A three-dimensional deep learning model for inter-site harmonization of structural MR images of the brain: Extensive validation with a multicenter dataset
HELIYON(2023)
摘要
In multicenter MRI studies, pooling the imaging data can introduce site-related variabilities and can therefore bias the subsequent analyses. To harmonize the intensity distributions of brain MR images in a multicenter dataset, unsupervised deep learning methods can be employed. Here, we developed a model based on cycle-consistent adversarial networks for the harmonization of T1-weighted brain MR images. In contrast to previous works, it was designed to process threedimensional whole-brain images in a stable manner while optimizing computation resources. Using six different MRI datasets for healthy adults (n=1525 in total) with different acquisition parameters, we tested the model in (i) three pairwise harmonizations with site effects of various sizes, (ii) an overall harmonization of the six datasets with different age distributions, and (iii) a traveling-subject dataset. Our results for intensity distributions, brain volumes, image quality metrics and radiomic features indicated that the MRI characteristics at the various sites had been effectively homogenized. Next, brain age prediction experiments and the observed correlation between the gray-matter volume and age showed that thanks to an appropriate training strategy and despite biological differences between the dataset populations, the model reinforced biological patterns. Furthermore, radiologic analyses of the harmonized images attested to the conservation of the radiologic information in the original images. The robustness of the harmonization model (as judged with various datasets and metrics) demonstrates its potential for application in retrospective multicenter studies.
更多查看译文
关键词
Brain MRI,Harmonization,CycleGAN,Brain volumetry,Radiomics,Brain age
AI 理解论文
溯源树
样例
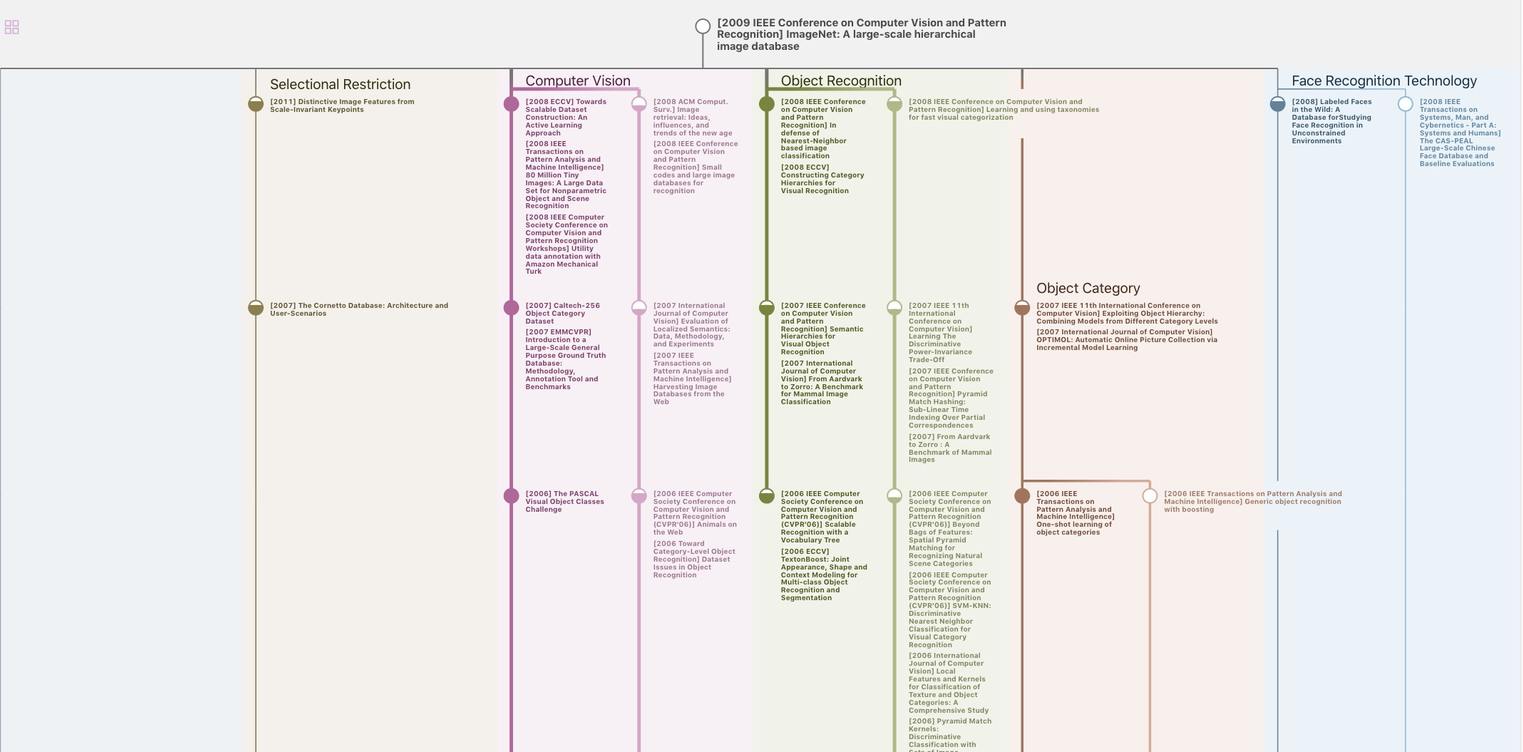
生成溯源树,研究论文发展脉络
Chat Paper
正在生成论文摘要