A Data-Related Patch Proposal for Semantic Segmentation of Aerial Images
IEEE GEOSCIENCE AND REMOTE SENSING LETTERS(2023)
摘要
Large-size images cannot be directly put into GPU for training and need to be cropped to patches due to GPU memory limitation. The commonly used cropping methods before are random cropping and sequential cropping, which are crude and fatally inefficient. First, categories of datasets are often imbalanced, and just simple cropping misses an excellent opportunity to make the data distribution balanced. Second, the training needs to crop a large number of patches to cover all patterns, which greatly increases the training time. This problem is of great practical hazards but is often overlooked by previous works. The optimal solution is to generate valuable patches. Valuable patches refer to the value to network training, i.e., the value of this patch for the convergence of the network, and the improvement of the accuracy. To this end, we propose a data-related patch proposal strategy to sample high valuable patches. The core idea is to score each patch according to the accuracy of each category, so as to perform balanced sampling. Compared with random cropping or sequential cropping, our method can improve the segmentation accuracy and accelerate the training vastly. Moreover, our method also shows great advantages over the loss-based balanced approaches. Experiments on Deepglobe and Potsdam show the excellent effect of our method.
更多查看译文
关键词
Training,Proposals,Crops,Graphics processing units,Electronic mail,Data mining,Sampling methods,Large-size images,patch proposal,semantic segmentation
AI 理解论文
溯源树
样例
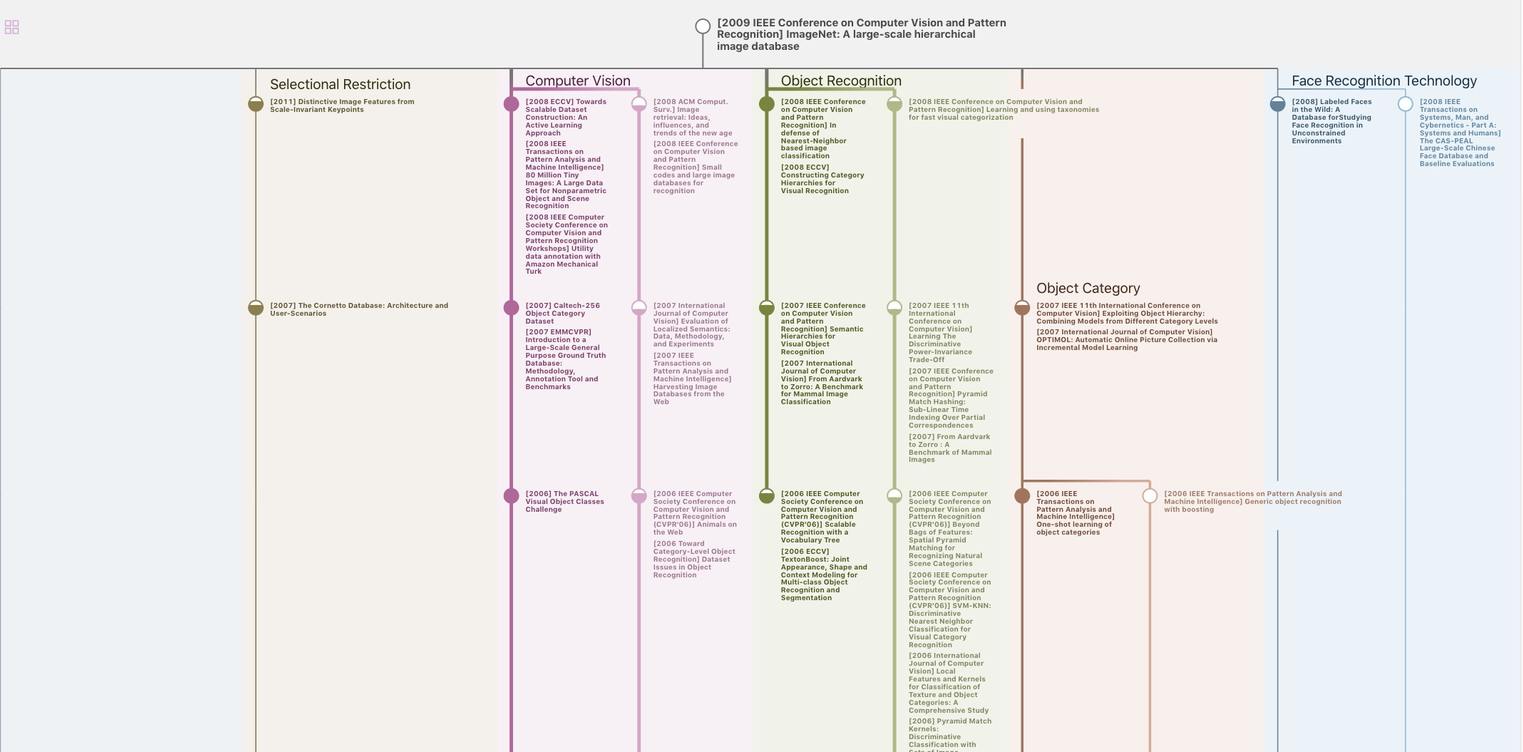
生成溯源树,研究论文发展脉络
Chat Paper
正在生成论文摘要