Protein domain embeddings for fast and accurate similarity search
biorxiv(2024)
摘要
Recently developed protein language models have enabled a variety of applications of the protein contextual embeddings. Per-protein representations (each protein is represented as a vector of fixed dimension) can be derived via averaging the embeddings of individual residues, or applying matrix transformation techniques such as the discrete cosine transformation to matrices of residue embeddings. Such protein-level embeddings have been applied to enable fast searches of similar proteins, however limitations have been found; for example, PROST is good at detecting global homologs but not local homologs, and knnProtT5 excels for proteins of single domains but not multi-domain proteins. Here we propose a novel approach that first segments proteins into domains (or subdomains) and then applies discrete cosine transformation to the vectorized embeddings of residues in each domain to infer domain-level contextual vectors. Our approach called DCTdomain utilizes predicted contact maps from ESM-2 for domain segmentation, which is formulated as a domain segmentation problem and can be solved using a recursive cut algorithm (RecCut in short) in quadratic time to the protein length; for comparison, an existing approach for domain segmentation uses a cubic-time algorithm. We showed such domain-level contextual vectors (referred as DCT fingerprints) enable fast and accurate detection of similarity between proteins that share global similarities but with undefined extended regions between shared domains, and those that only share local similarities.
### Competing Interest Statement
The authors have declared no competing interest.
更多查看译文
AI 理解论文
溯源树
样例
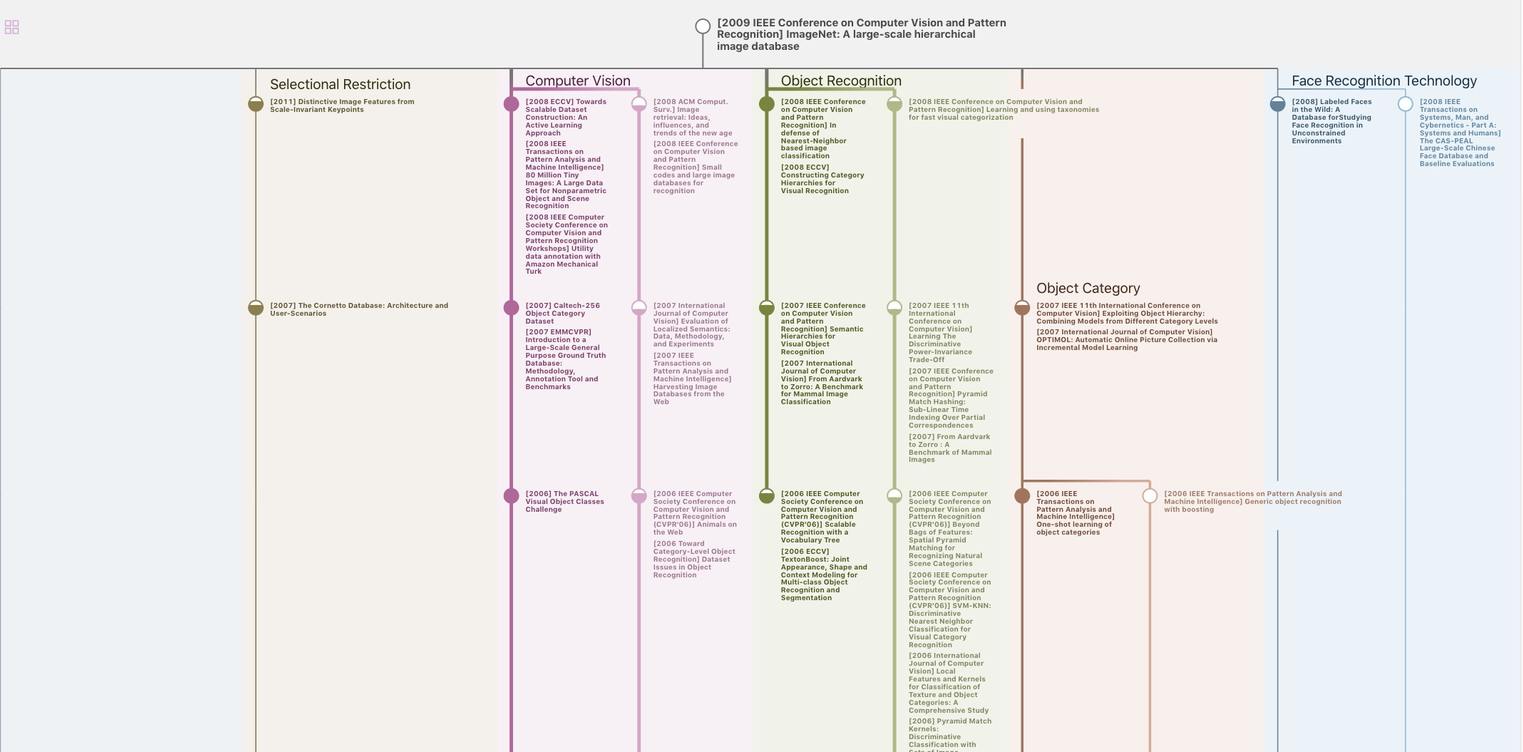
生成溯源树,研究论文发展脉络
Chat Paper
正在生成论文摘要