Difficulty-Aware Data Augmentor for Scene Text Recognition
ICASSP 2023 - 2023 IEEE International Conference on Acoustics, Speech and Signal Processing (ICASSP)(2023)
摘要
Deep neural network (DNN) based scene text recognition (STR) methods usually require a large amount of annotated data for training, which is time-consuming and cost-expensive in practice. To address this issue, many data augmentation methods have been developed to train recognizers by improving the diversity of training samples. However, most existing methods neglect the difficulty inherent in samples, and easily suffer from the problem of over-diversity, i.e., the distribution of the augmented data significantly deviates from that of clean data. In this paper, we propose a novel difficulty-aware data augmentation framework for scene text recognition, which jointly considers the difficulty of samples and the strength of augmentations. Specifically, our framework first predicts the sample difficulty, followed by an adaptive data augmentation strategy. Furthermore, we build a more diverse set of augmentation methods for STR and integrate it into our augmentation framework. Extensive experiments on scene text recognition benchmarks show that our augmentation framework significantly improves the performance of recognizers.
更多查看译文
关键词
Scene text recognition,data augmentation,difficulty-aware,deep neural network
AI 理解论文
溯源树
样例
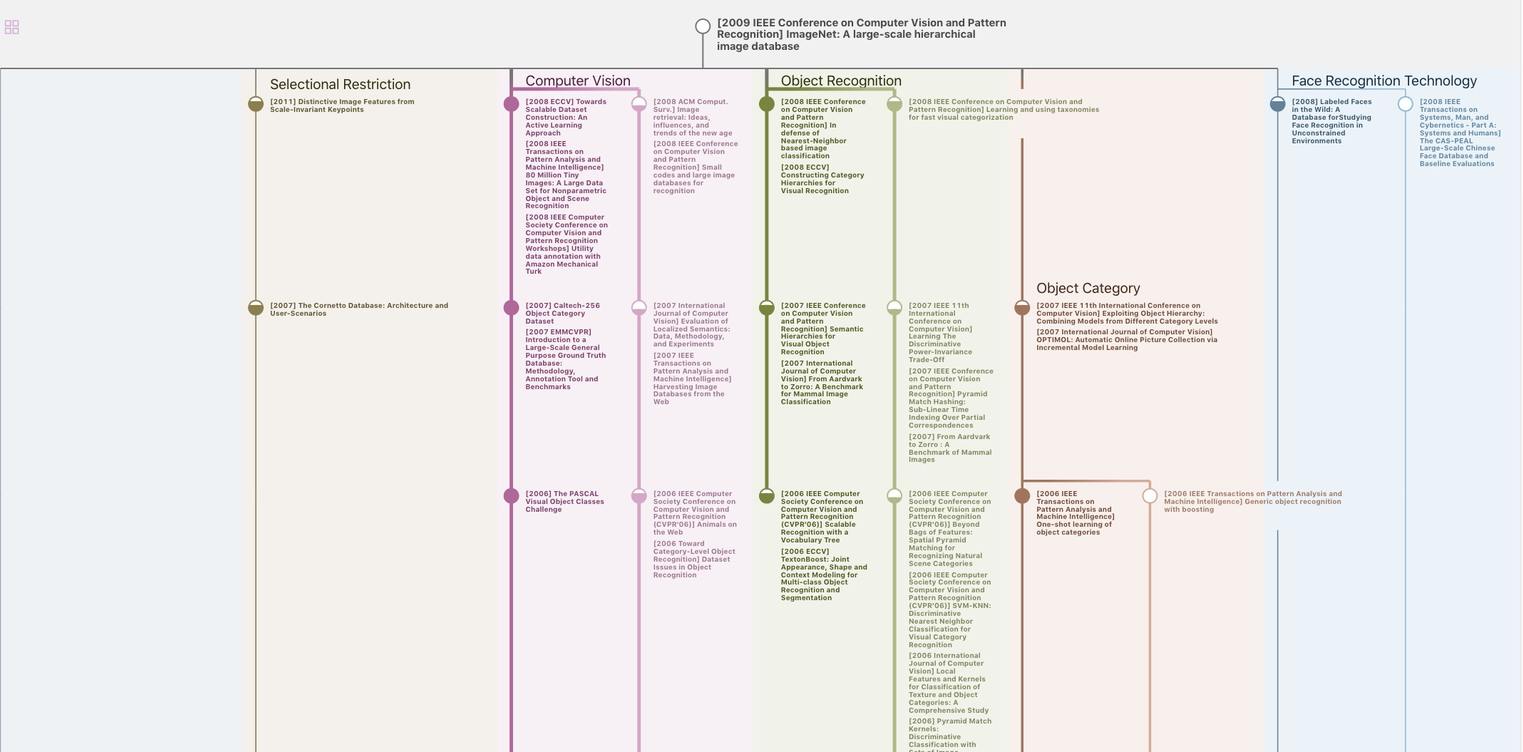
生成溯源树,研究论文发展脉络
Chat Paper
正在生成论文摘要