Self-Supervised Learning Method for SAR Multiinterference Suppression
IEEE TRANSACTIONS ON GEOSCIENCE AND REMOTE SENSING(2023)
摘要
As an active radar system, synthetic aperture radar (SAR) is often affected by different types of strong, complex, and variable electromagnetic interferences, which severely degrades the final imaging performance. Thus, how to effectively detect and suppress complex electromagnetic interferences is a crucial challenge currently. In this article, we propose a self-supervised learning interference suppression method based on deep learning, including interference localization filtering and radar signal recovery. First, we construct a novel convolutional autoencoder (CAE) deep-learning model-LocNet via the proposed optimization criterion, which is utilized to detect and locate the interference for subsequent filtration. Aiming at the issue of signal loss in the filtering process that is generally ignored in the current literature, we then reconstruct a novel U-Net neural network model-RecNet for the low-loss recovery of signal. Compared with the traditional parametric/nonparametric anti-interference methods, the most significant advantage of our method is that it overcomes the requirement for interference priori information, which is more consistent with the actual situation, and effectively solves the target information loss. Furthermore, since no interference information is involved in the training process (self-supervised training), our method applies to multiple types of interference rather than a specific one. Moreover, with our method, interference detection and suppression can be achieved simultaneously instead of separating the two steps as in existing literature. Measured and simulated SAR interference-contaminated (IF-contaminated) data test results validate the effectiveness and robustness of the proposed method.
更多查看译文
关键词
Interference,Time-frequency analysis,Synthetic aperture radar,Radar,Radar imaging,Interference suppression,Filtering,Deep learning,interference suppression,signal recovery,synthetic aperture radar (SAR)
AI 理解论文
溯源树
样例
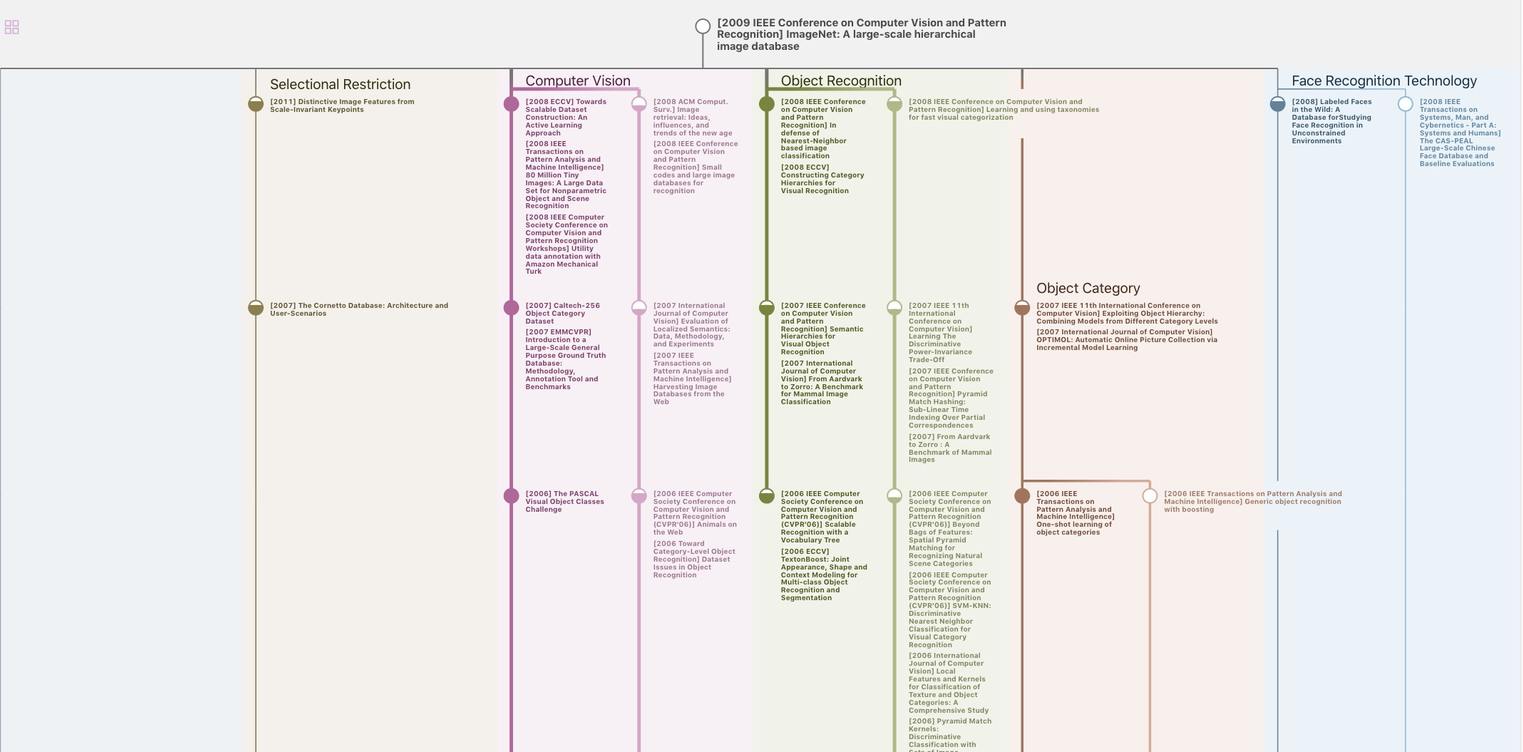
生成溯源树,研究论文发展脉络
Chat Paper
正在生成论文摘要