A novel hybrid search strategy for evolutionary fuzzy optimization approach
Neural Computing and Applications(2023)
摘要
Hybridization of metaheuristic algorithms has recently been introduced to increase the search capabilities of a traditional metaheuristic algorithms. The fuzzy optimization approach (FOA) is a metaheuristic algorithm that implements fuzzy logic systems to incorporate the knowledge and experience of an expert metaheuristic designer. This integration enables the FOA to guide the search strategy throughout the optimization process. It has been widely applied in high-dimensional problems due to its robustness. However, it presents some issues, such as a slow convergence rate, low exploration and exploitation mechanisms, and high computational effort. In this paper, hybrid search mechanisms are implemented to the original structure of FOA to increase its performance in terms of search capabilities over the limits of the fitness functions. The proposed method called ODM-FOA combines the advantages of Metropolis Hasting (MH) initialization, opposition-based learning (OBL), and Diversity measures to correctly identify and register prominent areas within the search space by approximating the fitness function and improving the exploration and exploitation of the search space. A comparison between ODM-FOA and the original FOA is implemented. Additionally, to evaluate the performance of the developed scheme, it is compared among six well-known metaheuristic algorithms on a set of benchmark functions. Experimental results demonstrate the effectiveness and robustness of the proposed approach against the other original FOA and the other methodologies in terms of solution quality, dimensionality, similarity, and convergence criteria.
更多查看译文
关键词
Hybridization,Metaheuristics,Fuzzy optimization,Metropolis hasting,Opposition-based learning
AI 理解论文
溯源树
样例
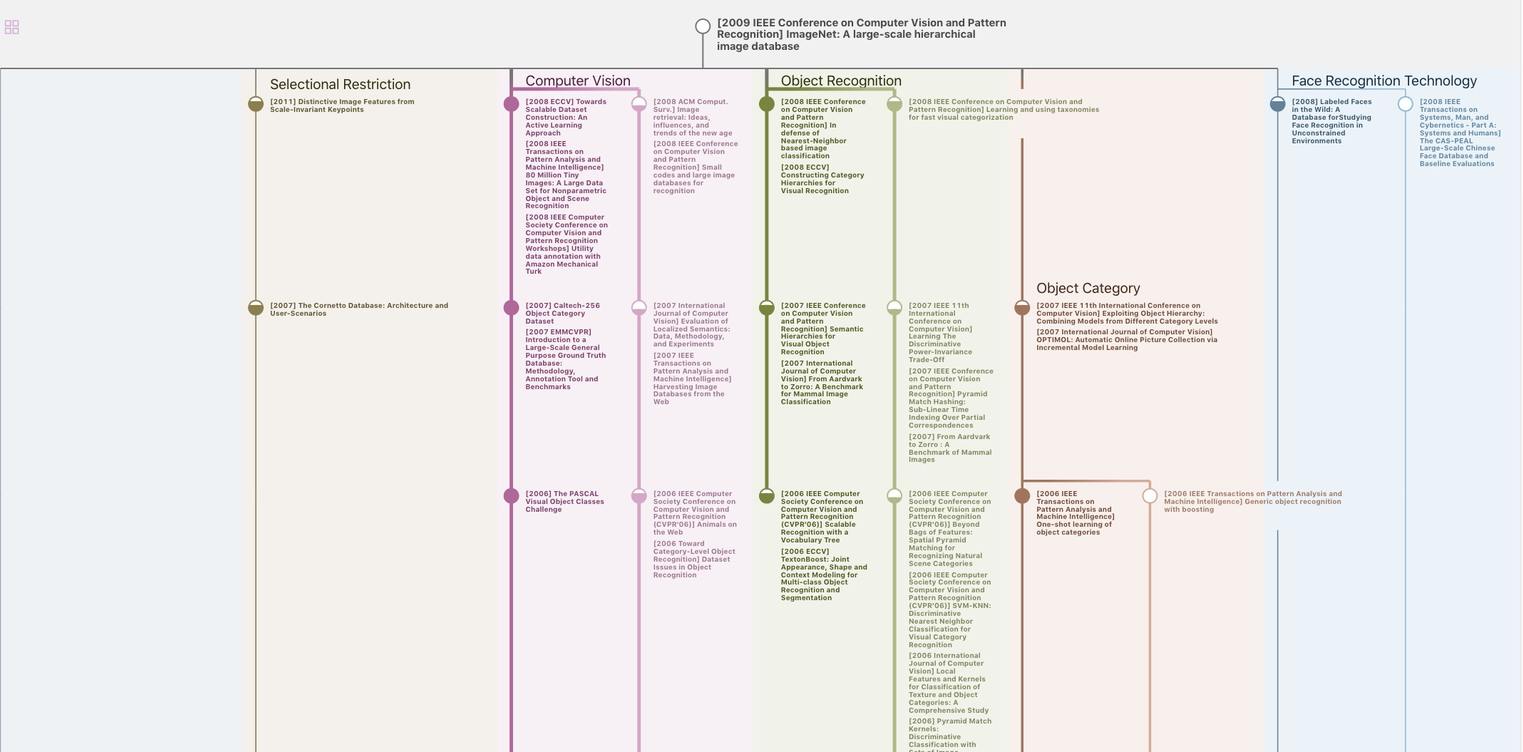
生成溯源树,研究论文发展脉络
Chat Paper
正在生成论文摘要