Loss Function Design for DNN-Based Sound Event Localization and Detection on Low-Resource Realistic Data
ICASSP 2023 - 2023 IEEE International Conference on Acoustics, Speech and Signal Processing (ICASSP)(2023)
摘要
This study focuses on the design of a loss function for a deep neural network (DNN)-based model with two branches, which is used to solve sound event localization and detection (SELD) on low-resource realistic data. To this end, we employ a secondary network for audio classification, which provides global event information to the main network, enabling it to make robust SELD predictions. Furthermore, we suggest utilizing a momentum strategy for direction-of-arrival (DOA) estimation, taking advantage of the strong temporal consistency of sound events, thereby effectively reducing localization error. Lastly, we incorporate a regularization term into the loss function to alleviate the overfitting problem on the small dataset. We evaluate our proposed methods on the Detection and Classification of Acoustic Scenes and Events (DCASE) 2022 Task 3 dataset, and the results demonstrate consistent improvements in SELD performance. In comparison to the baseline system, the proposed loss function yields significantly improved results for both localization and detection metrics on realistic data. Moreover, the proposed loss function demonstrates its ability to generalize across different network architectures, as evidenced by the consistent improvements achieved.
更多查看译文
关键词
Sound event localization and detection,loss function design,low-resource,realistic data,overfitting
AI 理解论文
溯源树
样例
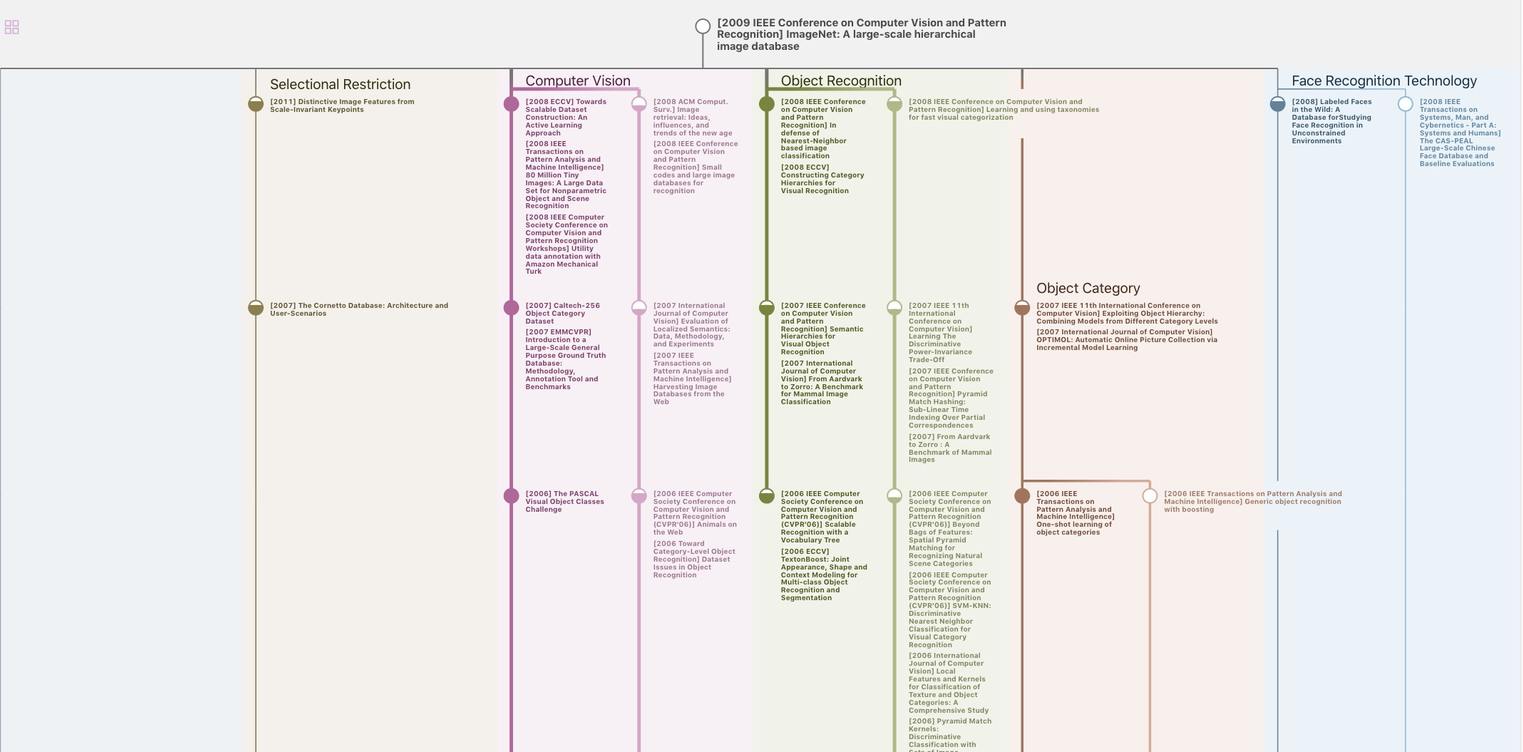
生成溯源树,研究论文发展脉络
Chat Paper
正在生成论文摘要