End-to-end Transfer Learning for Speaker-independent Cross-language Speech Emotion Recognition
arxiv(2023)
摘要
Data-driven models achieve successful results in Speech Emotion Recognition
(SER). However, these models, which are based on general acoustic features or
end-to-end approaches, show poor performance when the testing set has a
different language (i.e., the cross-language setting) than the training set or
when they come from a different dataset (i.e., the cross-corpus setting). To
alleviate this problem, this paper presents an end-to-end Deep Neural Network
(DNN) model based on transfer learning for cross-language SER. We use the
wav2vec 2.0 pre-trained model to transform audio time-domain waveforms from
different languages, different speakers and different recording conditions into
a feature space shared by multiple languages, thereby it reduces the language
variabilities in the speech features. Next, we propose a new Deep-Within-Class
Co-variance Normalisation (Deep-WCCN) layer that can be inserted into the DNN
model and it aims to reduce other variabilities including speaker variability,
channel variability and so on. The whole model is fine-tuned in an end-to-end
manner on a combined loss and is validated on datasets from three languages
(i.e., English, German, Chinese). Experiment results show that our proposed
method not only outperforms the baseline model that is based on common acoustic
feature sets for SER in the within-language setting, but also significantly
outperforms the baseline model for cross-language setting. In addition, we also
experimentally validate the effectiveness of Deep-WCCN, which can further
improve the model performance. Finally, to comparing the results in the recent
literatures that use the same testing datasets, our proposed model shows
significantly better performance than other state-of-the-art models in
cross-language SER.
更多查看译文
AI 理解论文
溯源树
样例
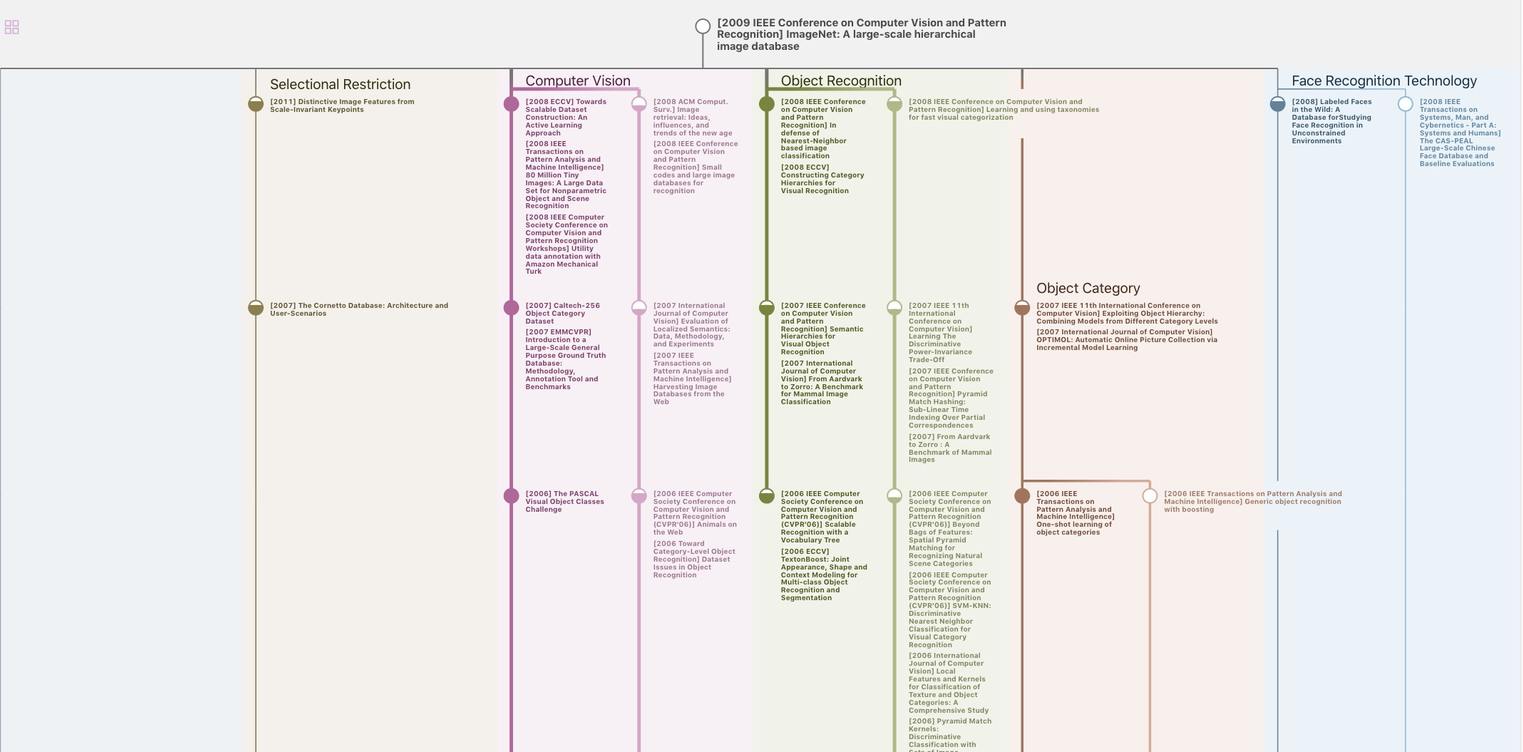
生成溯源树,研究论文发展脉络
Chat Paper
正在生成论文摘要