LDANet: the laplace-guided detail-constrained asymmetric network for real-time semantic segmentation
Multimedia Tools and Applications(2023)
摘要
The current mainstream image semantic segmentation networks often suffer from mis-segmentation, segmentation discontinuity, and high model complexity, which limit their application in real-time processing scenarios. The work established a lightweight neural network model for semantic segmentation to address this issue. The network used a dual-branch strategy to solve low semantic boundary segmentation accuracy in semantic segmentation tasks. The semantic branch applied the characteristics of the deeplabv3 + model structure. Besides, dilated convolutions with different dilation rates in the encoder were used to expand the receptive field of convolutional operations and enhance the ability to capture local features. The boundary refinement branch extracted second-order differential features of the input image through the Laplace operator, and it gradually refined the second-order differential features through a feature refinement extraction module to obtain advanced semantic features. A convolutional block attention module was introduced to filter the features from both the channel and spatial dimensions and finally fused with the semantic branch to achieve constrained segmentation boundary effects. Based on this, a multi-channel attention fusion module was proposed to aggregate features from different stages. Low-resolution features were first up-sampled and then fused with high-resolution features to enhance the spatial information of high-level features. Proposed network’s effectiveness was demonstrated through extensive experiments on the MaSTr1325 dataset, the MID dataset, the Camvid dataset, and the PASCAL VOC2012 dataset, with mIoU of 98.1, 73.1, and 81.1% and speeds of 111.40, 100.36, and 111.43 fps on a single NVIDIA RTX 3070 GPU, respectively.
更多查看译文
关键词
Semantic segmentation,Attention mechanism,Dual-branch structure,Real-time analysis,Neural network
AI 理解论文
溯源树
样例
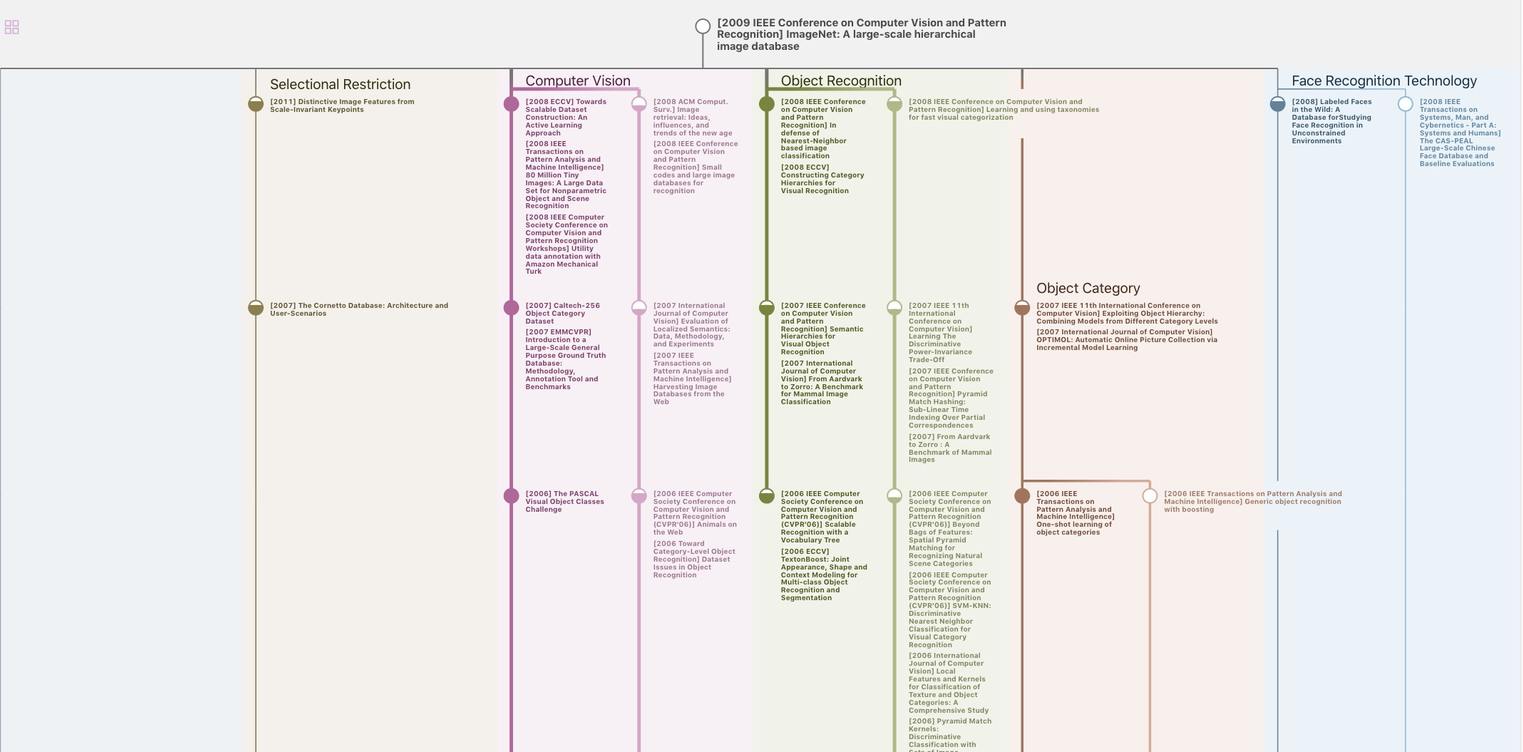
生成溯源树,研究论文发展脉络
Chat Paper
正在生成论文摘要