Contention Matters: Modeling and Analyzing the Performance of Federated Learning over WiFi
2023 IEEE 34th Annual International Symposium on Personal, Indoor and Mobile Radio Communications (PIMRC)(2023)
摘要
Federated Learning (FL) is a distributed machine learning framework coordinated by a central node. The performance of the FL is characterized by the participating nodes and their underlying communication mechanism. The state-of-the-art FL over wireless predominantly considers a contention-free communication model. The proposed work argues the need for capturing random effects of the MAC layer, as this significantly affects the performance of FL in terms of the random order of node participation, and their random times of communication. To this end, a novel mathematical model is developed to characterize the performance of FL in a WiFi network. The carrier-sensing and asynchronous random-backoff behavior of WiFi clients, and the synchronous coordination of the FL algorithm are modeled using renewal reward processes, and the performance metrics of FL are computed with the help of an underlying DTMC. Furthermore, a multicast-based MAC protocol for WiFi is also modeled and shown to perform marginally better than the unicast counterpart, thereby reaffirming the dominant effects of MAC level contention towards affecting the performance of FL. The proposed models are validated against extensive performance evaluation.
更多查看译文
关键词
Federated learning,performance modeling,and analysis,renewal reward processes,throughput analysis,WiFi
AI 理解论文
溯源树
样例
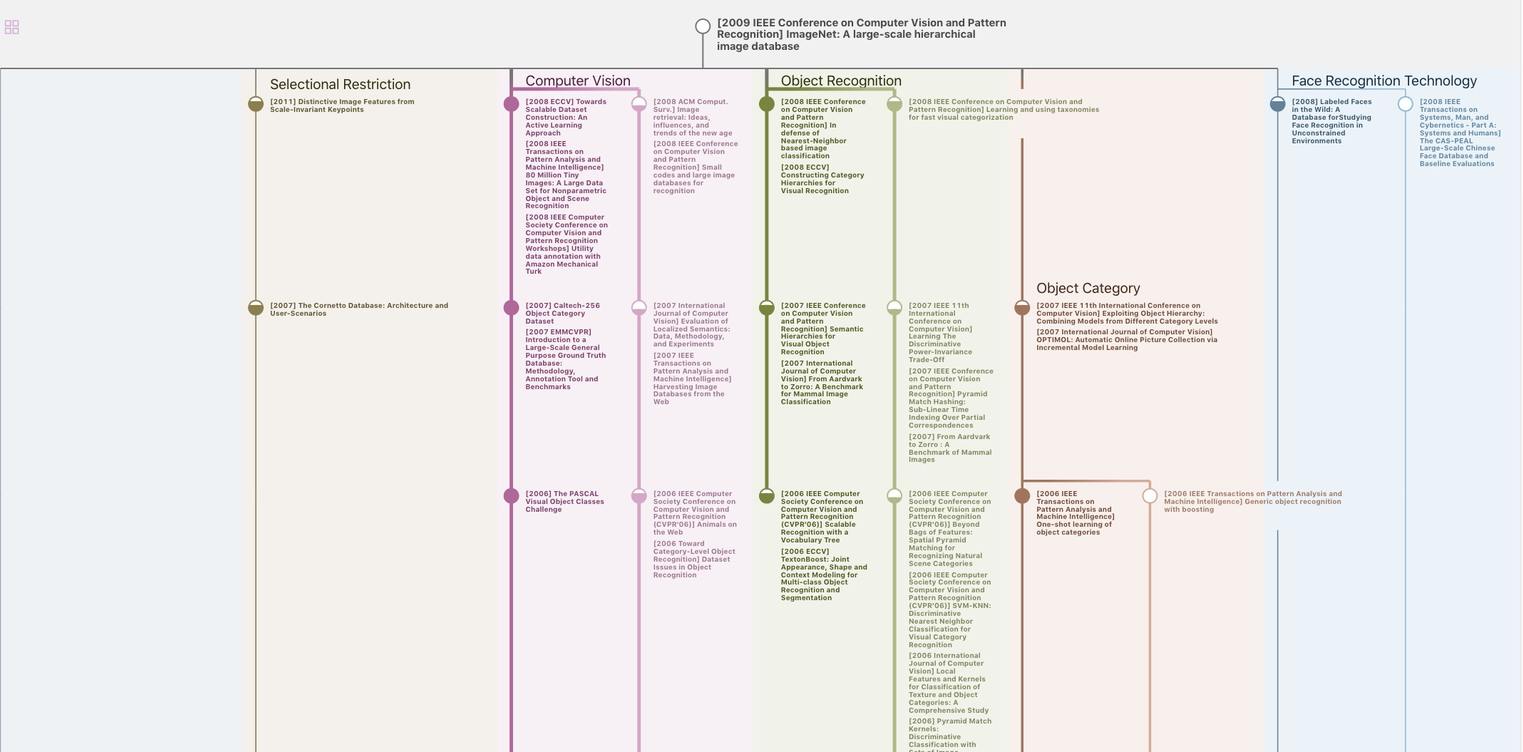
生成溯源树,研究论文发展脉络
Chat Paper
正在生成论文摘要