Modeling User's Neutral Feedback in Conversational Recommendation
NEURAL INFORMATION PROCESSING, ICONIP 2023, PT IV(2024)
摘要
Conversational recommendation systems (CRS) enable the traditional recommender systems to obtain dynamic user preferences with interactive conversations. Although CRS has shown success in generating recommendation lists based on user's preferences, existing methods restrict users to make binary responses, i.e., accept and reject, after recommending, which limits users from expressing their needs. In fact, the user's rejection feedback may contain other valuable information. To address this limitation, we try to refine user's negative item-level feedback into attribute-level and extend CRS to a more realistic scenario that not only incorporates positive and negative feedback, but also neutral feedback. Neutral feedback denotes incomplete satisfaction with recommended items, which can help CRS infer user's preferences. To better cope with the new setting, we propose a CRS model called Neutral Feedback in Conversational Recommendation (NFCR). We adopt a joint learning task framework for feature extraction and use inverse reinforcement learning to train the decision network, helping CRS make appropriate decisions at each turn. Finally, we utilize the fine-grained neutral feedback from users to acquire their dynamic preferences in the update and deduction module. We conducted comprehensive evaluations on four benchmark datasets to demonstrate the effectiveness of our model.
更多查看译文
关键词
Multi-round Conversational Recommendation,User's Neutral Feedback,Graph Representation Learning
AI 理解论文
溯源树
样例
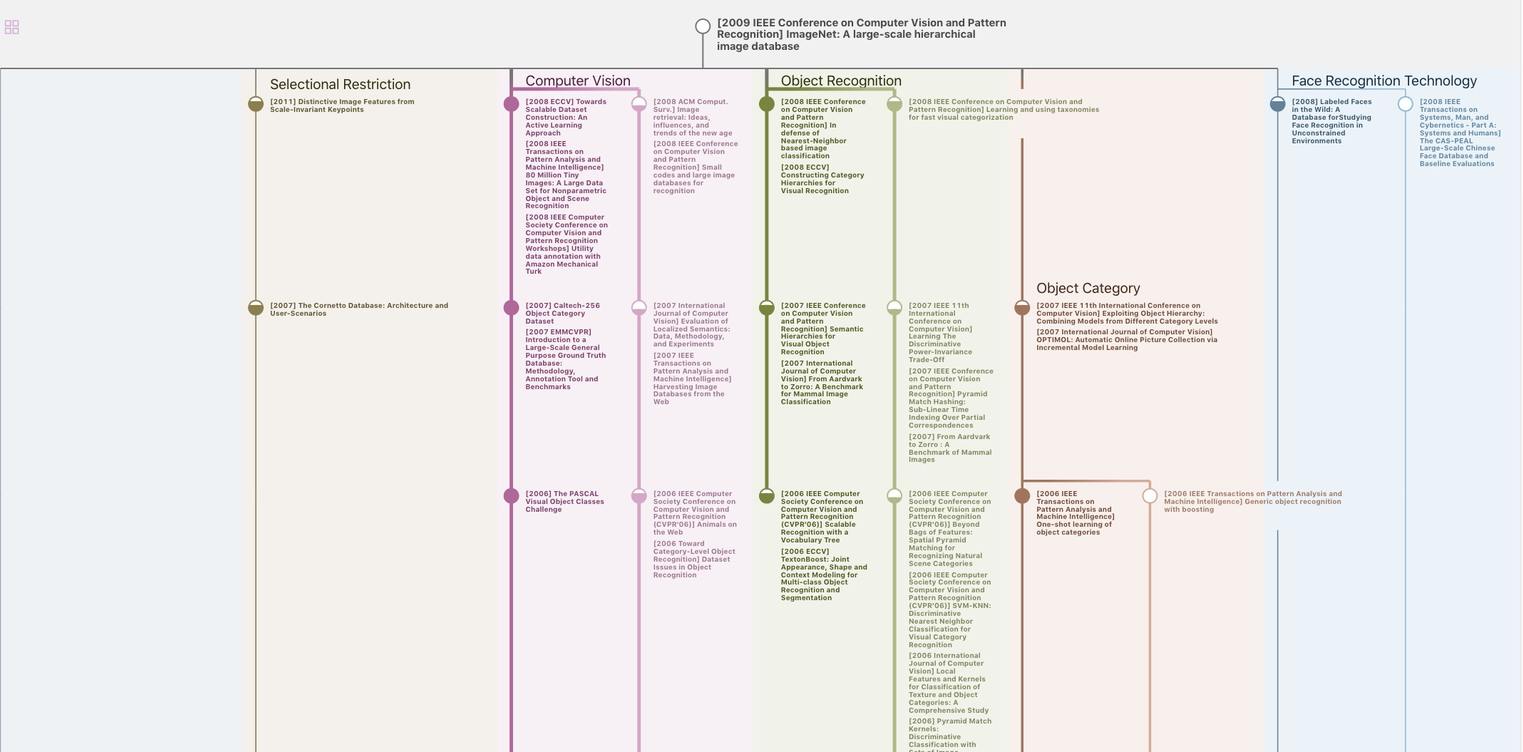
生成溯源树,研究论文发展脉络
Chat Paper
正在生成论文摘要