Adaptive Scale and Spatial Aggregation for Real-Time Object Detection
ICASSP 2023 - 2023 IEEE International Conference on Acoustics, Speech and Signal Processing (ICASSP)(2023)
摘要
Cutting-edge real-time detectors usually reach real-time performance by adopting lightweight architectures. The accuracy of detection may be limited by their insufficient capabilities to obtain powerful feature representation, which is a notoriously onerous task in machine vision applications. Aiming at this problem, this study proposes a method of adaptive aggregation of features at both scale and spatial levels in an anchor-free framework: 1) at the scale level, a Multi-scale Point Feature Fusion (MPFF) module has been proposed to fuse point features from multiple scales via a self-adaptive re-weighting manner; 2) at the spatial level, a Restrained Deformable Convolution (R-DCN) has been designed to focus on the most informative features in a pre-defined region while avoiding the remote feature distraction. Based on R-DCN, an Adaptive Spatial Aggregation (ASA) module has been presented to alleviate the feature misalignment problem in classification and regression tasks via their respective spatial divisions. Extensive experimental results on MS COCO indicate that Adaptive Aggregation Detector (AADet) achieves a state-of-the-art detection performance, i.e., 41.8 AP at 60 FPS.
更多查看译文
关键词
Real-time,Object detection,Anchor-free,Adaptive Feature Aggregation
AI 理解论文
溯源树
样例
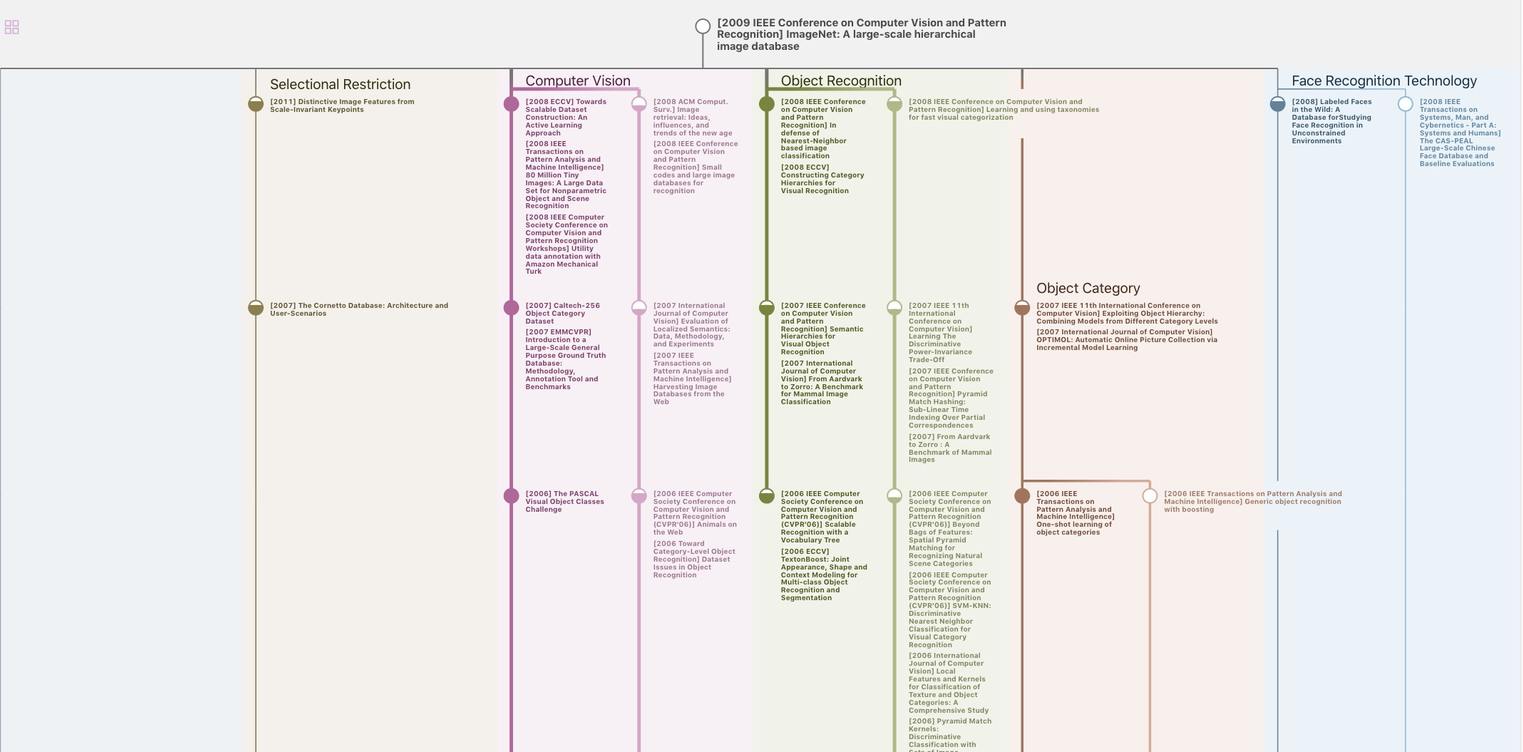
生成溯源树,研究论文发展脉络
Chat Paper
正在生成论文摘要