MSFORMER: Multi-Scale Transformer with Neighborhood Consensus for Feature Matching
ICASSP 2023 - 2023 IEEE International Conference on Acoustics, Speech and Signal Processing (ICASSP)(2023)
摘要
Existing feature matching methods tend to extract feature descriptors by feeding down-sampled feature maps into a Transformer that is unable to extend feature scales, leading to false correspondences between small-size objects. This paper proposes MSFormer, which uses Transformers situated in different branches to obtain feature descriptors. In one branch, convolutions are integrated into self-attention layers elegantly to compensate for the lack of the local structure information. In another branch, a multi-scale Transformer is proposed through injecting heterogeneous receptive field sizes into tokens. Additionally, a neighborhood consensus mechanism is proposed by re-ranking initial matches to make a constraint of geometric consensus on neighborhood feature descriptors. Extensive experiments on indoor and outdoor pose estimations show that MSFormer outperforms existing state-of-the- art methods by a large margin.
更多查看译文
关键词
Feature matching,Transformer,Neighborhood consensus,Convolutional neural network
AI 理解论文
溯源树
样例
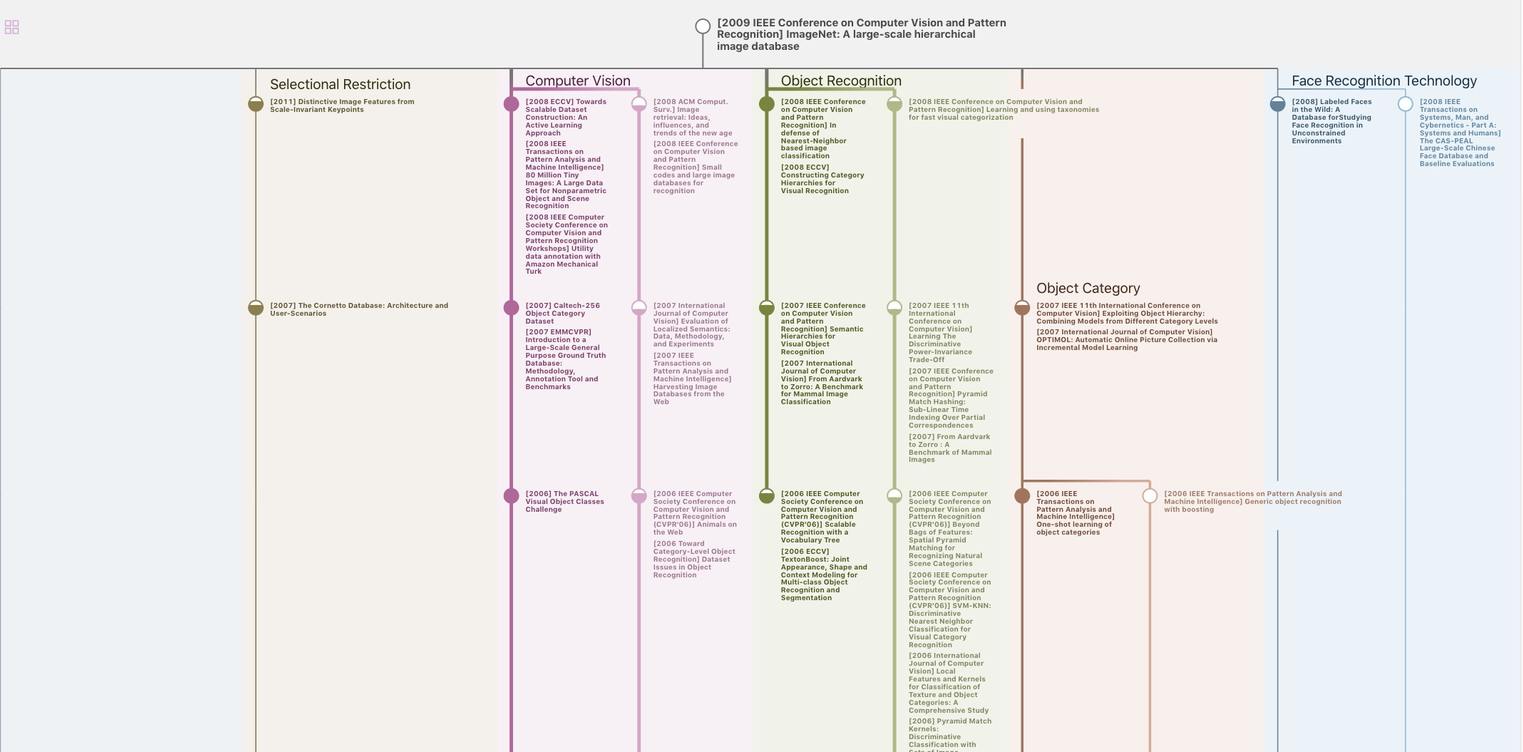
生成溯源树,研究论文发展脉络
Chat Paper
正在生成论文摘要