Reinforcement Learning-Based Optimal Self-Learning Tracking Control for Two-Time Scale System
2023 5th International Conference on Industrial Artificial Intelligence (IAI)(2023)
摘要
This paper devotes to solving the optimal tracking control (OTC) problem of singular perturbation systems in industrial processes under the framework of reinforcement learning technology. The encountered challenges include the different time scales in system operations and an unknown slow process. The immeasurabilty of slow process states especially increases the difficulty of finding the optimal tracking controller. To overcome these challenges, a novel off-policy ridge RL method is developed after decomposing the singular perturbed systems using the singular perturbation (SP) theory and replacing unmeasured states using important mathematical manipulations. Theoretical analysis of approximate equivalence of the sum of solutions of subproblems to the solution of the OTC problem is presented. Finally, a mixed separation thickening process (MSTP) and a numerical example are used to verify the effectiveness.
更多查看译文
关键词
Optimal tracking control,reinforcement learning,singular perturbation theory,off-policy learning
AI 理解论文
溯源树
样例
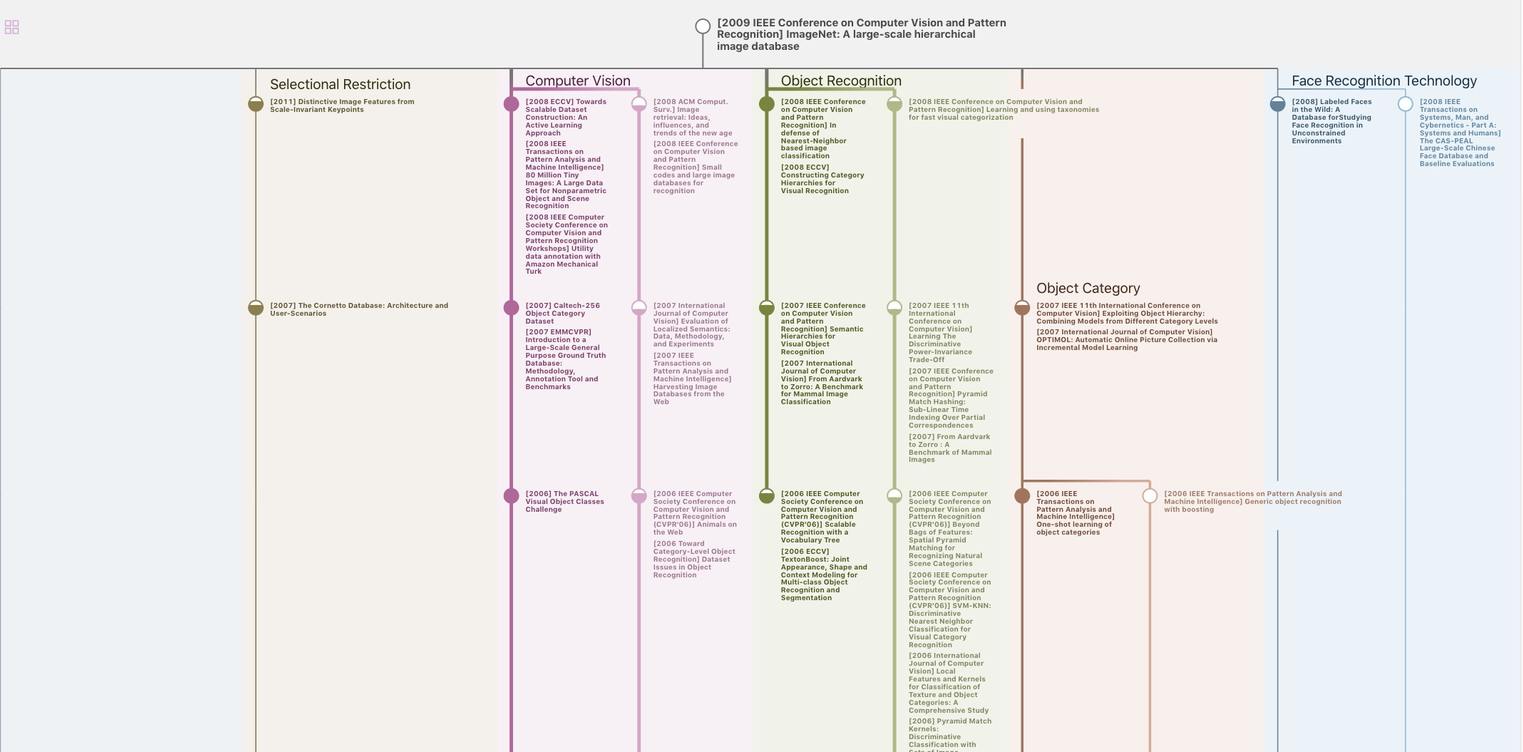
生成溯源树,研究论文发展脉络
Chat Paper
正在生成论文摘要