Noise-Aware Target Extension with Self-Distillation for Robust Speech Recognition
ICASSP 2023 - 2023 IEEE International Conference on Acoustics, Speech and Signal Processing (ICASSP)(2023)
摘要
Data augmentation using additive noise is a framework for robustly training automatic speech recognition models. To utilize noise information efficiently, previous studies used an additional branch to classify noise conditions. This added branch has a limited effect on the ASR because it performs independently of the ASR branch that classifies senones. In this paper, we propose a noise-aware target extension (NATE) that extends the senone target to contain noise awareness by jointly classifying the senone and noise in a single branch. In the inference stage, the output of the model is processed separately by the noise condition and then aggregated to match the senone posterior distribution. In addition, we combine NATE with self-distillation (NATE
sd
) to reduce the model parameters and avoid discrepancies between the outputs of training and inference. The effectiveness of the NATE method is validated on the two benchmark development and evaluation sets and simulated noisy test sets, resulting in significant improvements over the previous methods.
更多查看译文
关键词
Automatic speech recognition,data augmentation,self-distillation
AI 理解论文
溯源树
样例
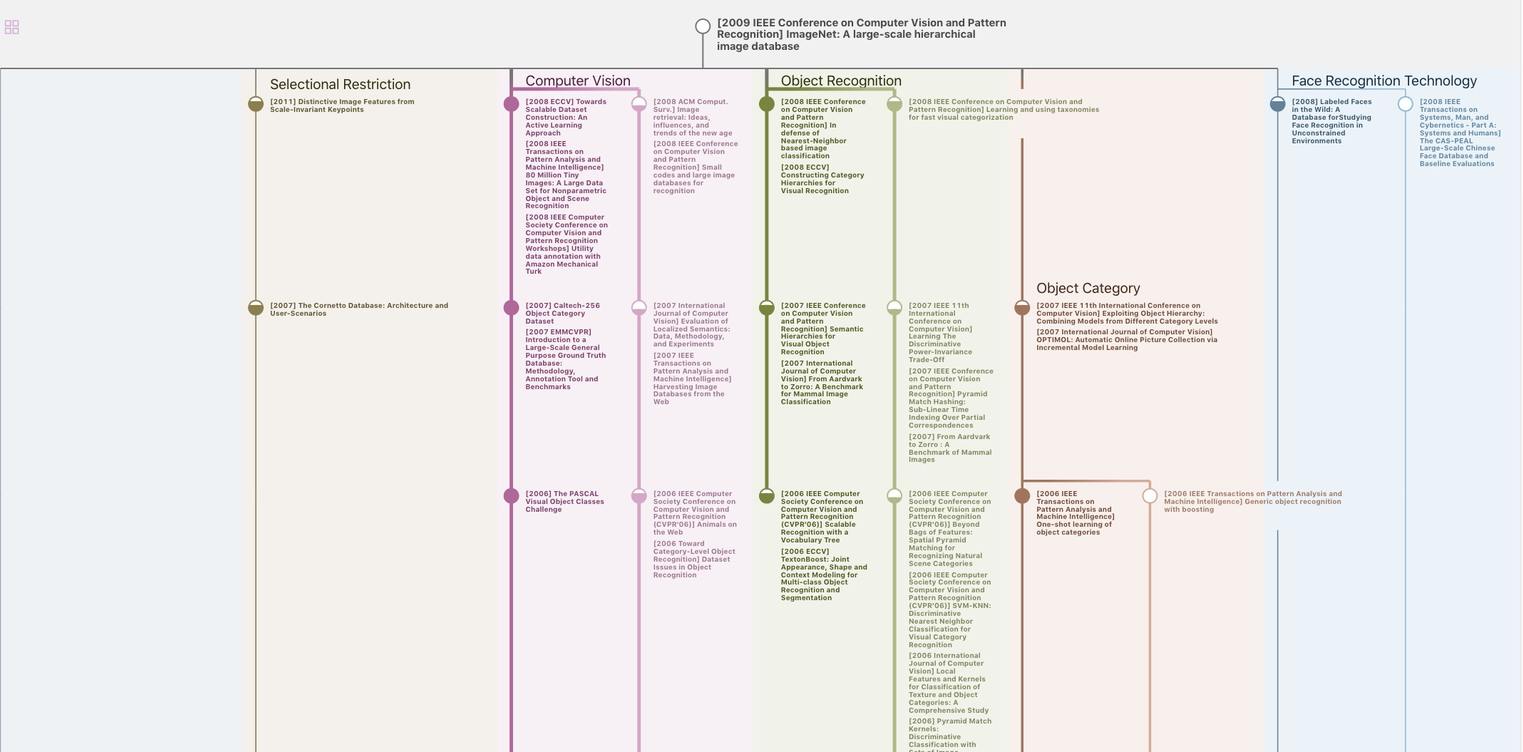
生成溯源树,研究论文发展脉络
Chat Paper
正在生成论文摘要