UFDA: Universal Federated Domain Adaptation with Practical Assumptions
AAAI 2024(2024)
摘要
Conventional Federated Domain Adaptation (FDA) approaches usually demand an abundance of assumptions, which makes them significantly less feasible for real-world situations and introduces security hazards. This paper relaxes the assumptions from previous FDAs and studies a more practical scenario named Universal Federated Domain Adaptation (UFDA). It only requires the black-box model and the label set information of each source domain, while the label sets of different source domains could be inconsistent, and the target-domain label set is totally blind. Towards a more effective solution for our newly proposed UFDA scenario, we propose a corresponding methodology called Hot-Learning with Contrastive Label Disambiguation (HCLD). It particularly tackles UFDA's domain shifts and category gaps problems by using one-hot outputs from the black-box models of various source domains. Moreover, to better distinguish the shared and unknown classes, we further present a cluster-level strategy named Mutual-Voting Decision (MVD) to extract robust consensus knowledge across peer classes from both source and target domains. Extensive experiments on three benchmark datasets demonstrate that our method achieves comparable performance for our UFDA scenario with much fewer assumptions, compared to previous methodologies with comprehensive additional assumptions.
更多查看译文
关键词
ML: Transfer, Domain Adaptation, Multi-Task Learning,ML: Privacy,ML: Unsupervised & Self-Supervised Learning,PEAI: Privacy & Security
AI 理解论文
溯源树
样例
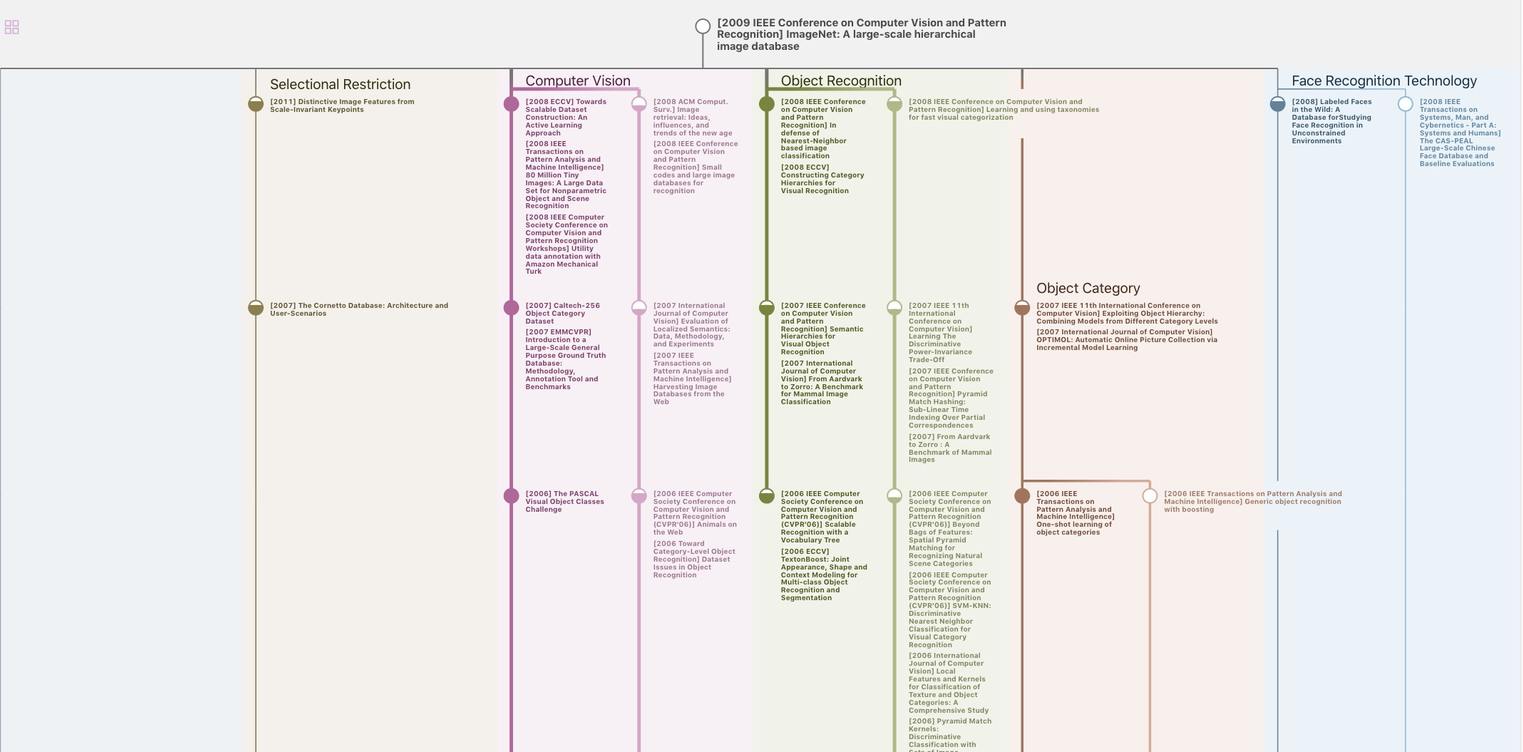
生成溯源树,研究论文发展脉络
Chat Paper
正在生成论文摘要