A deep generative model for estimating single-cell RNA splicing and degradation rates
biorxiv(2023)
摘要
Messenger RNA splicing and degradation kinetics contribute to gene expression regulation, abnormality of which is closely associated with the development of various diseases. The RNA velocity theory achieves optimization of the kinetic rates by applying splicing mathematical models to single-cell transcriptome data; however, most previous methods assumed a single value for all cells. Here, we introduce DeepKINET, a novel method for estimating splicing and degradation rates at single-cell resolution, using a deep generative model framework. DeepKINET assumes a single-cell transcriptome generation process based on a mathematical splicing model and latent cellular state-dependent kinetic rates. Using these assumptions and optimizing real scRNA-seq data, DeepKINET achieved a single-cell kinetic rate estimation. We first validated the accuracy of the estimated rates using a simulated dataset and a metabolic labeling dataset and confirmed the superiority of our method over an existing method. We further applied DeepKINET to forebrain and breast cancer datasets to extract RNA-binding proteins responsible for the diversity of splicing and degradation rates and to identify genes showing significant changes in kinetic rates across cell populations. Our results indicated that DeepKINET is an effective method for revealing cellular heterogeneity in post-transcriptional regulation and the molecular mechanisms that produce heterogeneity.
### Competing Interest Statement
The authors have declared no competing interest.
更多查看译文
AI 理解论文
溯源树
样例
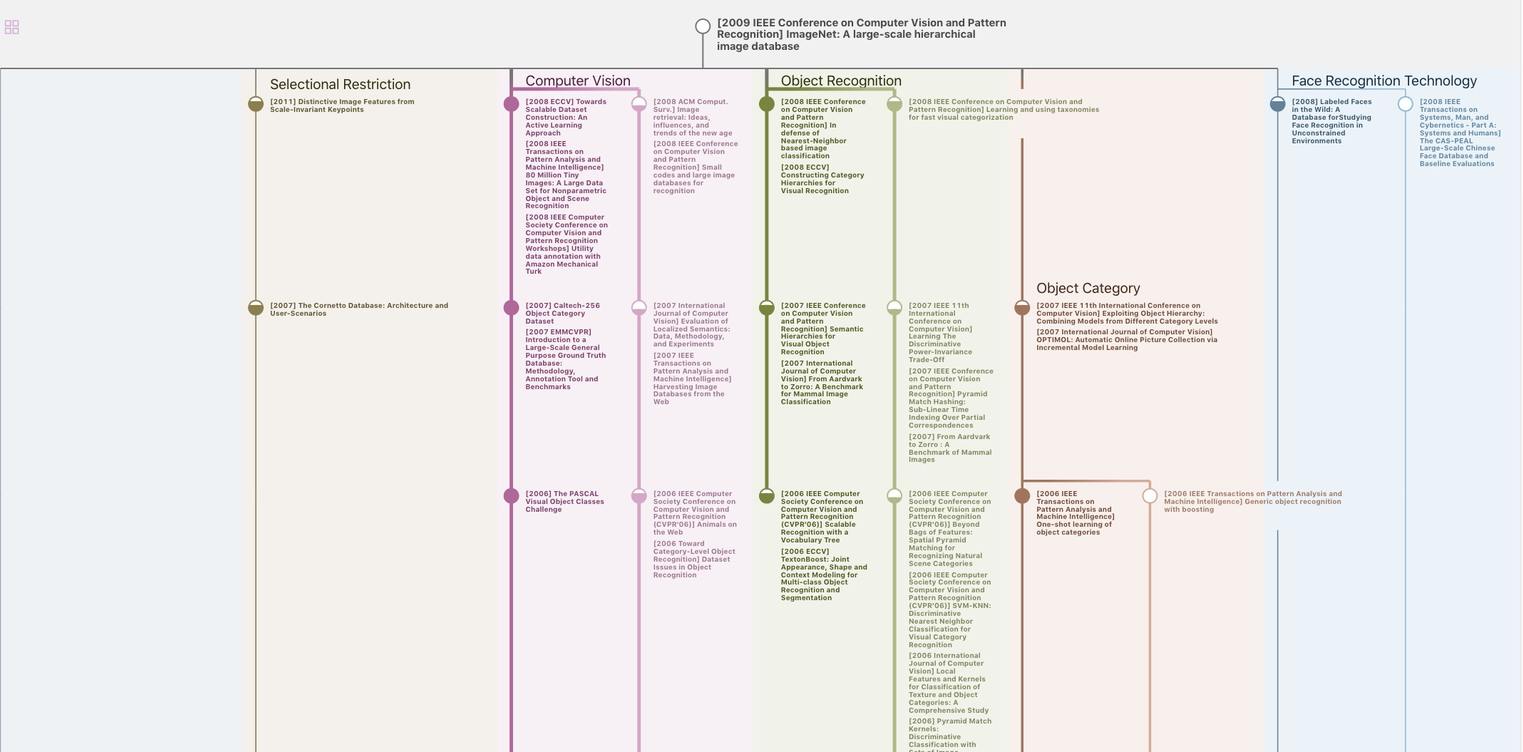
生成溯源树,研究论文发展脉络
Chat Paper
正在生成论文摘要