-sampling,feature importance,data imbalance
AI 理解论文
溯源树
样例
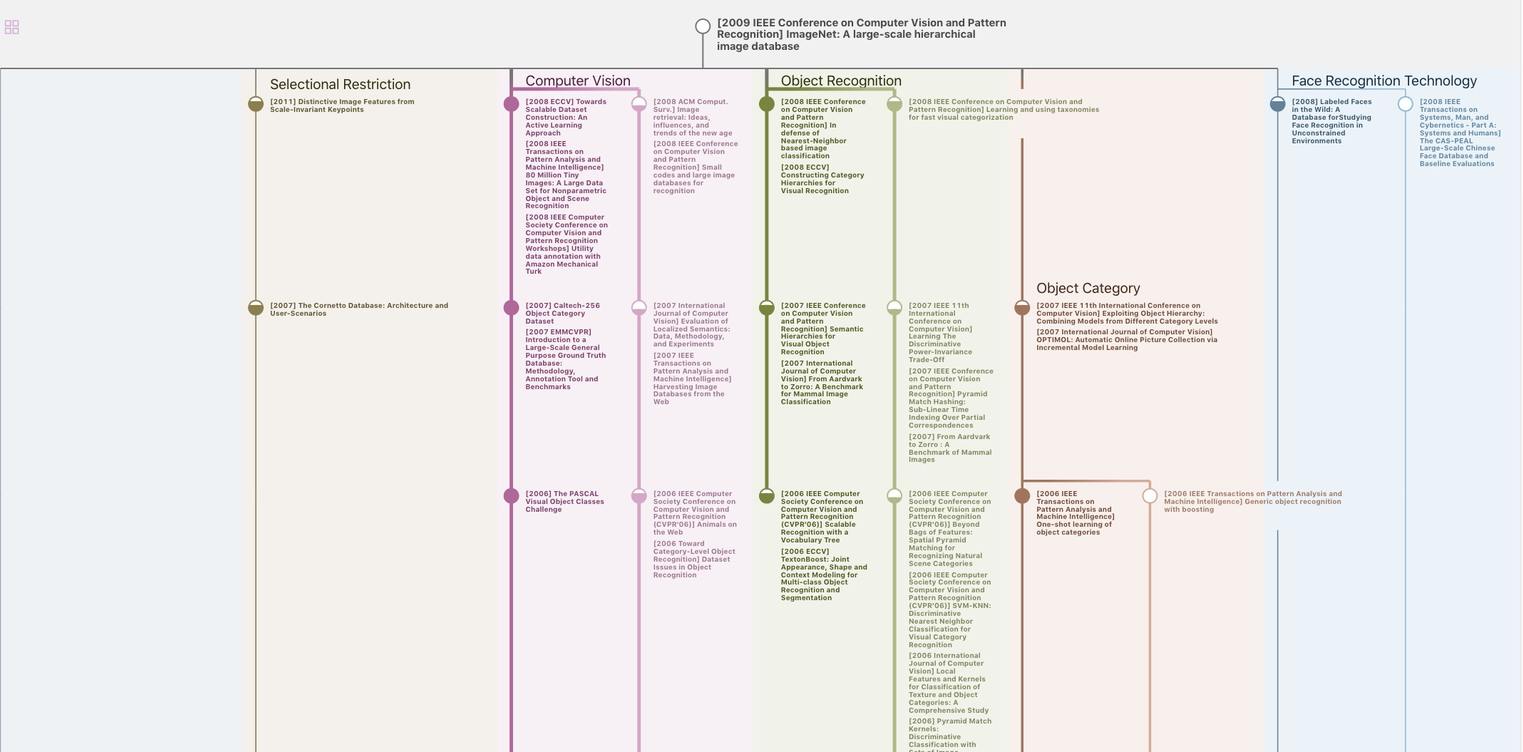
生成溯源树,研究论文发展脉络
Chat Paper
正在生成论文摘要