Cross-Domain Learning with Normalizing Flow
ICASSP 2023 - 2023 IEEE International Conference on Acoustics, Speech and Signal Processing (ICASSP)(2023)
摘要
Cross-domain learning aims to transfer knowledge learned from one or more datasets to other datasets in different domains, so that less data will be required for learning in new tasks and datasets. One big challenge in cross-domain learning is to effectively synergize the knowledge learning between domains. In this paper, we propose a new solution to address this challenge using normalizing flow, named as DomainFlow, which works as a learned mapping to establish knowledge sharing between source and target domains. The learned flow encourages the posterior distributions in multi-domain learning to be better aligned, leading to better performance in the target domain tasks. We conduct extensive experiments on three representative cross-domain learning tasks: unsupervised domain adaptation, domain generalization and zero-shot sketch-based image retrieval, which demonstrates that with DomainFlow, the overall performance on these diverse tasks can all be improved.
更多查看译文
关键词
cross-domain learning,normalizing flow,auto-encoder
AI 理解论文
溯源树
样例
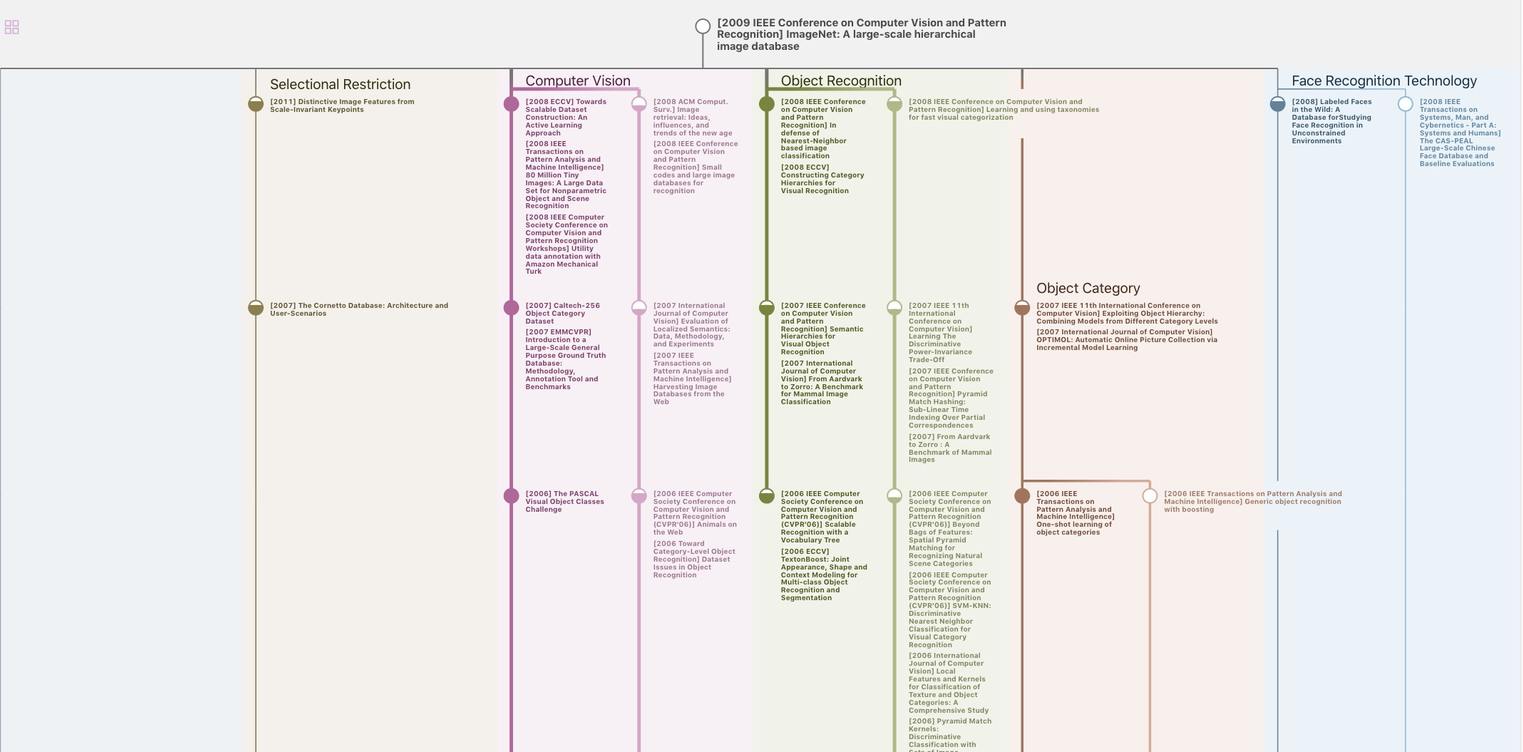
生成溯源树,研究论文发展脉络
Chat Paper
正在生成论文摘要