Deep Proximal Gradient Method for Learned Convex Regularizers
ICASSP 2023 - 2023 IEEE International Conference on Acoustics, Speech and Signal Processing (ICASSP)(2023)
摘要
We consider the problem of simultaneously learning a convex penalty function and its proximity operator for image reconstruction from incomplete measurements. Our goal is to apply Accelerated Proximal Gradient Method (APGM) using a learned proximity operator in place of the true proximity operator of the learned penalty function. Starting from a Gaussian image denoiser, we learn an associated penalty function and its proximity operator. The learned penalty function offers provable reconstruction guarantees, whereas access to its proximity operator presents the opportunity to achieve APGM convergence rates, which are faster than those of subgradient descent approaches.
更多查看译文
关键词
Deep learning,proximal gradient method,plugand-play prior,input-convex neural network
AI 理解论文
溯源树
样例
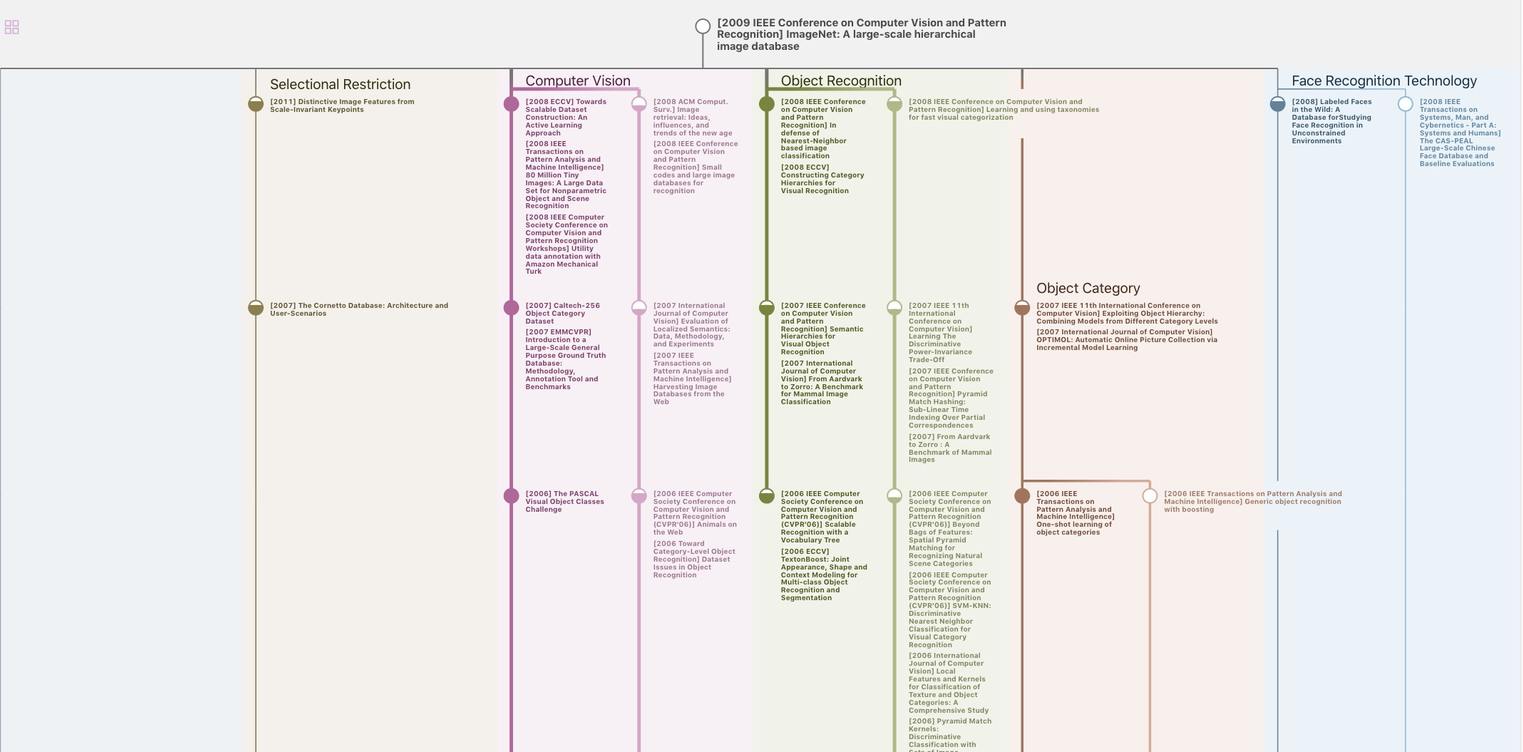
生成溯源树,研究论文发展脉络
Chat Paper
正在生成论文摘要