Enlightening the Student in Knowledge Distillation
ICASSP 2023 - 2023 IEEE International Conference on Acoustics, Speech and Signal Processing (ICASSP)(2023)
摘要
Knowledge distillation is a common method of model compression, which uses large models (teacher networks) to guide the training of small models (student networks). However, the student may find a hard time absorbing the knowledge from a sophisticated teacher due to the capacity and confidence gaps between them. To address this issue, a new knowledge distillation and refinement (KDrefine) framework is proposed to enlighten the student by expending and refining its network structure. In addition, a confidence refinement strategy is utilized to generate adaptive soften logits for efficient distillation. The experiments show that the proposed framework outperforms state-of-the-art methods on both CIFAR-100 and Tiny-ImageNet datasets. The code is available at https://github.com/YujieZheng99/KDrefine.
更多查看译文
关键词
knowledge distillation,model compression,structural re-parameterization
AI 理解论文
溯源树
样例
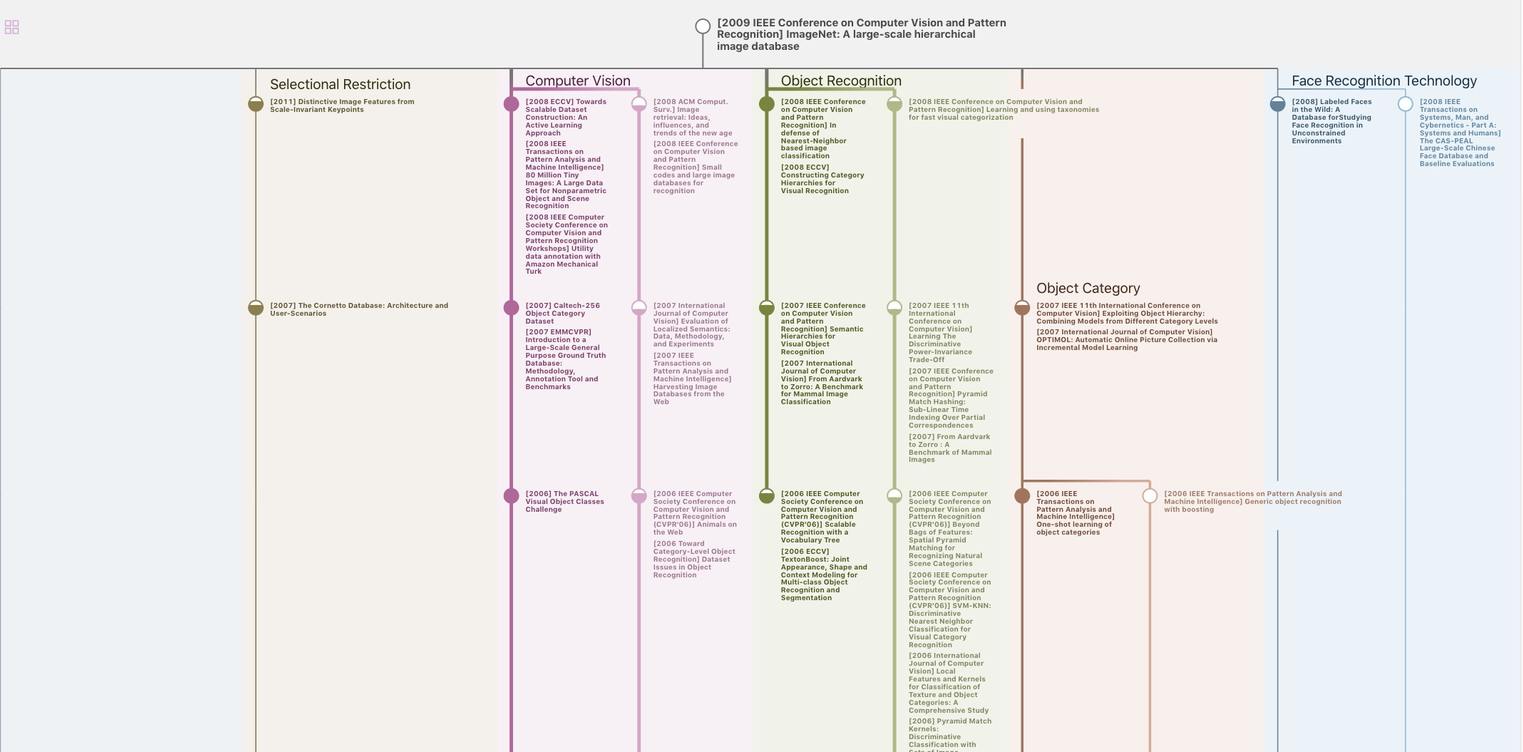
生成溯源树,研究论文发展脉络
Chat Paper
正在生成论文摘要