A Review of Stability in Topic Modeling: Metrics for Assessing and Techniques for Improving Stability.
ACM Comput. Surv.(2024)
摘要
Topic modeling includes a variety of machine learning techniques for identifying latent themes in a corpus of documents. Generating an exact solution (i.e., finding global optimum) is often computationally intractable. Various optimization techniques (e.g., Variational Bayes or Gibbs Sampling) are employed to generate topic solutions approximately by finding local optima. Such an approximation often begins with a random initialization, which leads to different results with different initializations. The term “stability” refers to a topic model’s ability to produce solutions that are partially or completely identical across multiple runs with different random initializations. Although a variety of work has been done analyzing, measuring, or improving stability, no single paper has provided a thorough review of different stability metrics nor of various techniques that improved the stability of a topic model. This paper fills that gap and provides a systematic review of different approaches to measure stability and of various techniques that are intended to improve stability. It also describes differences and similarities between stability measures and other metrics (e.g., generality, coherence). Finally, the paper discusses the importance of analyzing both stability and quality metrics to assess and to compare topic models.
更多查看译文
关键词
topic modeling,stability
AI 理解论文
溯源树
样例
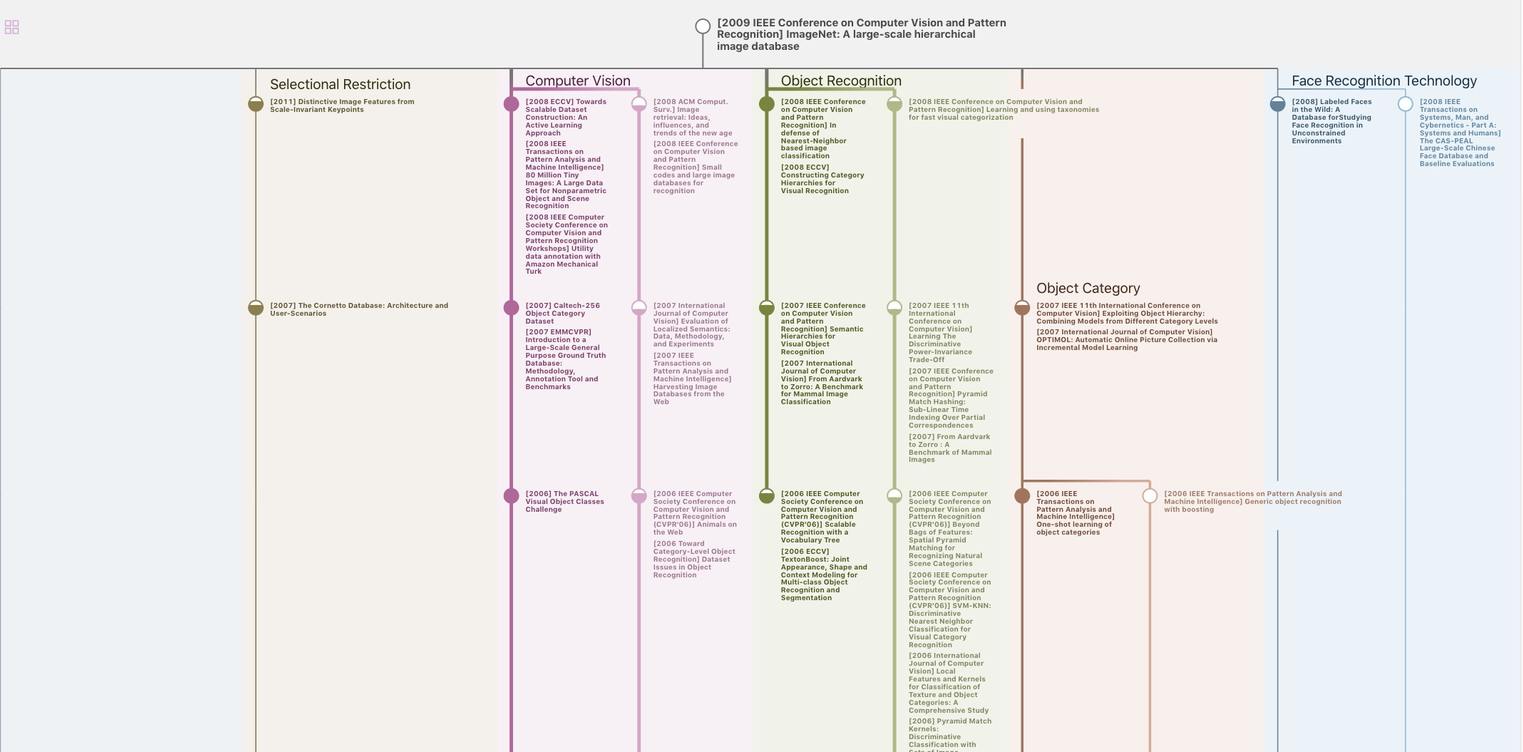
生成溯源树,研究论文发展脉络
Chat Paper
正在生成论文摘要