Can SAM recognize crops? Quantifying the zero-shot performance of a semantic segmentation foundation model on generating crop-type maps using satellite imagery for precision agriculture.
CoRR(2023)
摘要
Climate change is increasingly disrupting worldwide agriculture, making global food production less reliable.To tackle the growing challenges in feeding the planet, cutting-edge management strategies, such as precision agriculture, empower farmers and decision-makers with rich and actionable information to increase the efficiency and sustainability of their farming practices.Crop-type maps are key information for decision-support tools but are challenging and costly to generate.We investigate the capabilities of Meta AI's Segment Anything Model (SAM) for crop-map prediction task, acknowledging its recent successes at zero-shot image segmentation.However, SAM being limited to up-to 3 channel inputs and its zero-shot usage being class-agnostic in nature pose unique challenges in using it directly for crop-type mapping.We propose using clustering consensus metrics to assess SAM's zero-shot performance in segmenting satellite imagery and producing crop-type maps.Although direct crop-type mapping is challenging using SAM in zero-shot setting, experiments reveal SAM's potential for swiftly and accurately outlining fields in satellite images, serving as a foundation for subsequent crop classification.This paper attempts to highlight a use-case of state-of-the-art image segmentation models like SAM for crop-type mapping and related specific needs of the agriculture industry, offering a potential avenue for automatic, efficient, and cost-effective data products for precision agriculture practices.
更多查看译文
AI 理解论文
溯源树
样例
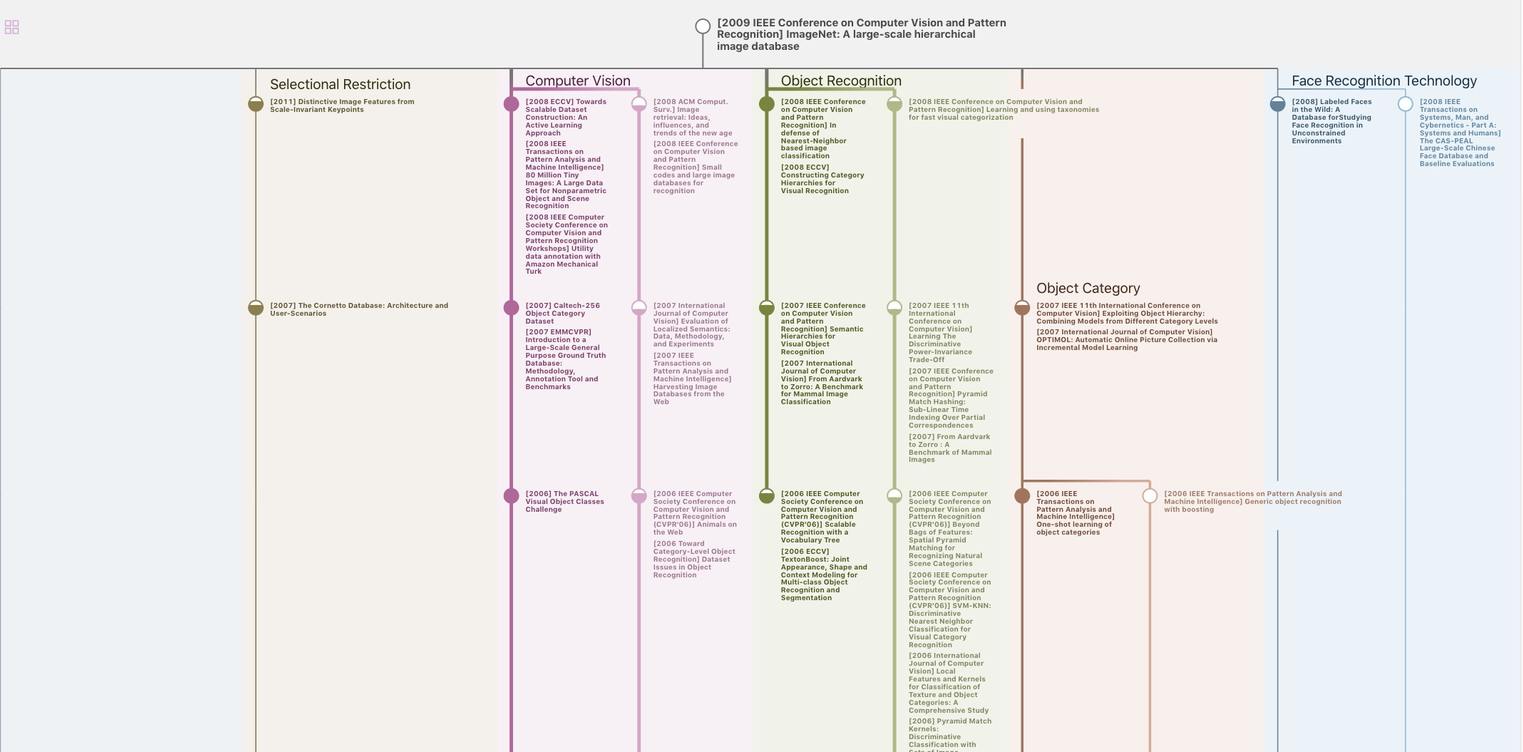
生成溯源树,研究论文发展脉络
Chat Paper
正在生成论文摘要