Anomaly Detection for Multi-Zone Buildings Using Cluster-Trained LSTM Autoencoders
2023 9th International Conference on Control, Decision and Information Technologies (CoDIT)(2023)
摘要
The optimal energy performance of building operations is affected by component faults, which may go unnoticed for long periods of time. Significant energy savings can be achieved if faulty behaviors are detected and rectified in a timely manner. In this work, we adopt an unsupervised approach for anomaly detection that combines automatic data engineering using clustering methods with Long-Short Term Memory (LSTM)-based Autoencoders. First, data engineering is used to extract multiple operating modes from nominal data of building operations. Then, an LSTM-based Autoencoder is trained to capture the characteristics of non-linear and temporal dynamics for each operating mode. Finally, the ensemble of models can be used for anomaly detection after training has been completed. We benchmark variants of our approach against state-of-the-art Autoencoders for anomaly detection by using a recently developed experimental dataset provided by the ASHRAE Research Project RP-1312. Unsupervised anomaly detection is a challenging task due to the lack of faulty labels and the need to identify faults while avoiding false alarms. Our novel approach improves the average true positive rate for fault detection by 11.4% against a state-of-the-art plain LSTM Autoencoder while keeping the false alarm rate around 5 % without having to use labeled fault data.
更多查看译文
关键词
ASHRAE Research Project RP-1312,automatic data engineering,building operations,cluster-trained LSTM autoencoders,fault detection,faulty labels,labeled fault data,long-short term memory-based autoencoders,LSTM-based autoencoder,multiple operating modes,multizone buildings,operating mode,optimal energy performance,state-of-the-art autoencoders,state-of-the-art plain LSTM autoencoder,temporal dynamics,unsupervised anomaly detection
AI 理解论文
溯源树
样例
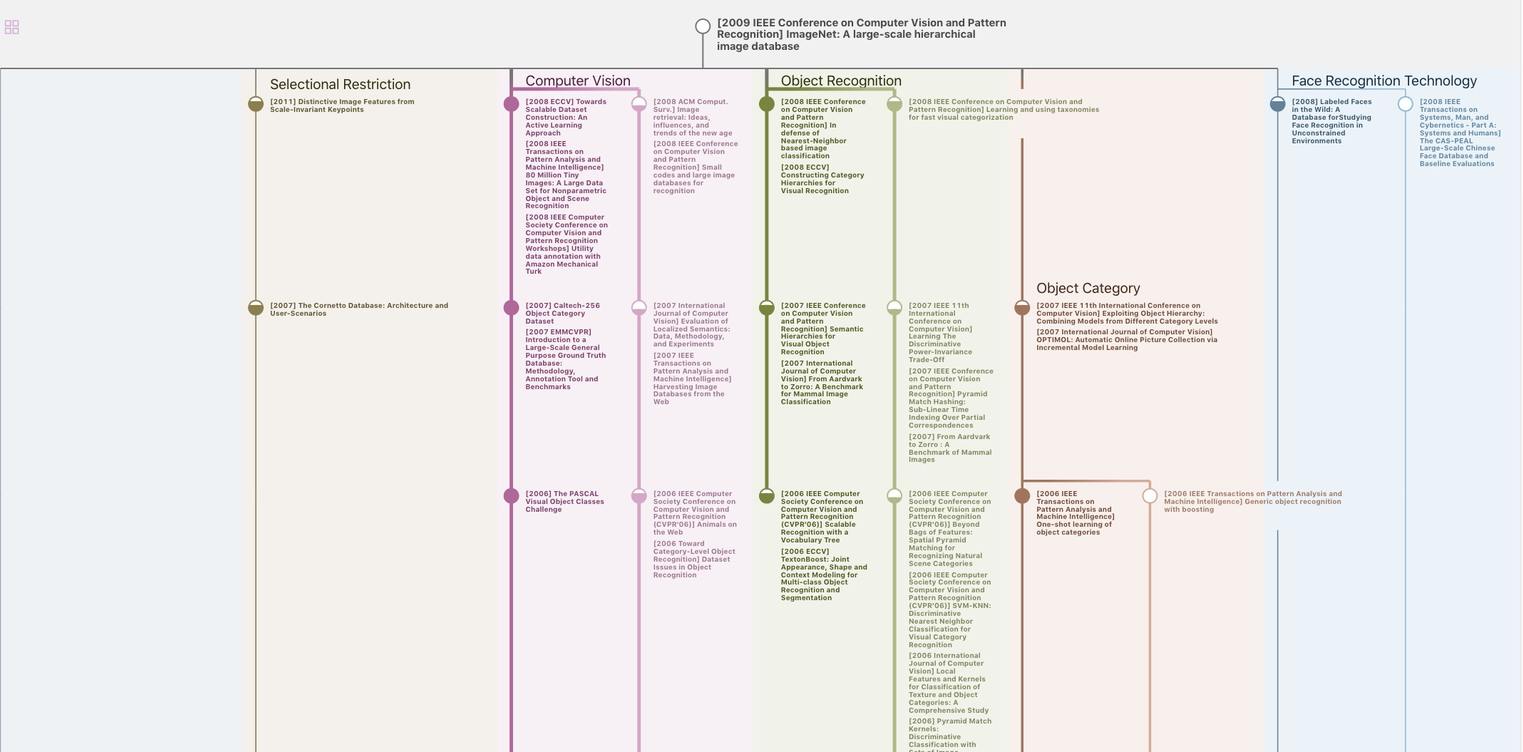
生成溯源树,研究论文发展脉络
Chat Paper
正在生成论文摘要