Multi-branch Attention Consistency Network for Facial Expression Recognition
IECON 2023- 49th Annual Conference of the IEEE Industrial Electronics Society(2023)
摘要
Due to the high inter-class similarity and subjective annotation of facial expressions, annotation uncertainty has become the key challenge in recent years. In this paper, we propose a Multi-branch Attention Consistency Network for facial expression recognition by combining latent label distribution learning and attention consistency to alleviate the annotation uncertainty. To be specific, we design three modules, namely multi-branch feature classification (MFC), multi-branch latent distribution learning (MLD) and multi-class attention consistency (MAC). The MFC classifies uncertain expressions through multiple auxiliary branches, which obtains attention maps and the degree of confidence for different facial categories. The MLD guides the target branch to learn latent label distributions from auxiliary branches. The MAC learns attention regions by multi-class attention consistency between auxiliary and target branches. Finally, we demonstrate the effectiveness of our proposed method by conducting experiments on three popular facial expression datasets. Experimental results show that our method achieves the state-of-the-art results of 90.16%, 89.98%, 63.12% accuracy on RAF-DB, FERPlus and AffectNet datasets, respectively.
更多查看译文
关键词
Facial expression recognition,Latent distribution learning,Attention consistency
AI 理解论文
溯源树
样例
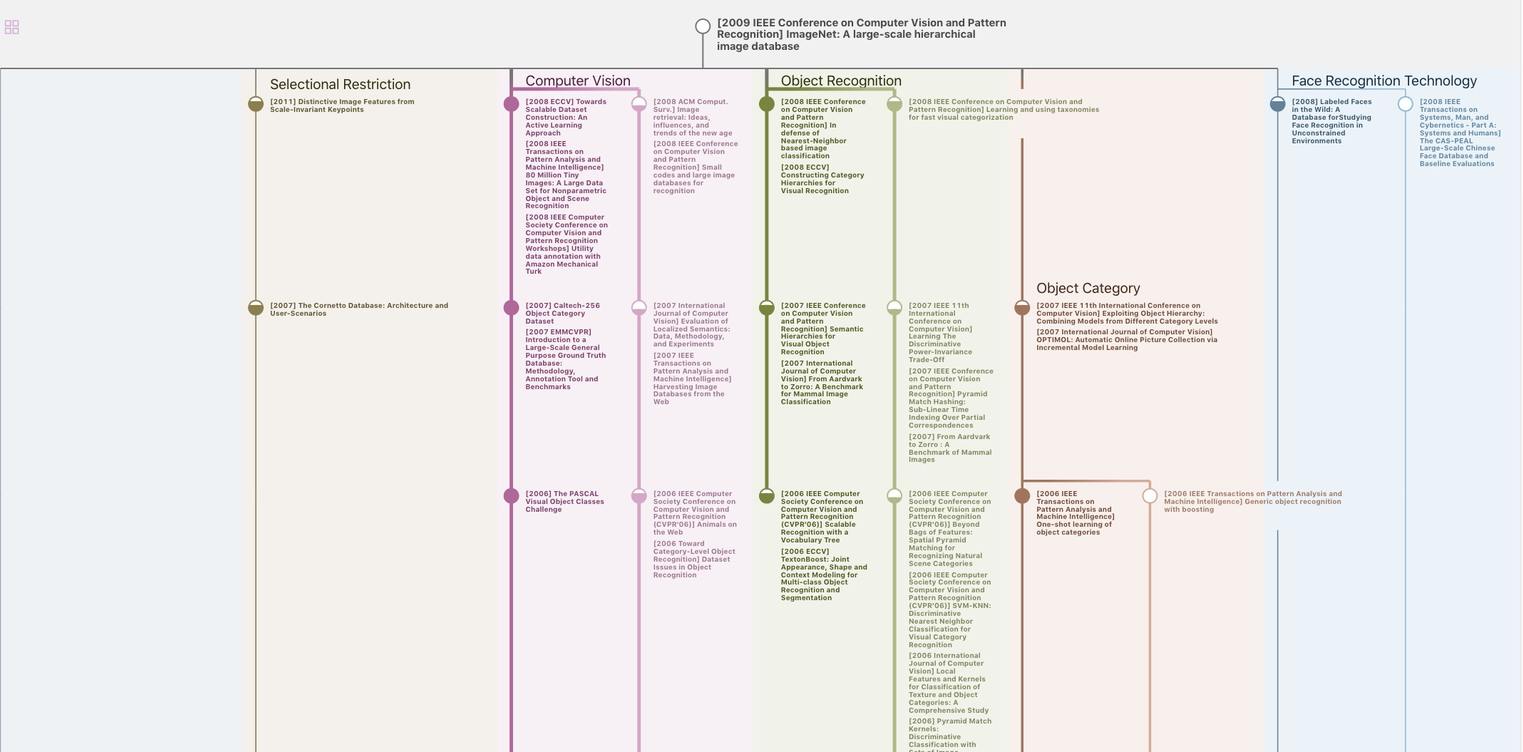
生成溯源树,研究论文发展脉络
Chat Paper
正在生成论文摘要