Aligning Non-Causal Factors for Transformer-Based Source-Free Domain Adaptation
2024 IEEE/CVF Winter Conference on Applications of Computer Vision (WACV)(2023)
摘要
Conventional domain adaptation algorithms aim to achieve better
generalization by aligning only the task-discriminative causal factors between
a source and target domain. However, we find that retaining the spurious
correlation between causal and non-causal factors plays a vital role in
bridging the domain gap and improving target adaptation. Therefore, we propose
to build a framework that disentangles and supports causal factor alignment by
aligning the non-causal factors first. We also investigate and find that the
strong shape bias of vision transformers, coupled with its multi-head
attention, make it a suitable architecture for realizing our proposed
disentanglement. Hence, we propose to build a Causality-enforcing Source-Free
Transformer framework (C-SFTrans) to achieve disentanglement via a novel
two-stage alignment approach: a) non-causal factor alignment: non-causal
factors are aligned using a style classification task which leads to an overall
global alignment, b) task-discriminative causal factor alignment: causal
factors are aligned via target adaptation. We are the first to investigate the
role of vision transformers (ViTs) in a privacy-preserving source-free setting.
Our approach achieves state-of-the-art results in several DA benchmarks.
更多查看译文
关键词
Algorithms,Machine learning architectures,formulations,and algorithms,Algorithms,Image recognition and understanding
AI 理解论文
溯源树
样例
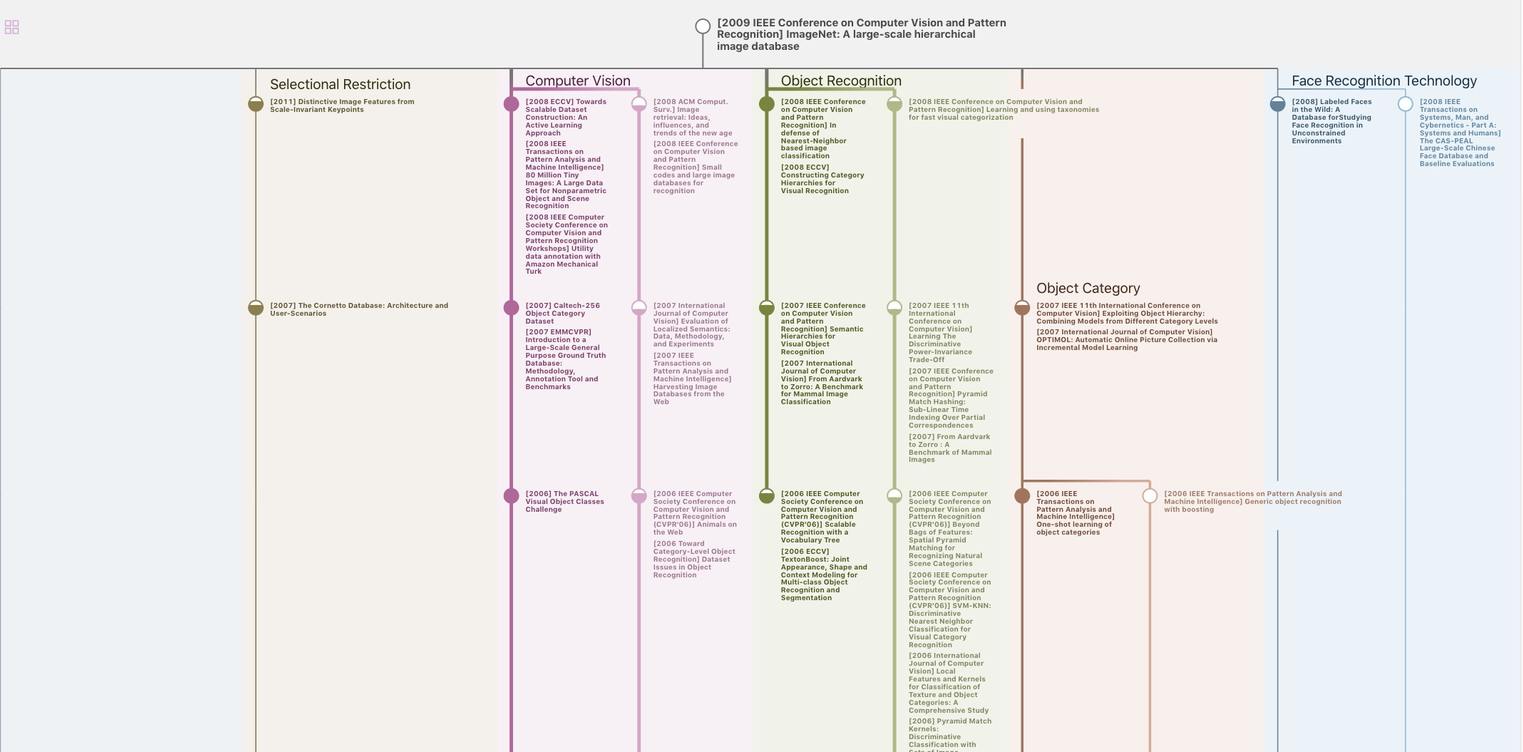
生成溯源树,研究论文发展脉络
Chat Paper
正在生成论文摘要