One-Shot Action Detection via Attention Zooming In
ICASSP 2023 - 2023 IEEE International Conference on Acoustics, Speech and Signal Processing (ICASSP)(2023)
摘要
Hinted by a modest support set, few-shot action detection (FSAD) aims at localizing the action instances of unseen classes within an untrimmed query video. Existing FSAD techniques mostly rely on generating a set of class-agnostic action proposals from the query video and then finding the most plausible ones by assessing their correlation to the support set. Such two-stage approaches are feasible but not efficient, largely due to neglecting the support information in generating the proposals. This work focuses on the one-shot image scenario and introduces the attention zooming in strategy to effectively and progressively carry out support-query cross-attention while generating proposals. The resulting one-stage model yields high-quality action proposals for boosting one-shot action detection (OSAD) performance. Our extensive experiments on the ActivityNet-1.3 and THUMOS-14 datasets demonstrate that the proposed framework can achieve state-of-the-art performance in tack-ling challenging image-based OSAD tasks.
更多查看译文
关键词
Few-shot action detection,attention
AI 理解论文
溯源树
样例
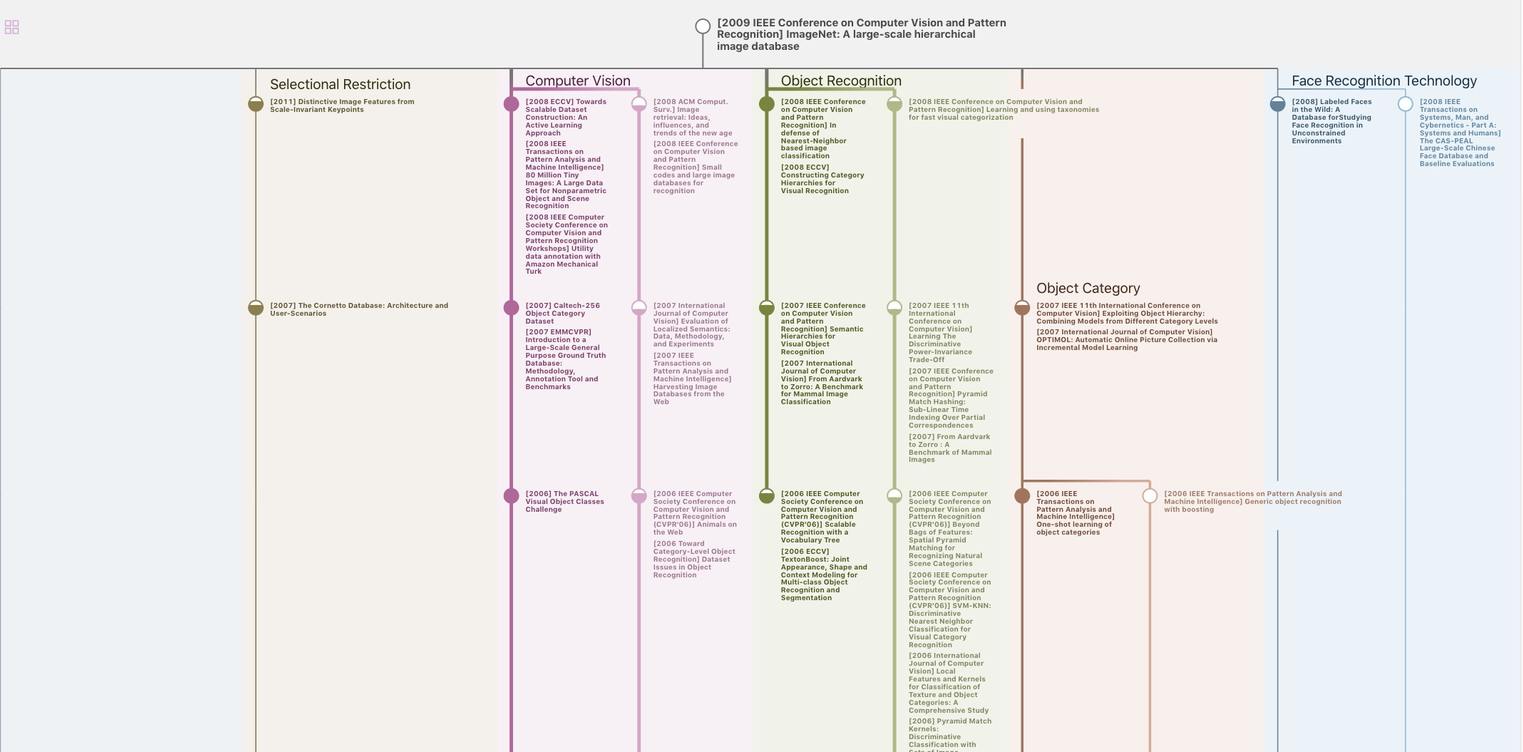
生成溯源树,研究论文发展脉络
Chat Paper
正在生成论文摘要