A Novel Graph Convolutional Gated Recurrent Unit Framework for Network-Based Traffic Prediction
IEEE ACCESS(2023)
摘要
A Smart City is characterized mainly as an efficient, technologically advanced, green, and socially informed city. An intelligent transportation system (ITS) is a subset area of smart cities that enhances the safety and mobility of road vehicles. It essentially makes travel more convenient, time-efficient and improves the citizens' quality of life. Accurate and real-time traffic prediction enables law enforcement agencies with well-informed about traffic congestion. However, accurate traffic prediction has been considered a challenging issue. Traffic prediction has restrictions on road network topology and the patterns of dynamic change in time-series data. We propose a novel deep learning framework GCST-GRU, called graph convolutional Spatio-temporal gated recurrent unit, to determine the next traffic state from traffic data. The proposed model learns complex topological structures by capturing a) spatial dependencies from data by using the graph convolution operator, and b) temporal dependencies by using the GRU neural network. Experimental results demonstrate that our framework can obtain complex Spatio-temporal correlations efficiently from the traffic network and perform better than state-of-the-art baseline models on a real-world traffic dataset. The graphical visualization by using convolution operation over the neural network shows that the model outperforms the reachability of the 3-hops neighbor effect in the traffic data graph. Additionally, the training time of the proposed framework is better than the existing state-of-the-art deep learning studies.
更多查看译文
关键词
Intelligent transportation system,traffic flow prediction,traffic network graph,gated recurrent unit,hyperparameters optimization,deep learning
AI 理解论文
溯源树
样例
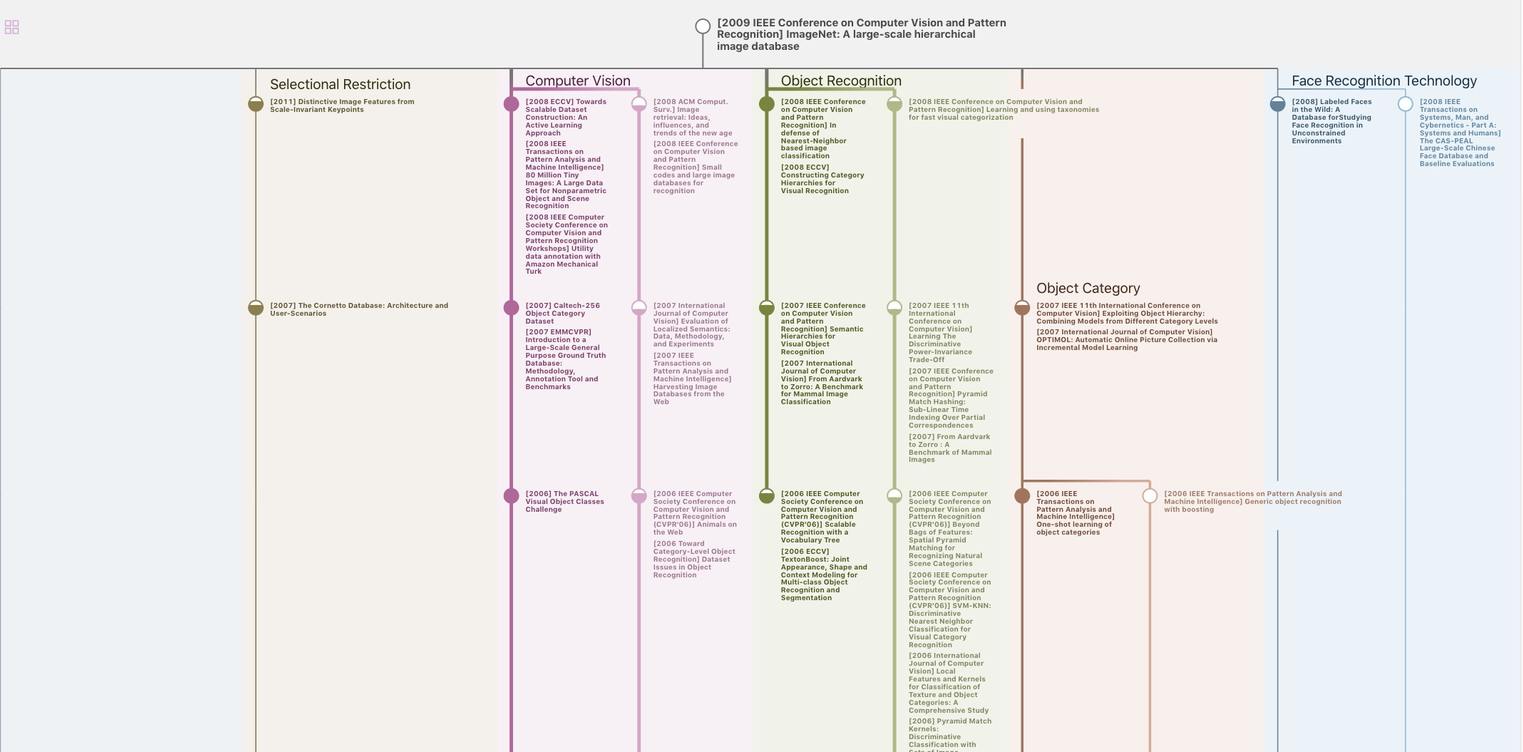
生成溯源树,研究论文发展脉络
Chat Paper
正在生成论文摘要