Inter-modal Fusion Network with Graph Structure Preserving for Fake News Detection
NEURAL INFORMATION PROCESSING, ICONIP 2023, PT VI(2024)
摘要
The continued ferment of fake news on the network threatens the stability and security of society, prompting researchers to focus on fake news detection. The development of social media has made it challenging to detect fake news by only using uni-modal information. Existing studies tend to integrate multi-modal information to pursue completeness for information mining. How to eliminate modality differences effectively while capturing structure information well from multimodal data remains a challenging issue. To solve this problem, we propose an Inter-modal Fusion network with Graph Structure Preserving (IF-GSP) approach for fake news detection. An inter-modal cross-layer fusion module is designed to bridge the modality differences by integrating features in different layers between modalities. Intra-modal and cross-modal contrastive losses are designed to enhance the inter-modal semantic similarity while focusing on modal-specific discriminative representation learning. A graph structure preserving module is designed to make the learned features fully perceive the graph structure information based on a graph convolutional network (GCN). A multi-modal fusion module utilizes an attention mechanism to adaptively integrate cross-modal feature representations. Experiments on two widely used datasets show that IF-GSP outperforms related multi-modal fake news detection methods.
更多查看译文
关键词
Contrastive Learning,Fake News Detection,Graph Convolutional Network,Multi-modal Fusion
AI 理解论文
溯源树
样例
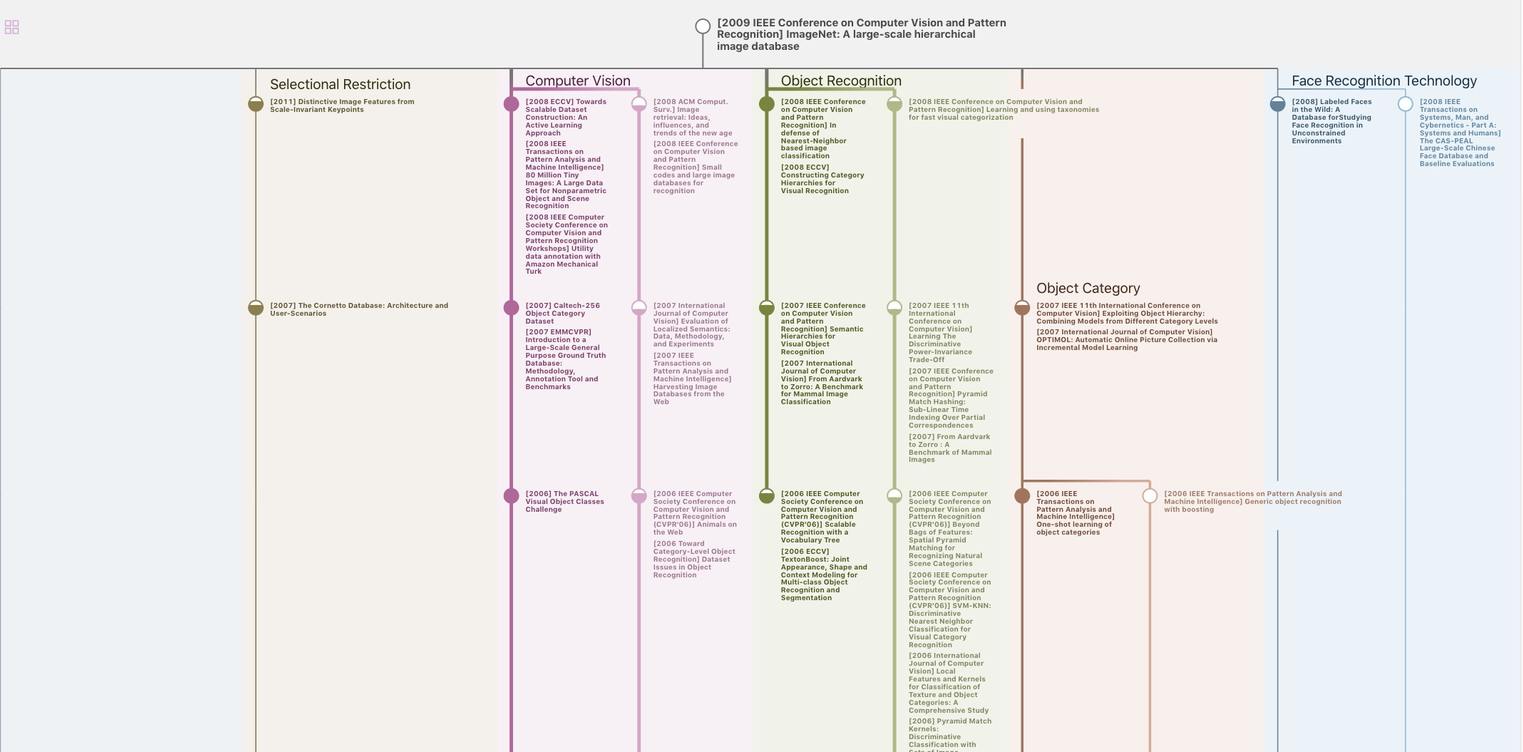
生成溯源树,研究论文发展脉络
Chat Paper
正在生成论文摘要