Space-Time Variable Density Samplings for Sparse Bandlimited Graph Signals Driven by Diffusion Operators
ICASSP 2023 - 2023 IEEE International Conference on Acoustics, Speech and Signal Processing (ICASSP)(2023)
摘要
We consider the space-time sampling and reconstruction of sparse bandlimited graph signals driven by a heat diffusion process. In this paper, we develop a sampling framework consisting of selecting a small subset of space-time nodes at random according to some probability distribution, generalizing the classical variable density sampling to the heat diffusion field. We show that the number of space-time samples required to ensure stable recovery depends on an incoherence parameter determined by the interplay between graph topology, temporal dynamics, and sampling probability distributions. In optimal scenarios, as few as $\mathcal{O}\left( {s\log k} \right)$ space-time samples are sufficient to ensure accurate recovery of all k-bandlimited graph signals that are additionally s-sparse. Our proposed sampling method requires much fewer spatial samples than the static case by leveraging temporal information. Finally, we test our sampling techniques on a wide variety of graphs. The numerical results on synthetic and real climate data sets support our theoretical findings and demonstrate the practical applicability.
更多查看译文
关键词
Graph signal recovery,sampling theo-rem,sparse signals,random space-time sampling,compres-sive sensing
AI 理解论文
溯源树
样例
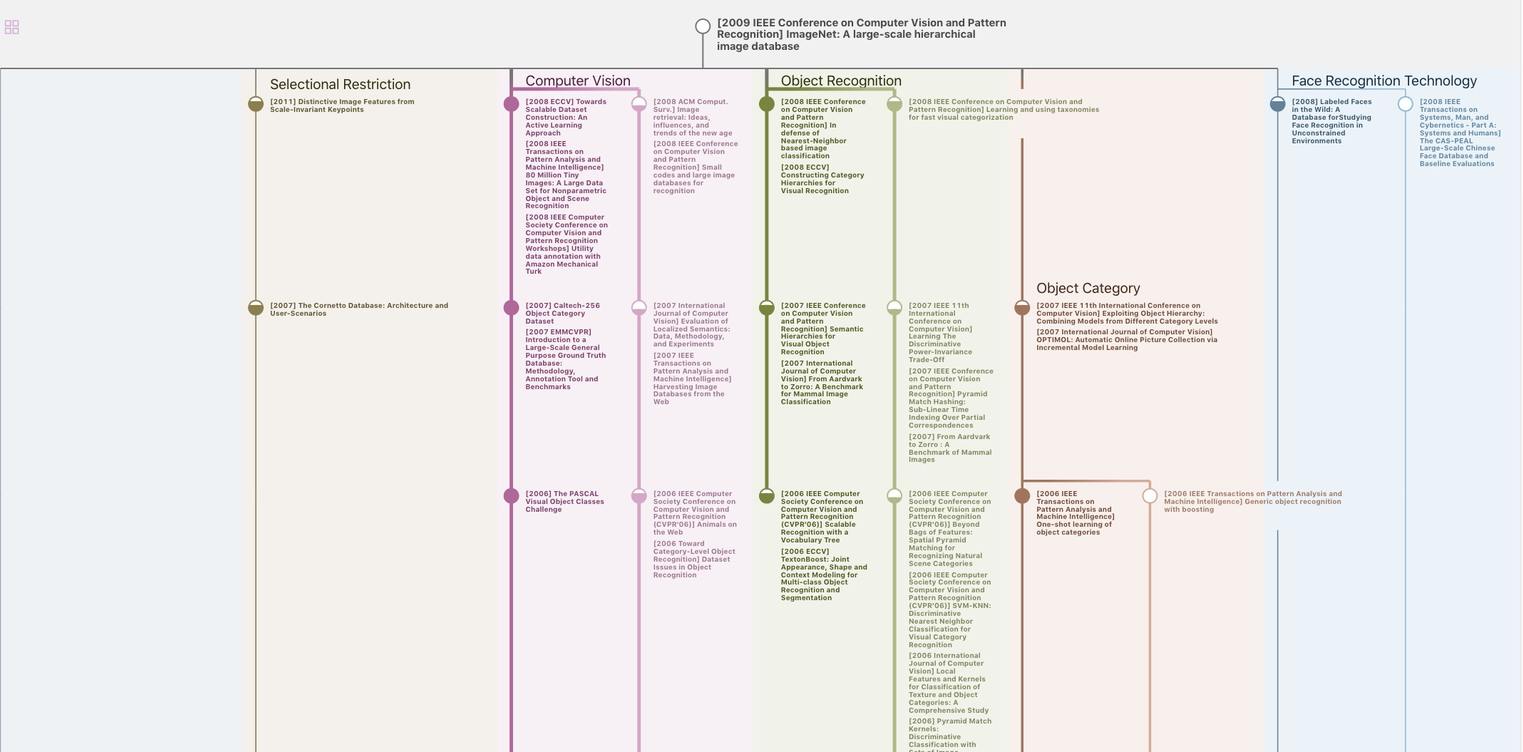
生成溯源树,研究论文发展脉络
Chat Paper
正在生成论文摘要