On the Hyperparameter Landscapes of Machine Learning Algorithms.
CoRR(2023)
摘要
Despite the recent success in a plethora of hyperparameter optimization (HPO)
methods for machine learning (ML) models, the intricate interplay between model
hyperparameters (HPs) and predictive losses (a.k.a fitness), which is a key
prerequisite for understanding HPO, remain notably underexplored in our
community. This results in limited explainability in the HPO process, rendering
a lack of human trust and difficulties in pinpointing algorithm bottlenecks. In
this paper, we aim to shed light on this black box by conducting large-scale
fitness landscape analysis (FLA) on 1,500 HP loss landscapes of 6 ML models
with more than 11 model configurations, across 67 datasets and different levels
of fidelities. We reveal the first unified, comprehensive portrait of their
topographies in terms of smoothness, neutrality and modality. We also show that
such properties are highly transferable across datasets and fidelities,
providing fundamental evidence for the success of multi-fidelity and transfer
learning methods. These findings are made possible by developing a dedicated
FLA framework that incorporates a combination of visual and quantitative
measures. We further demonstrate the potential of this framework by analyzing
the NAS-Bench-101 landscape, and we believe it is able to faciliate fundamental
understanding of a broader range of AutoML tasks.
更多查看译文
AI 理解论文
溯源树
样例
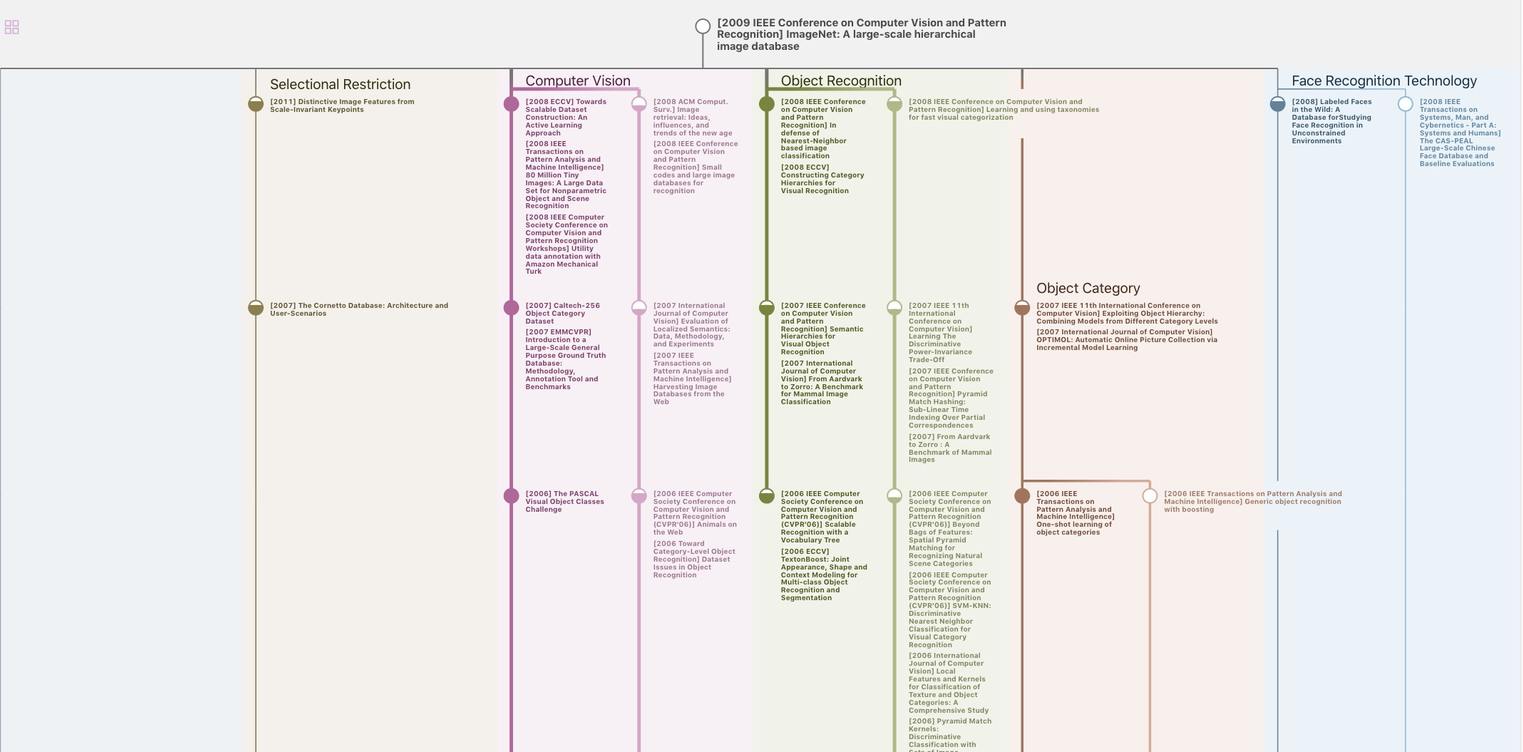
生成溯源树,研究论文发展脉络
Chat Paper
正在生成论文摘要