M2FNet: Multimodal Fusion Network for Airport Runway Subsurface Defect Detection Using GPR Data
IEEE Transactions on Geoscience and Remote Sensing(2023)
摘要
Ground-penetrating radar (GPR) is widely used for detecting airport runway subsurface defects. The trailing interference in the GPR data disguises subsurface defect responses, which seriously affects the accuracy of subsurface defect detection. To tackle the challenge of subsurface defects detection under trailing interference in real scenarios, a new multimodal fusion network referred to as M(2)FNet is proposed. Based on the premise that the trailing signal is highly similar across all adjacent A-scans, the model employs a transformer encoder to extract global features of the signal with long-distance correlation. In contrast, the subsurface defects only show echo characteristics in a few adjacent B-scans. The phase of the trailing and the target signal is opposite, which is easy to discover from the Top-scan view. Thus, a hybrid convolutional neural network structure is used to extract local features from GPR images of different views. This dual network structure extremely enhances the representation learning of GPR data. In order to investigate various subsurface defects and trailing interference collected by multiple GPR systems under different conditions, the first large-scale hybrid dataset called ASD-GPR is created. Transfer learning is employed to enhance the model's ability to detect rare defects by fine-tuning it for real-world situations, differing from synthetic training data scenarios. The results of the experiments reveal that M(2)FNet outperforms state-of-the-art object detection methods in various real-world scenarios, demonstrating superior performance in detecting subsurface defects.
更多查看译文
关键词
Airport runway inspection,ground-penetrating radar (GPR),multimodal fusion network (M²FNet),subsurface defect detection,trailing interference
AI 理解论文
溯源树
样例
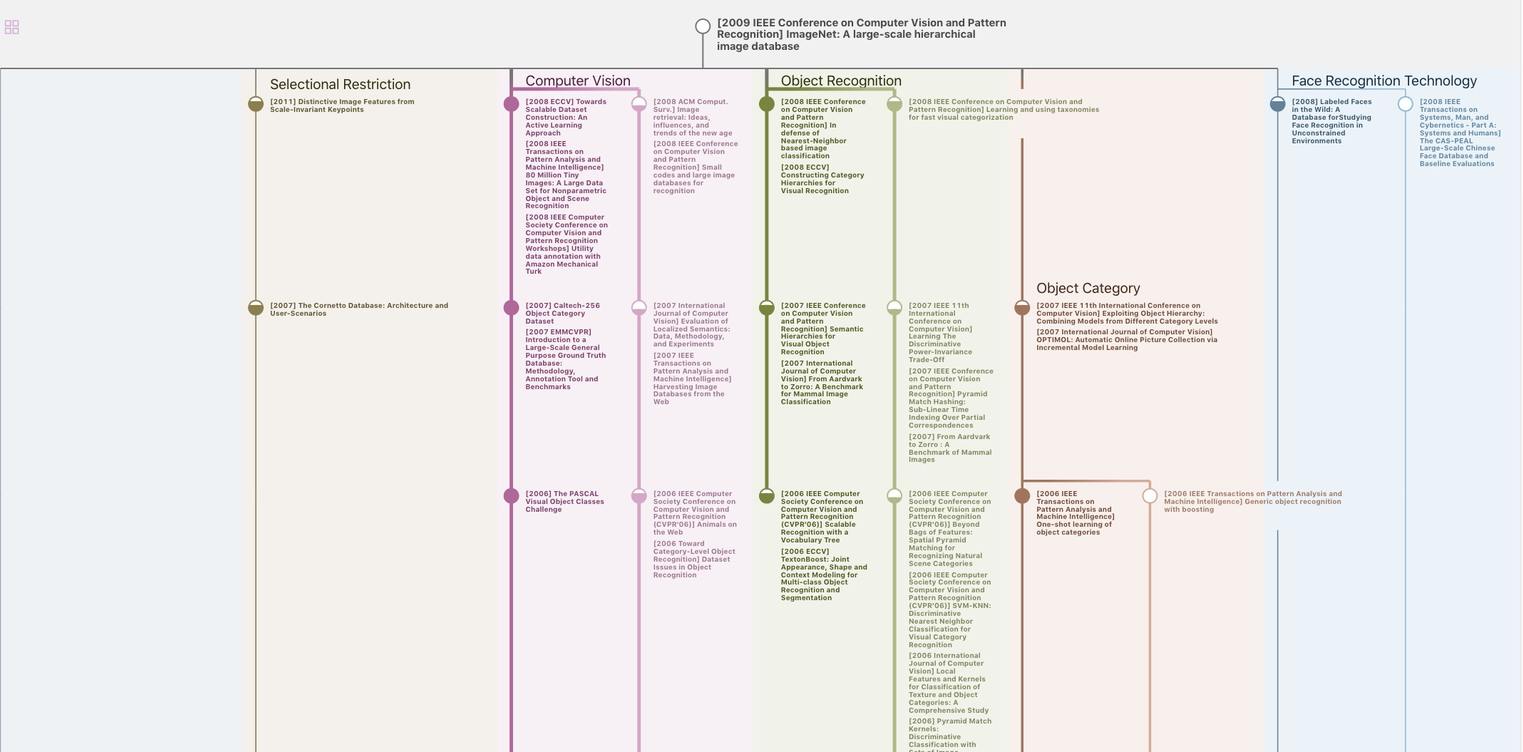
生成溯源树,研究论文发展脉络
Chat Paper
正在生成论文摘要