A Novel Long-Term Noise Prediction System Based on $\alpha$ DTW-DCRNN Using Periodically Unaligned Spatiotemporal Distribution Sequences
IEEE Systems Journal(2023)
摘要
In the era of the Internet of Things, spatial relationships are mostly constructed based on physical location information, and graph convolutional neural networks are used to solve the problem of multipoint noise prediction with the spatial distribution. To solve the problems of insufficient relation mining and poor long-term prediction accuracy in building spatial relations based on physical distance in graph convolution, the diffusion graph convolution recursive neural network (DCRNN) noise prediction system (
$ \alpha$
DTW-DCRNN) based on the
$ \alpha$
coefficient dynamic time warping (
$ \alpha$
DTW) method is proposed. First, the 3
$\sigma$
criterion is innovatively used to deal with sparse zero-value noise data, and the DTW method is proposed to mine the spatial relationship. Second, the
$\alpha$
DTW method is proposed to calculate the similarity distance of unaligned periodic sequences using the penalty coefficient. Moreover, spatial relationships are reconstructed using
$\alpha$
DTW and noise is predicted using DCRNN for long-term forecasting needs. Finally, the Kalman filter driven by traffic flow data is used to correct prediction noise to improve prediction stability. Experimental results show that the proposed system can save training time, reduce prediction errors, and make long-term prediction more accurate. Compared with the DCRNN model, the
$\alpha$
DTW-DCRNN system reduces the root-mean-square error and the mean absolute error by 11% and 5%, respectively, and reduces mean absolute percentage error by 5.26%.
更多查看译文
关键词
$\alpha$ DTW,3 $\sigma$ criterion,diffusion graph convolution recursive neural network (DCRNN),graph convolution,Kalman filter,noise prediction system
AI 理解论文
溯源树
样例
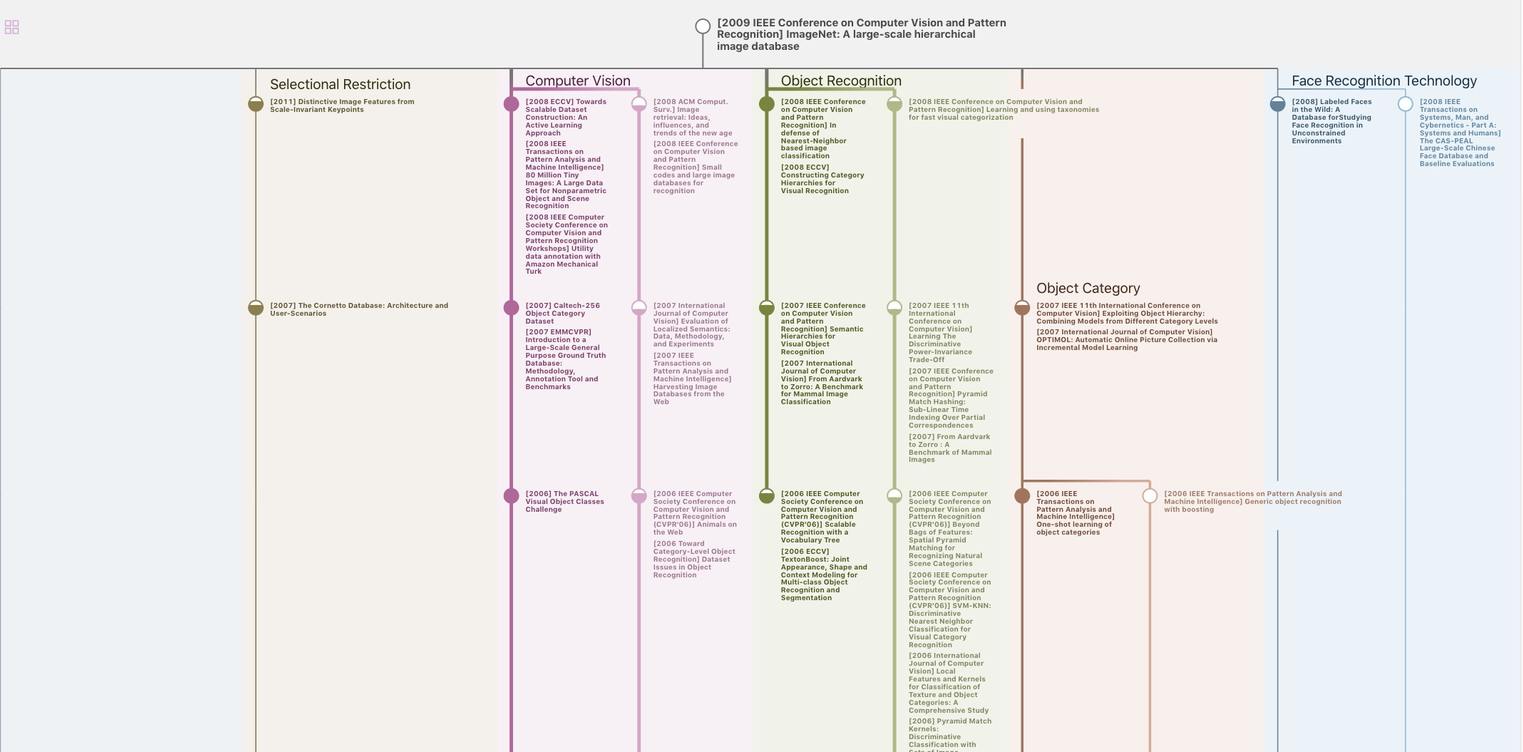
生成溯源树,研究论文发展脉络
Chat Paper
正在生成论文摘要