Feature Space Recovery for Incomplete Multi-View Clustering
ICASSP 2023 - 2023 IEEE International Conference on Acoustics, Speech and Signal Processing (ICASSP)(2023)
摘要
Incomplete multi-view clustering (IMVC), based on imputation and clustering unification, has received wide attention due to its ability to exploit hidden information from missing views. However, current methods mainly consider inter/intra-view correlations, ignoring the structural information of sample features within views. In this paper, we propose a feature space recovery based IMVC method, where low-rank feature space recovery and consensus representation learning of inter/intra-views are considered into a unified framework. Moreover, low-rank tensor ring approximation is used to capture the correlations of self-representation tensor. In an iterative way, the learned inter/intra-view correlations will guide the recovery of missing features, while the explored low-rank information from feature spaces will in turn facilitate self-representation learning, eventually achieving out-standing clustering performance. Experimental results show our method has a very significant improvement over known state-of-the-art algorithms in terms of ACC, NMI and Purity.
更多查看译文
关键词
Incomplete multi-view clustering,Low-rank tensor ring approximation,Subspace clustering,Feature space recovery,Low-rank matrix learning
AI 理解论文
溯源树
样例
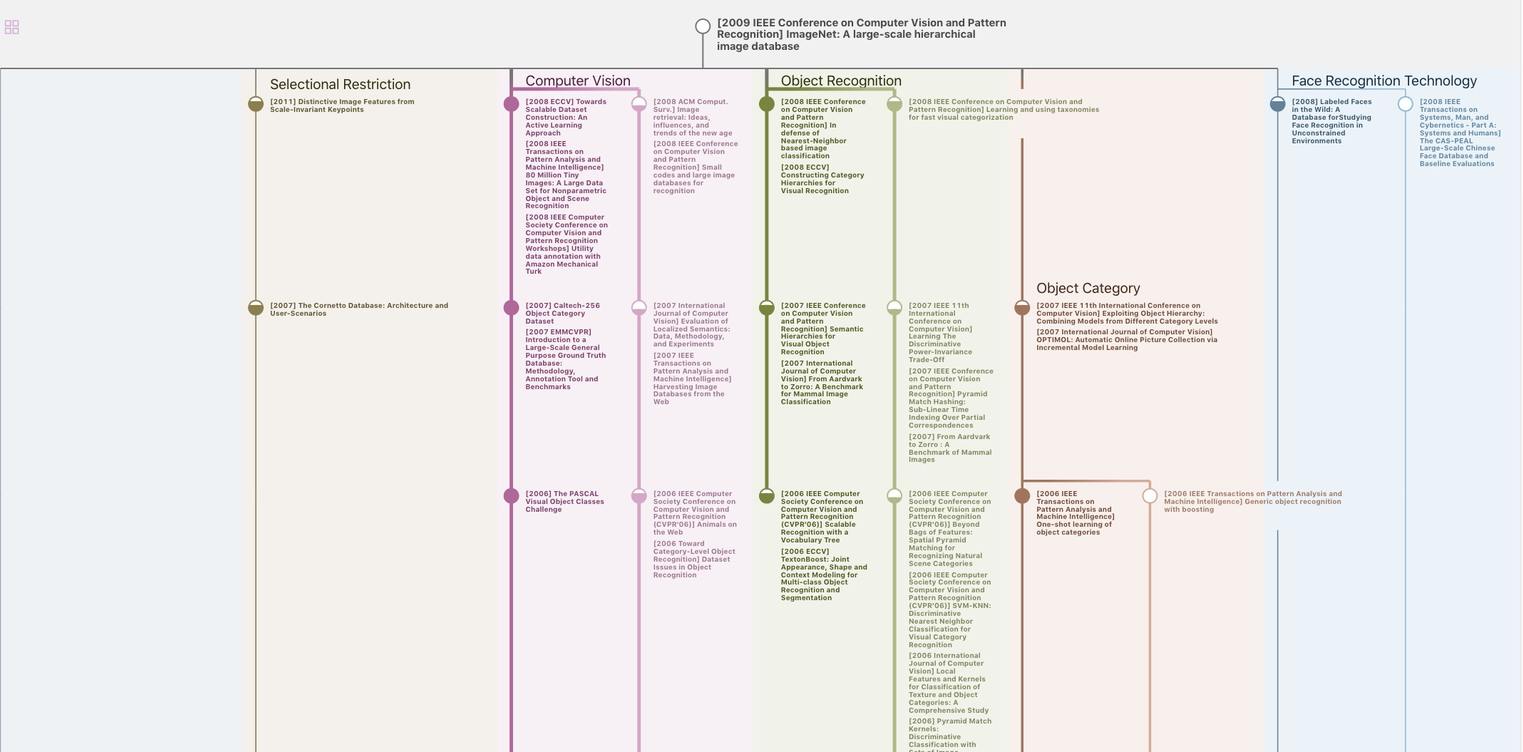
生成溯源树,研究论文发展脉络
Chat Paper
正在生成论文摘要